PCA filtering and probabilistic SOM for network intrusion detection
Neurocomputing(2015)
摘要
The growth of the Internet and, consequently, the number of interconnected computers, has exposed significant amounts of information to intruders and attackers. Firewalls aim to detect violations according to a predefined rule-set and usually block potentially dangerous incoming traffic. However, with the evolution of attack techniques, it is more difficult to distinguish anomalies from normal traffic. Different detection approaches have been proposed, including the use of machine learning techniques based on neural models such as Self-Organizing Maps (SOMs). In this paper, we present a classification approach that hybridizes statistical techniques and SOM for network anomaly detection. Thus, while Principal Component Analysis (PCA) and Fisher Discriminant Ratio (FDR) have been considered for feature selection and noise removal, Probabilistic Self-Organizing Maps (PSOM) aim to model the feature space and enable distinguishing between normal and anomalous connections. The detection capabilities of the proposed system can be modified without retraining the map, but only by modifying the units activation probabilities. This deals with fast implementations of Intrusion Detection Systems (IDS) necessary to cope with current link bandwidths.
更多查看译文
关键词
Probabilistic SOM,Bayesian SOM,IDS,Self-organizing maps,PCA filtering
AI 理解论文
溯源树
样例
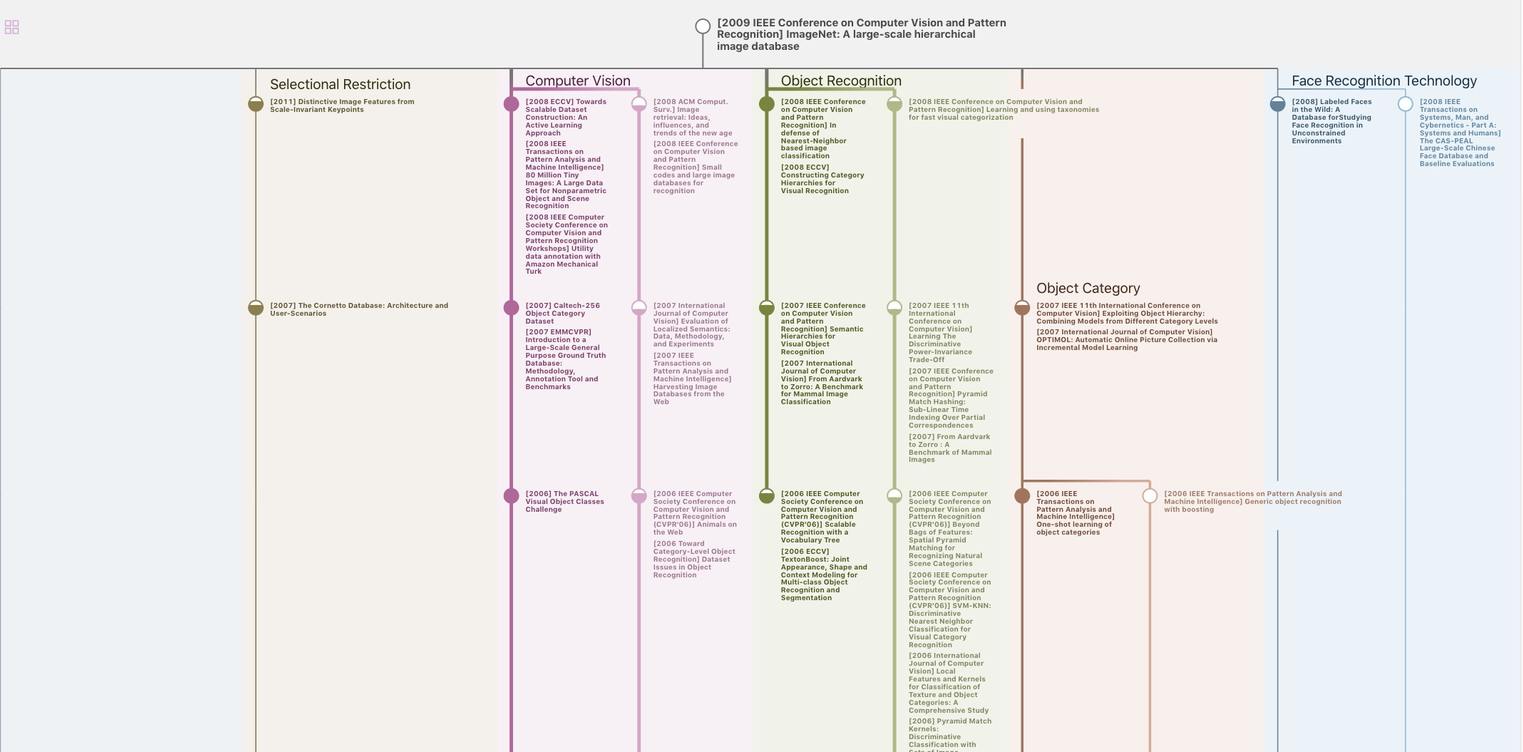
生成溯源树,研究论文发展脉络
Chat Paper
正在生成论文摘要