A multiwavelet-based time-varying model identification approach for time-frequency analysis of EEG signals.
Neurocomputing(2016)
Abstract
An efficient multiwavelet-based time-varying modeling scheme is proposed for time-frequency analysis (TFA) of electroencephalogram (EEG) data. In the new multiwavelet-based parametric modeling framework, the time-dependent parameters in the time-varying model are locally represented using a novel multiwavelet decomposition scheme. An effective orthogonal least squares (OLS) algorithm aided by mutual information criterion is then applied for sparse model selection and parameter estimation. The resultant estimation of time-dependent spectral density in the signal can simultaneously achieve a high resolution in both time and frequency, which is a powerful TFA technique for nonstationary biomedical signals including EEG. Two examples, one for an artificial EEG signal and another for a real EEG signal are included to show the effectiveness and applicability of the new proposed approach. Simulation studies and applications to real EEG data elucidate that the proposed wavelet approach is capable of achieving a high time-frequency representation for nonstationary processes.
MoreTranslated text
Key words
Chebyshev polynomials,EEG data,Kalman filter,Mutual information,Wavelet,Orthogonal least squares (OLS),Time-varying system identification,Time–frequency analysis
AI Read Science
Must-Reading Tree
Example
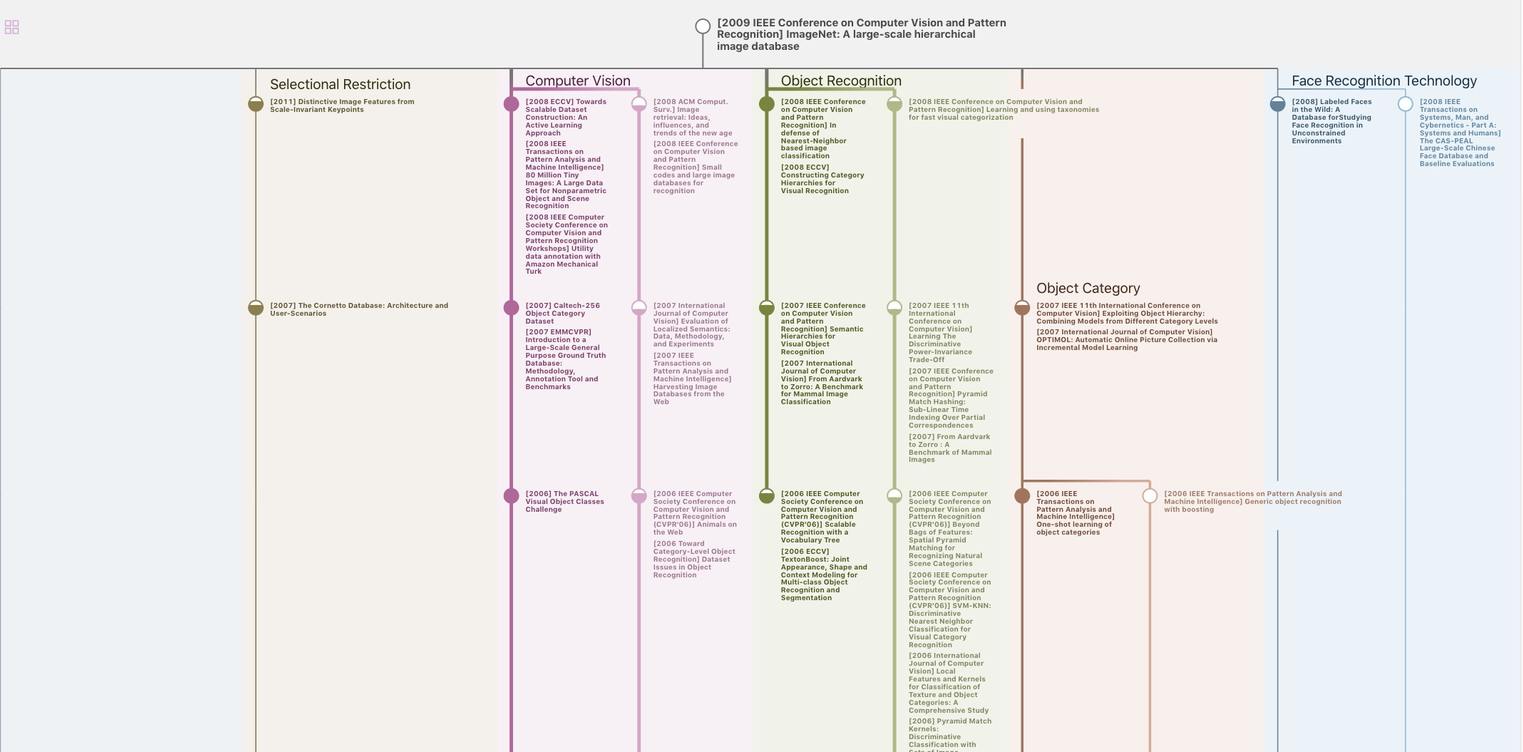
Generate MRT to find the research sequence of this paper
Chat Paper
Summary is being generated by the instructions you defined