The DTW-based representation space for seismic pattern classification
Computers & Geosciences(2015)
Abstract
Distinguishing among the different seismic volcanic patterns is still one of the most important and labor-intensive tasks for volcano monitoring. This task could be lightened and made free from subjective bias by using automatic classification techniques. In this context, a core but often overlooked issue is the choice of an appropriate representation of the data to be classified. Recently, it has been suggested that using a relative representation (i.e. proximities, namely dissimilarities on pairs of objects) instead of an absolute one (i.e. features, namely measurements on single objects) is advantageous to exploit the relational information contained in the dissimilarities to derive highly discriminant vector spaces, where any classifier can be used. According to that motivation, this paper investigates the suitability of a dynamic time warping (DTW) dissimilarity-based vector representation for the classification of seismic patterns. Results show the usefulness of such a representation in the seismic pattern classification scenario, including analyses of potential benefits from recent advances in the dissimilarity-based paradigm such as the proper selection of representation sets and the combination of different dissimilarity representations that might be available for the same data.
MoreTranslated text
Key words
Classification,Dissimilarity space,Dynamic time warping,Seismic patterns,Volcano monitoring
AI Read Science
Must-Reading Tree
Example
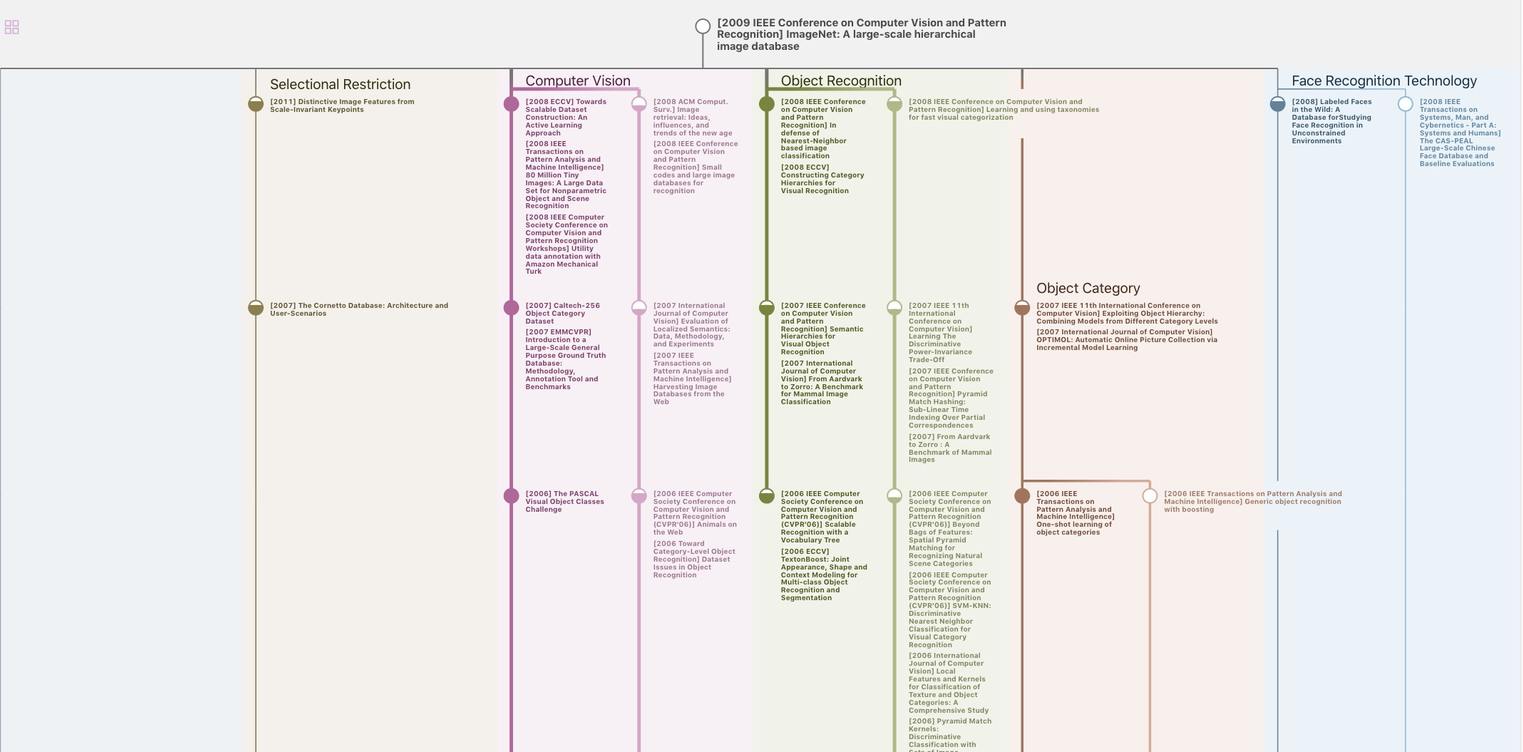
Generate MRT to find the research sequence of this paper
Chat Paper
Summary is being generated by the instructions you defined