Beyond The Shortest Path : Unsupervised Domain Adaptation By Sampling Subspaces Along The Spline Flow
2015 IEEE CONFERENCE ON COMPUTER VISION AND PATTERN RECOGNITION (CVPR)(2015)
摘要
Recently, a particular paradigm I in the domain adaptation field has received considerable attention by introducing novel and important insights to the problem. In this case, the source and target domains are represented in the form of subspaces, which are treated as points on the Grassmann manifold. The geodesic curve between them is sampled to obtain intermediate points. Then a classifier is learnt using the projections of the data onto these subspaces. Despite its relevance and popularity, this paradigm [18] contains some limitations. Firstly, in real-world applications, that simple curve (i.e. shortest path) does not provide the necessary flexibility to model the domain shift between the training and testing data sets. Secondly, by using the geodesic curve, we are restricted to only one source domain, which does not allow to take fully advantage of the multiple datasets that are available nowadays. It is then, natural to ask whether this popular concept could be extended to deal with more complex curves and to integrate multi-sources domains. This is a hard problem considering the Riemannian structure of the space, but we propose a mathematically well-founded idea that enables us to solve it. We exploit the geometric insight of rolling maps [30] to compute a spline curve on the Grassmann manifold. The benefits of the proposed idea are demonstrated through several empirical studies on standard datasets. This novel concept allows to explicitly integrate multi-source domains while the previous one [18] uses the mean of all sources. This enables to model better the domain shift and take fully advantage of the training datasets.
更多查看译文
关键词
unsupervised domain adaptation,subspace sampling,spline flow,domain adaptation field,source domain representation,target domain representation,Grassmann manifold,geodesic curve,intermediate points,classifier learning,data projections,real-world applications,domain shift,training data sets,testing data sets,source domain,complex curves,multisource domain integration,Riemannian structure,rolling maps,spline curve,Grassmann manifold,empirical analysis,standard datasets,explicitly integrated multisource domains
AI 理解论文
溯源树
样例
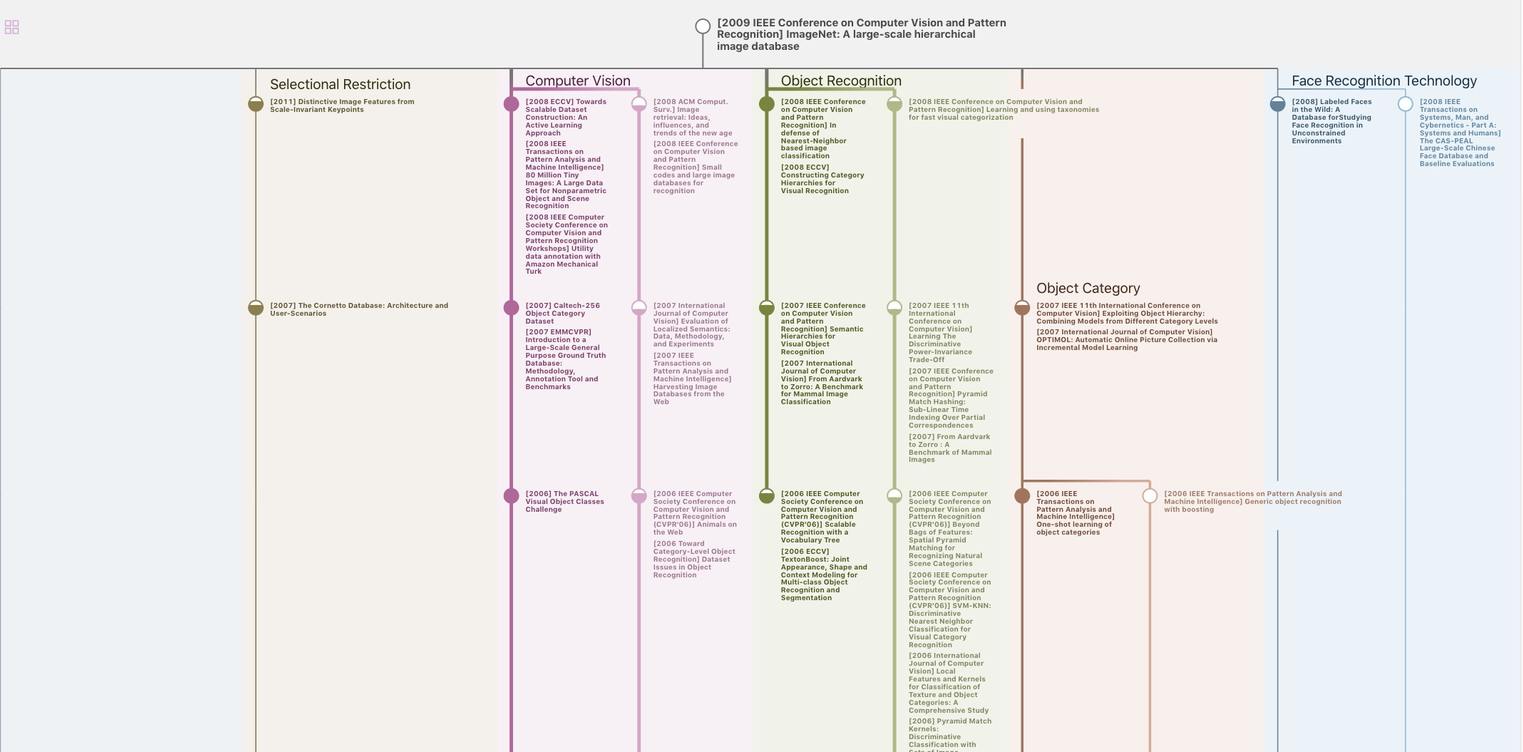
生成溯源树,研究论文发展脉络
Chat Paper
正在生成论文摘要