Efficient Saliency-Based Object Detection in Remote Sensing Images Using Deep Belief Networks
IEEE Geoscience and Remote Sensing Letters(2016)
摘要
Object detection has been one of the hottest issues in the field of remote sensing image analysis. In this letter, an efficient object detection framework is proposed, which combines the strength of the unsupervised feature learning of deep belief networks (DBNs) and visual saliency. In particular, we propose an efficient coarse object locating method based on a saliency mechanism. The method could avoid an exhaustive search across the image and generate a small number of bounding boxes, which can locate the object quickly and precisely. After that, the trained DBN is used for feature extraction and classification on subimages. The feature learning of the DBN is operated by pretraining each layer of restricted Boltzmann machines (RBMs) using the general layerwise training algorithm. An unsupervised blockwise pretraining strategy is introduced to train the first layer of RBMs, which combines the raw pixels with a saliency map as inputs. This makes an RBM generate local and edge filters. The precise edge position information and pixel value information are more efficient to build a good model of images. Comparative experiments are conducted on the data set acquired by QuickBird with a 60-cm resolution. The results demonstrate the accuracy and efficiency of our method.
更多查看译文
关键词
Deep belief networks (DBNs),object detection,visual saliency
AI 理解论文
溯源树
样例
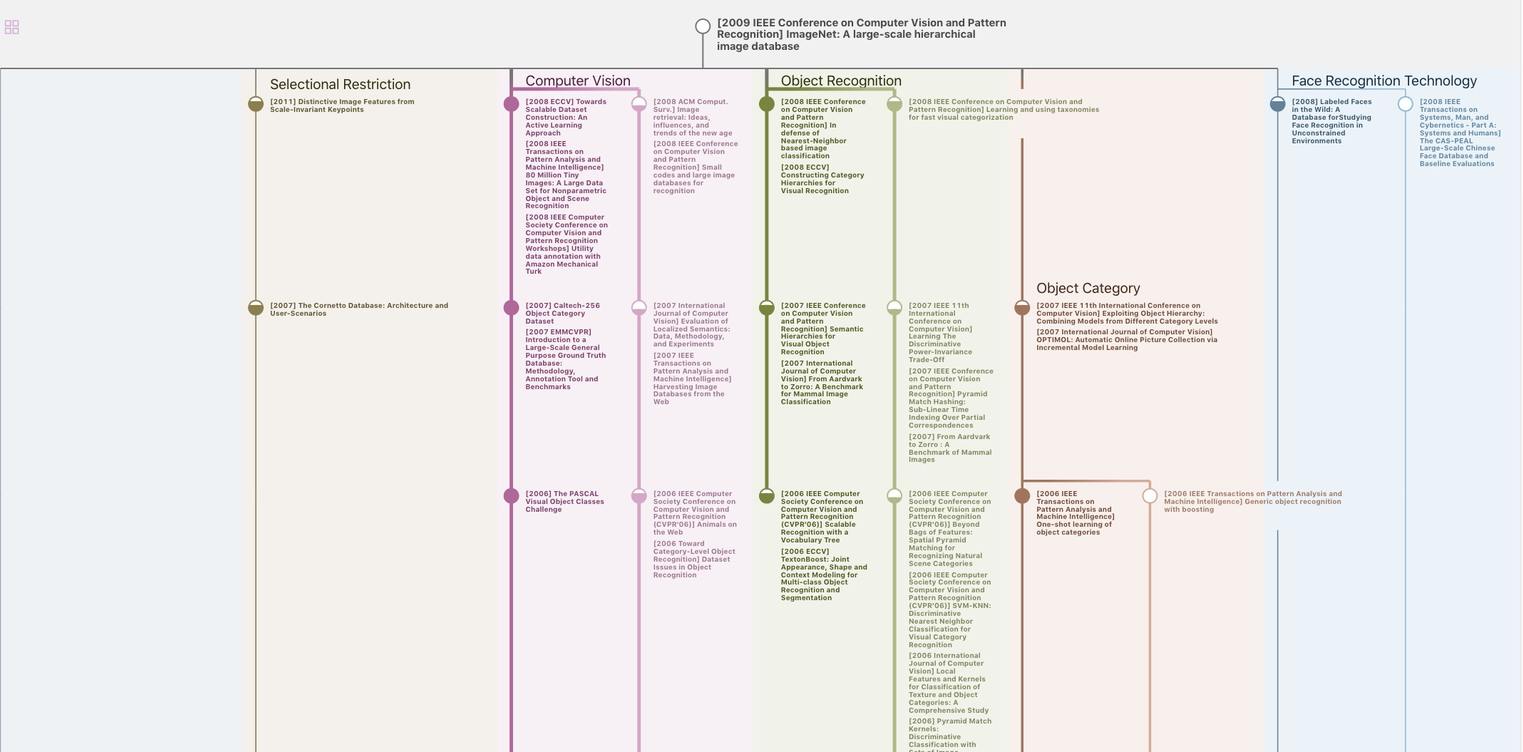
生成溯源树,研究论文发展脉络
Chat Paper
正在生成论文摘要