Super-thresholding: Supervised Thresholding of Protein Crystal Images
IEEE/ACM Trans. Comput. Biology Bioinform.(2017)
摘要
In general, a single thresholding technique is developed or enhanced to separate foreground objects from background for a domain of images. This idea may not generate satisfactory results for all images in a dataset, since different images may require different types of thresholding methods for proper binarization or segmentation. To overcome this limitation, in this study, we propose a novel approach called "super-thresholding" that utilizes a supervised classifier to decide an appropriate thresholding method for a specific image. This method provides a generic framework that allows selection of the best thresholding method among different thresholding techniques that are beneficial for the problem domain. A classifier model is built using features extracted priori from the original image only or posteriori by analyzing the outputs of thresholding methods and the original image. This model is applied to identify the thresholding method for new images of the domain. We performed our method on protein crystallization images, and then we compared our results with 6 thresholding techniques. Numerical results are provided using 4 different correctness measurements. Superthresholding outperforms the best single thresholding method around 10%, and it gives the best performance for protein crystallization dataset in our experiments.
更多查看译文
关键词
Image Binarization,Protein Crystallization,Supervised Thresholding
AI 理解论文
溯源树
样例
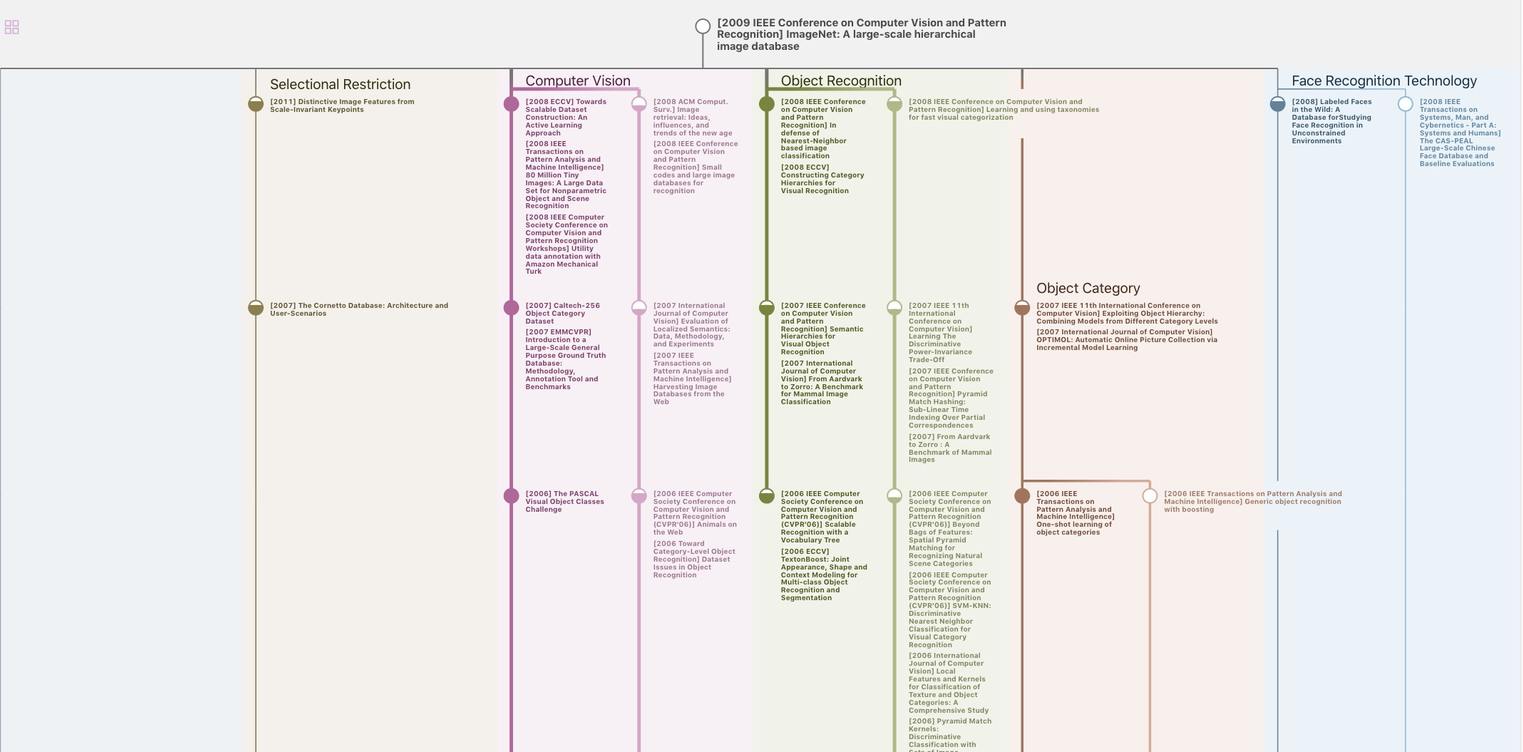
生成溯源树,研究论文发展脉络
Chat Paper
正在生成论文摘要