Low-Overhead Reinforcement Learning-Based Power Management Using 2QoSM
JOURNAL OF LOW POWER ELECTRONICS AND APPLICATIONS(2022)
Abstract
With the computational systems of even embedded devices becoming ever more powerful, there is a need for more effective and pro-active methods of dynamic power management. The work presented in this paper demonstrates the effectiveness of a reinforcement-learning based dynamic power manager placed in a software framework. This combination of Q-learning for determining policy and the software abstractions provide many of the benefits of co-design, namely, good performance, responsiveness and application guidance, with the flexibility of easily changing policies or platforms. The Q-learning based Quality of Service Manager (2QoSM) is implemented on an autonomous robot built on a complex, powerful embedded single-board computer (SBC) and a high-resolution path-planning algorithm. We find that the 2QoSM reduces power consumption up to 42% compared to the Linux on-demand governor and 10.2% over a state-of-the-art situation aware governor. Moreover, the performance as measured by path error is improved by up to 6.1%, all while saving power.
MoreTranslated text
Key words
middleware, power management, dynamic power management, reinforcement learning, dvfs, voltage scaling, machine learning
AI Read Science
Must-Reading Tree
Example
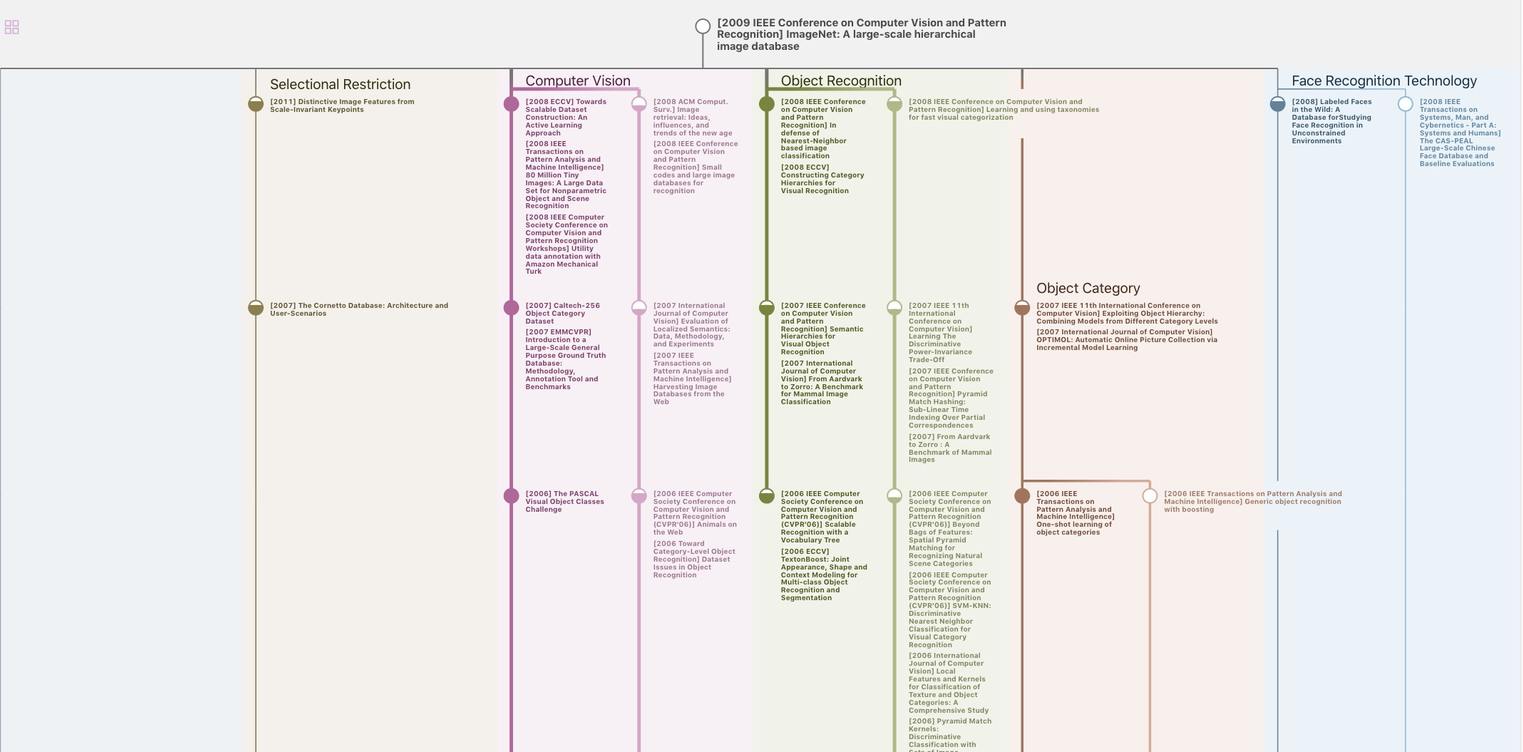
Generate MRT to find the research sequence of this paper
Chat Paper
Summary is being generated by the instructions you defined