An integrated PSO for parameter determination and feature selection of ELM and its application in classification of power system disturbances
Applied Soft Computing(2015)
摘要
new learning method for power system disturbances is introduced using extreme learning machine.Simultaneously optimize the feature subset and model selection for the ELM using PSO.Proposed method can improve convergence accuracy and generalization performance of ELM. This paper presents a performance enhancement scheme for the recently developed extreme learning machine (ELM) for classifying power system disturbances using particle swarm optimization (PSO). Learning time is an important factor while designing any computational intelligent algorithms for classifications. ELM is a single hidden layer neural network with good generalization capabilities and extremely fast learning capacity. In ELM, the input weights are chosen randomly and the output weights are calculated analytically. However, ELM may need higher number of hidden neurons due to the random determination of the input weights and hidden biases. One of the advantages of ELM over other methods is that the parameter that the user must properly adjust is the number of hidden nodes only. But the optimal selection of its parameter can improve its performance. In this paper, a hybrid optimization mechanism is proposed which combines the discrete-valued PSO with the continuous-valued PSO to optimize the input feature subset selection and the number of hidden nodes to enhance the performance of ELM. The experimental results showed the proposed algorithm is faster and more accurate in discriminating power system disturbances.
更多查看译文
关键词
Wavelet transforms,Feature selection,Particle swarm optimization,Extreme learning machine
AI 理解论文
溯源树
样例
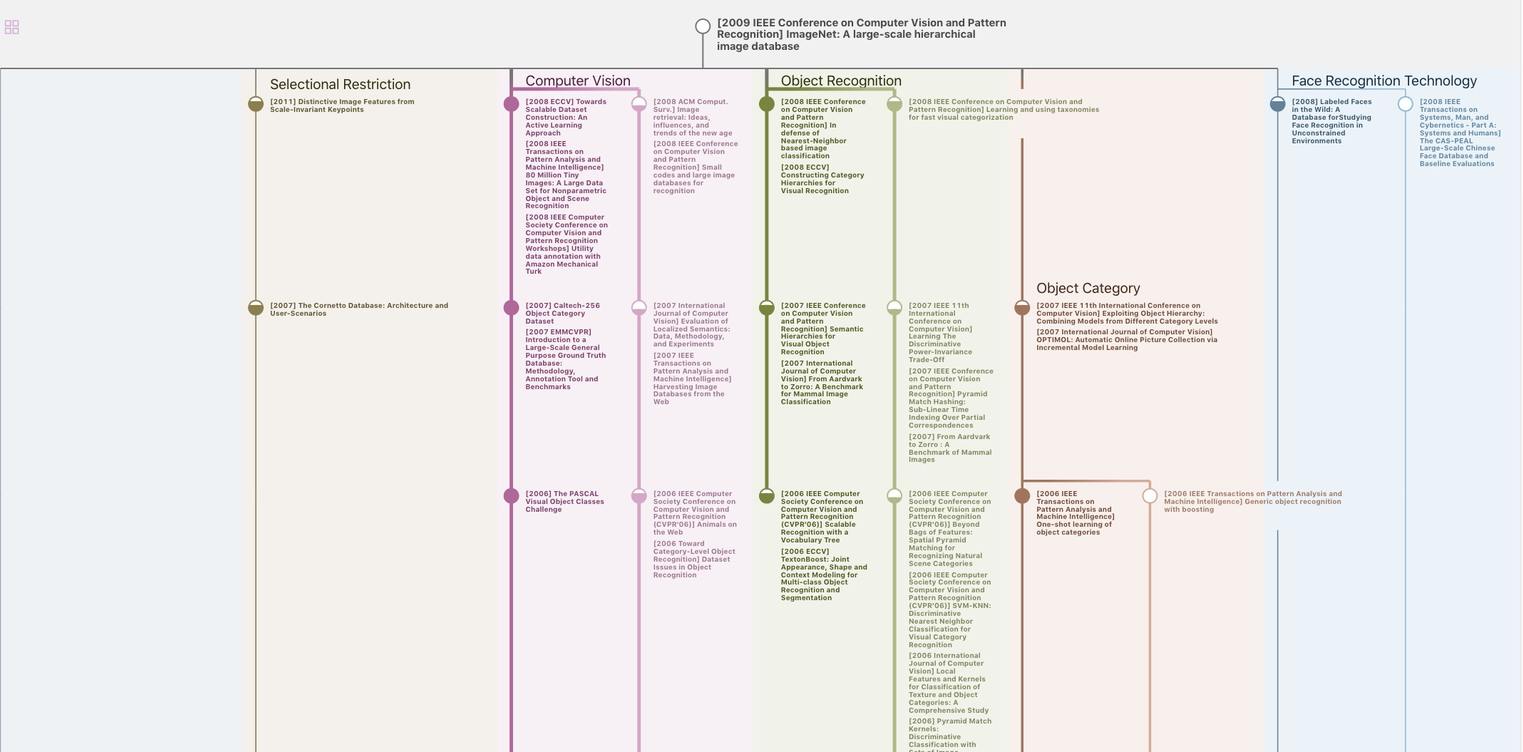
生成溯源树,研究论文发展脉络
Chat Paper
正在生成论文摘要