Budget constrained non-monotonic feature selection
Neural Networks(2015)
摘要
Feature selection is an important problem in machine learning and data mining. We consider the problem of selecting features under the budget constraint on the feature subset size. Traditional feature selection methods suffer from the “monotonic” property. That is, if a feature is selected when the number of specified features is set, it will always be chosen when the number of specified feature is larger than the previous setting. This sacrifices the effectiveness of the non-monotonic feature selection methods. Hence, in this paper, we develop an algorithm for non-monotonic feature selection that approximates the related combinatorial optimization problem by a Multiple Kernel Learning (MKL) problem. We justify the performance guarantee for the derived solution when compared to the global optimal solution for the related combinatorial optimization problem. Finally, we conduct a series of empirical evaluation on both synthetic and real-world benchmark datasets for the classification and regression tasks to demonstrate the promising performance of the proposed framework compared with the baseline feature selection approaches.
更多查看译文
关键词
Feature selection,Multiple kernel learning,Budget constraint,Non-monotonic
AI 理解论文
溯源树
样例
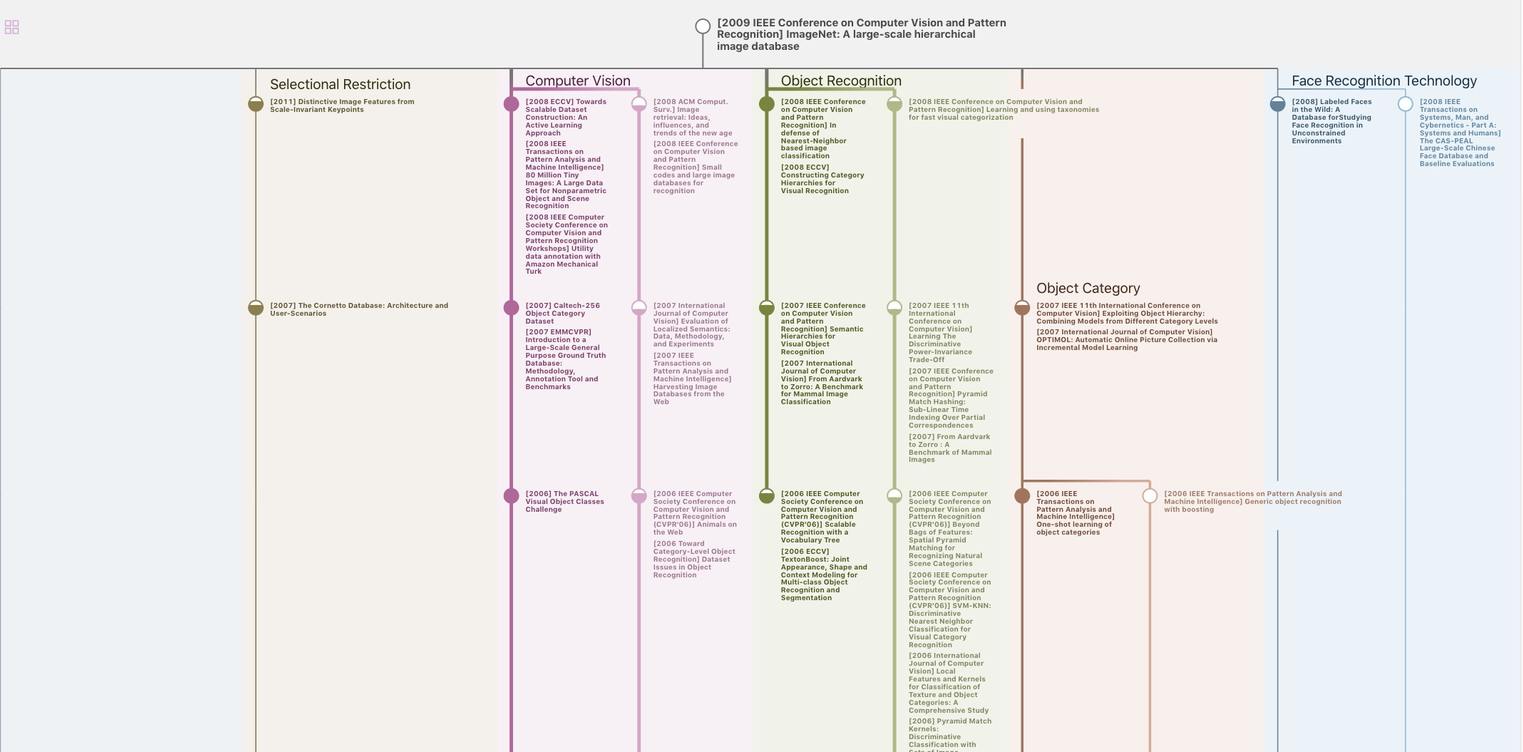
生成溯源树,研究论文发展脉络
Chat Paper
正在生成论文摘要