S4-Tuning: A Simple Cross-lingual Sub-network Tuning Method
ACL 2022(2022)
Abstract
The emergence of multilingual pre-trained language models makes it possible to adapt to target languages with only few labeled examples.However, vanilla fine-tuning tends to achieve degenerated and unstable results, owing to the Language Interference among different languages, and Parameter Overload under the few-sample transfer learning scenarios.To address two problems elegantly, we propose S 4 -Tuning, a Simple Cross-lingual Sub-network Tuning method. S 4 -Tuning first detects the most essential sub-network for each target language, and only updates it during fine-tuning.In this way, the language sub-networks lower the scale of trainable parameters, and hence better suit the low-resource scenarios.Meanwhile, the commonality and characteristics across languages are modeled by the overlapping and non-overlapping parts to ease the interference among languages.Simple but effective, S 4 -Tuning gains consistent improvements over vanilla fine-tuning on three multi-lingual tasks involving 37 different languages in total (XNLI, PAWS-X, and Tatoeba).
MoreTranslated text
AI Read Science
Must-Reading Tree
Example
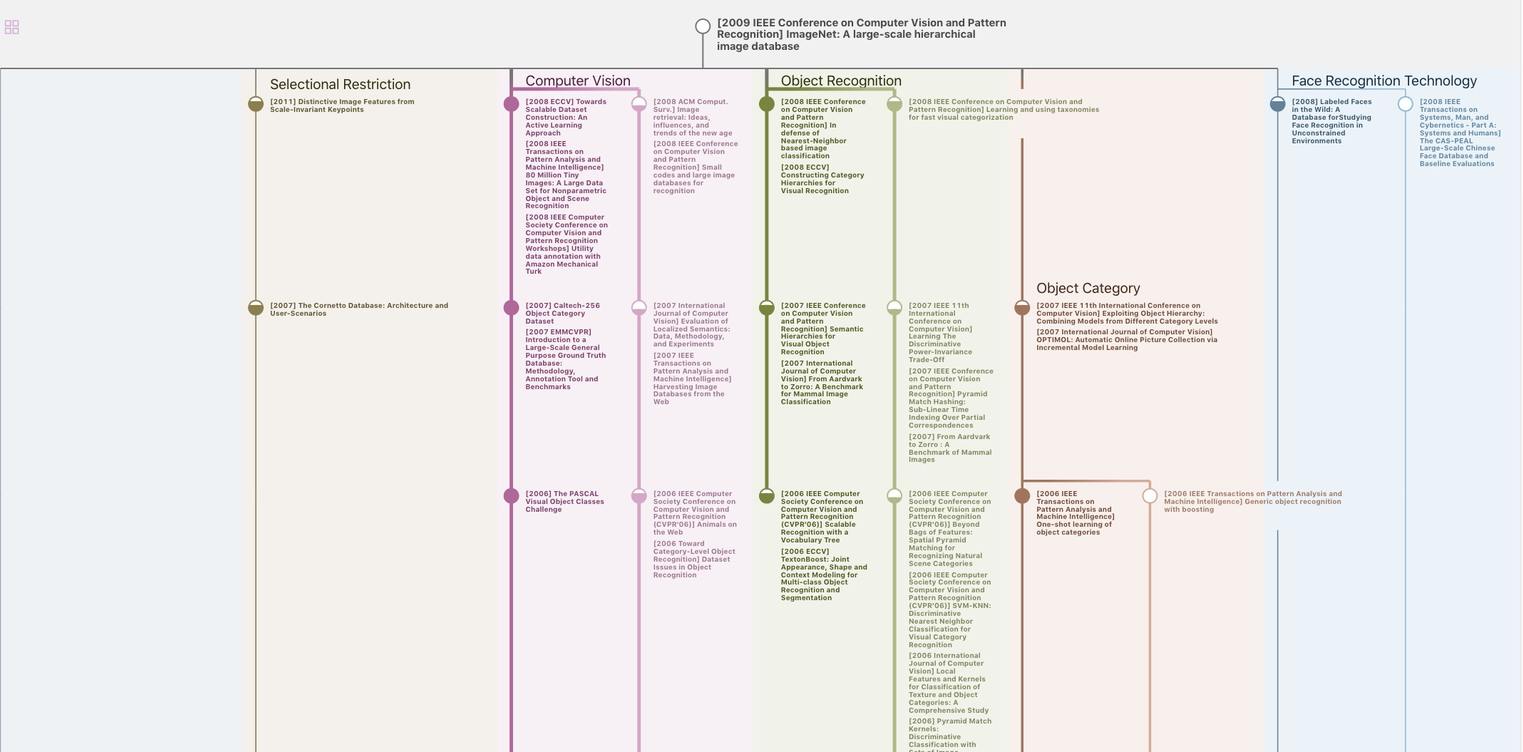
Generate MRT to find the research sequence of this paper
Chat Paper
Summary is being generated by the instructions you defined