Image reconstruction from random samples using multiscale regression framework.
Neurocomputing(2016)
摘要
Preserving edge details is an important issue in most of the image reconstruction problems. In this paper, we propose a multiscale regression framework for image reconstruction from sparse random samples. A multiscale framework is used here to combine the modeling strengths of parametric and non-parametric statistical techniques in a pyramidal fashion. This algorithm is designed to preserve edge structures using an adaptive filter, where the filter coefficients are derived using locally adapted kernels which take into account both the local density of the available samples, and the actual values of these samples. As such, they are automatically directed and adapted to both the given sampling geometry and the samples׳ radiometry. Both the upscaling and missing pixel recovery processes are made locally adaptive so that the image structures can be well preserved. Experimental results demonstrate that the proposed method achieves better improvement over the state-of-the-art algorithms in terms of both subjective and objective quality.
更多查看译文
关键词
Image modeling,Image reconstruction,Kernel regression,Inverse problem
AI 理解论文
溯源树
样例
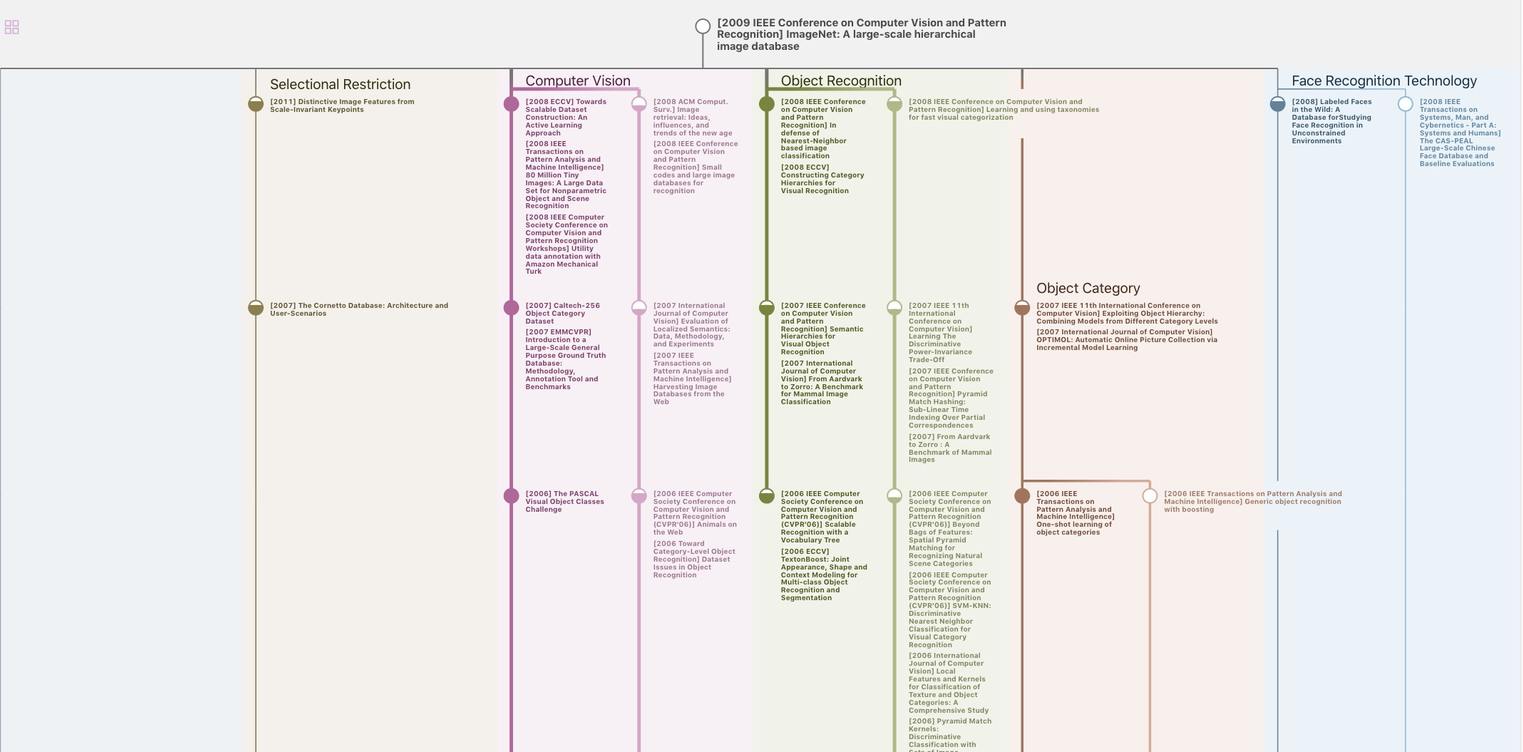
生成溯源树,研究论文发展脉络
Chat Paper
正在生成论文摘要