Ellipse Fitting Using the Finite Rate of Innovation Sampling Principle
IEEE Transactions on Image Processing(2016)
摘要
Standard approaches for ellipse fitting are based on the minimization of algebraic or geometric distance between the given data and a template ellipse. When the data are noisy and come from a partial ellipse, the state-of-the-art methods tend to produce biased ellipses. We rely on the sampling structure of the underlying signal and show that the $x$ - and $y$ -coordinate functions of an ellipse are finite-rate-of-innovation (FRI) signals, and that their parameters are estimable from partial data. We consider both uniform and nonuniform sampling scenarios in the presence of noise and show that the data can be modeled as a sum of random amplitude-modulated complex exponentials. A low-pass filter is used to suppress noise and approximate the data as a sum of weighted complex exponentials. The annihilating filter used in FRI approaches is applied to estimate the sampling interval in the closed form. We perform experiments on simulated and real data, and assess both objective and subjective performances in comparison with the state-of-the-art ellipse fitting methods. The proposed method produces ellipses with lesser bias. Furthermore, the mean-squared error is lesser by about 2 to 10 dB. We show the applications of ellipse fitting in iris images starting from partial edge contours, and to free-hand ellipses drawn on a touch-screen tablet.
更多查看译文
关键词
curve fitting,iris recognition,low-pass filters,mean square error methods,FRI approaches,ellipse fitting method,finite-rate-of-innovation signals,geometric distance,innovation sampling principle,iris images,low-pass filter,mean-squared error,weighted complex exponentials,x-coordinate functions,y-coordinate functions,Ellipse fitting,annihilating filter,conic section,finite rate of innovation (FRI),nonuniform sampling,random amplitude modulation
AI 理解论文
溯源树
样例
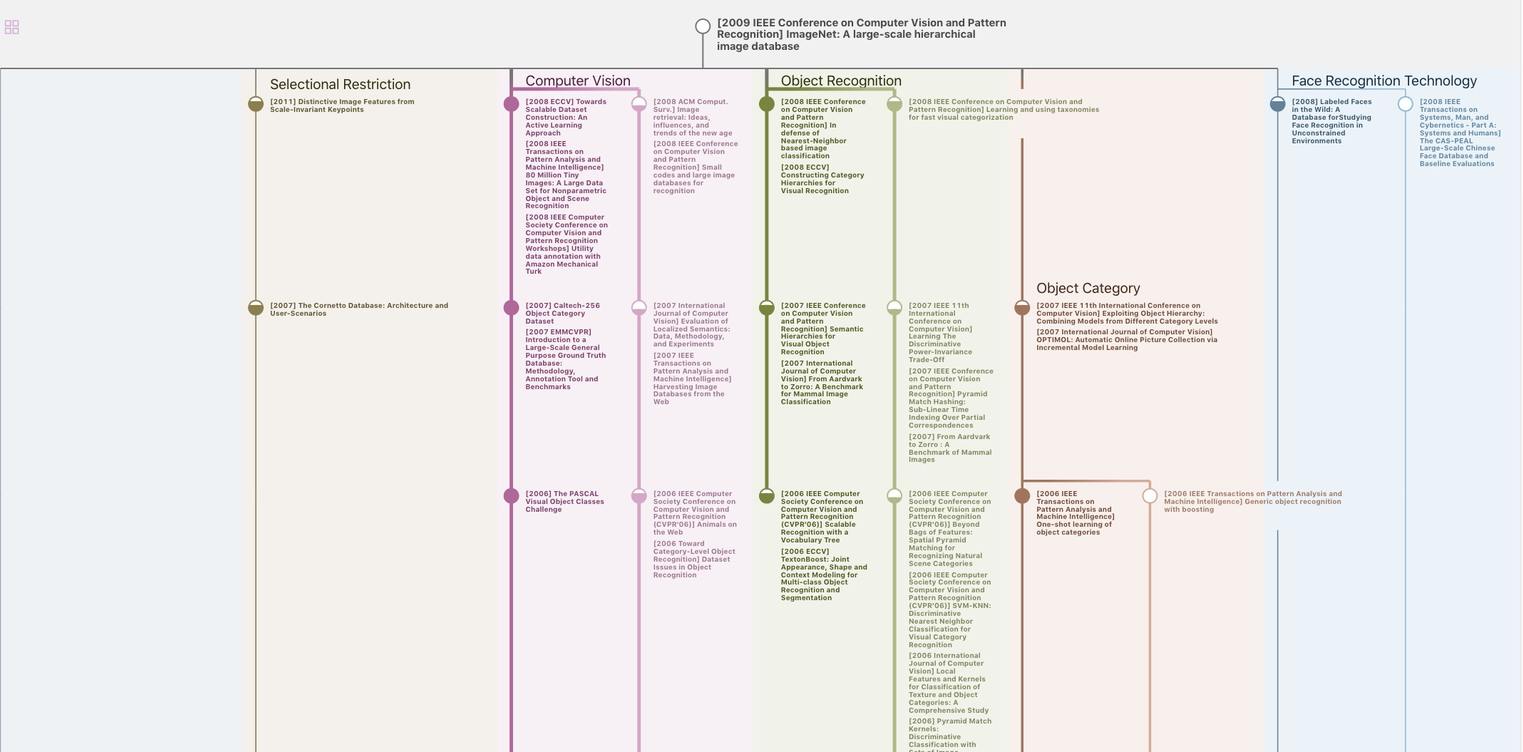
生成溯源树,研究论文发展脉络
Chat Paper
正在生成论文摘要