Fuzzy time series forecasting based on fuzzy logical relationships and similarity measures
Information Sciences(2016)
摘要
In this paper, we propose a new fuzzy time series forecasting method for forecasting the Taiwan Stock Exchange Capitalization Weighted Stock Index (TAIEX) based on fuzzy time series, fuzzy logical relationships, particle swarm optimization techniques, the K-means clustering algorithm, and similarity measures between the subscript of the fuzzy set of the fuzzified historical testing datum on the previous trading day and the subscripts of the fuzzy sets appearing in the current states of the fuzzy logical relationships in the chosen fuzzy logical relationship group. The particle swarm optimization techniques are used to get the optimal partition of the intervals in the universe of discourse. The K-means clustering algorithm is used to cluster the subscripts of the fuzzy sets of the current states of the fuzzy logical relationships to get the cluster center of each cluster and to divide the constructed fuzzy logical relationships into fuzzy logical relationship groups. The experimental results show that the proposed fuzzy forecasting method gets higher forecasting accuracy rates than the existing methods. The advantages of the proposed fuzzy forecasting method is that it uses the particle swarm optimization techniques to get the optimal partition of the intervals in the universe of discourse and uses the K-means clustering algorithm to cluster the subscripts of the fuzzy sets of the current states of the fuzzy logical relationships to get the cluster center of each cluster and to divide the constructed fuzzy logical relationships into fuzzy logical relationship groups for increasing the forecasting accuracy rates.
更多查看译文
关键词
Fuzzy logical relationships,Fuzzy time series,K-means clustering algorithm,Particle swarm optimization,Similarity measures
AI 理解论文
溯源树
样例
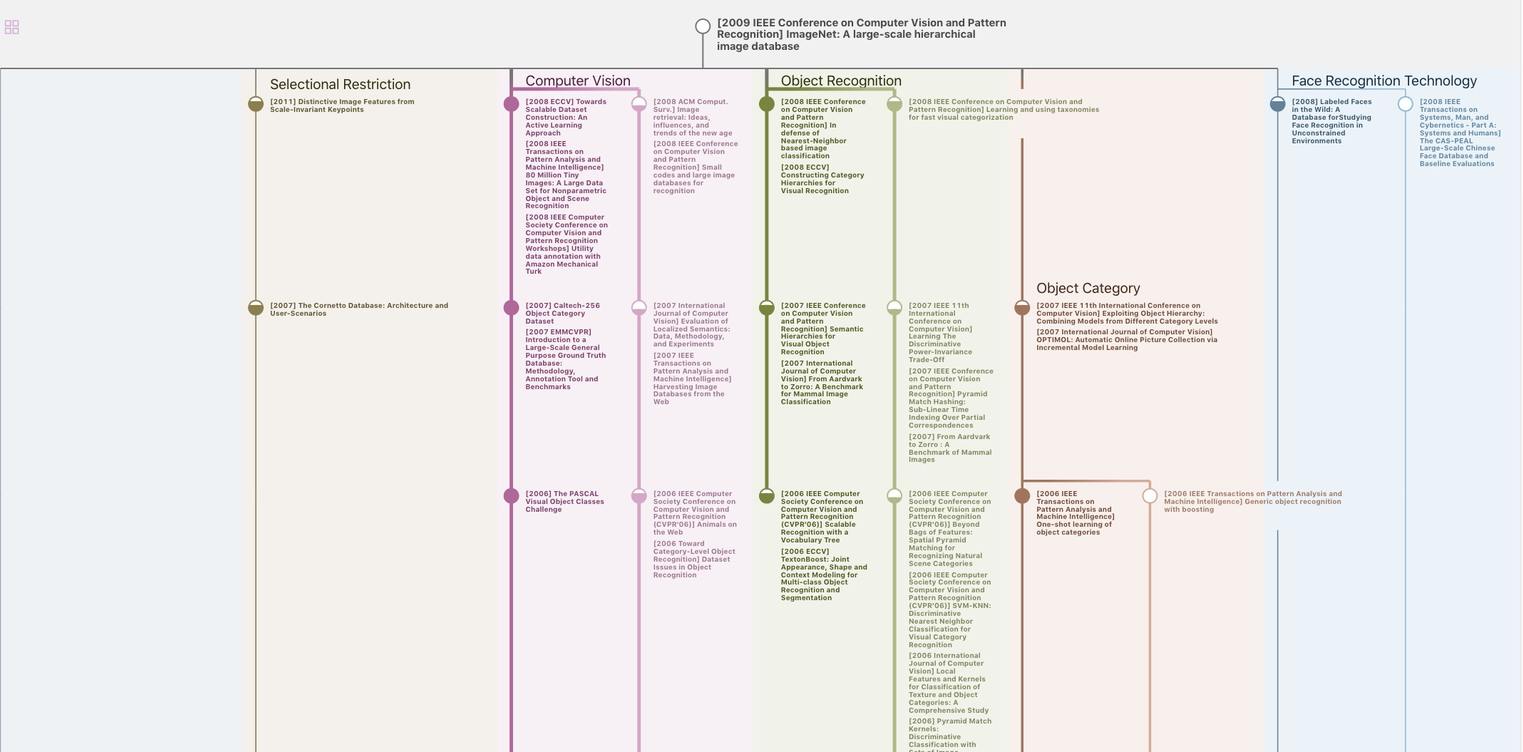
生成溯源树,研究论文发展脉络
Chat Paper
正在生成论文摘要