Parallel outlier detection on uncertain data for GPUs
Distributed and Parallel Databases(2014)
摘要
Outlier detection, also known as anomaly detection, is a common data mining task in identifying data points that are outside expected patterns in a given dataset. It has useful applications such as network intrusion, system faults, and fraudulent activity. In addition, real world data are uncertain in nature and they may be represented as uncertain data. In this paper, we propose an improved parallel algorithm for outlier detection on uncertain data using density sampling and develop an implementation running on both GPUs and multi-core CPUs, using the OpenCL framework. Our main focus is on GPUs, as they are a cost effective massively parallel floating point processor that is suitable for many data mining applications. Our implementation exploits some key features in GPUs, and is significantly different from a traditional CPU implementation. We first present an improved uncertain outlier detection algorithm. Then, we demonstrate two parallel micro-clustering implementations. The performance and detection quality comparisons demonstrate the benefits of the improved algorithm and parallel implementation on GPUs.
更多查看译文
关键词
GPU,Outlier detection,Parallel processing,Uncertain data
AI 理解论文
溯源树
样例
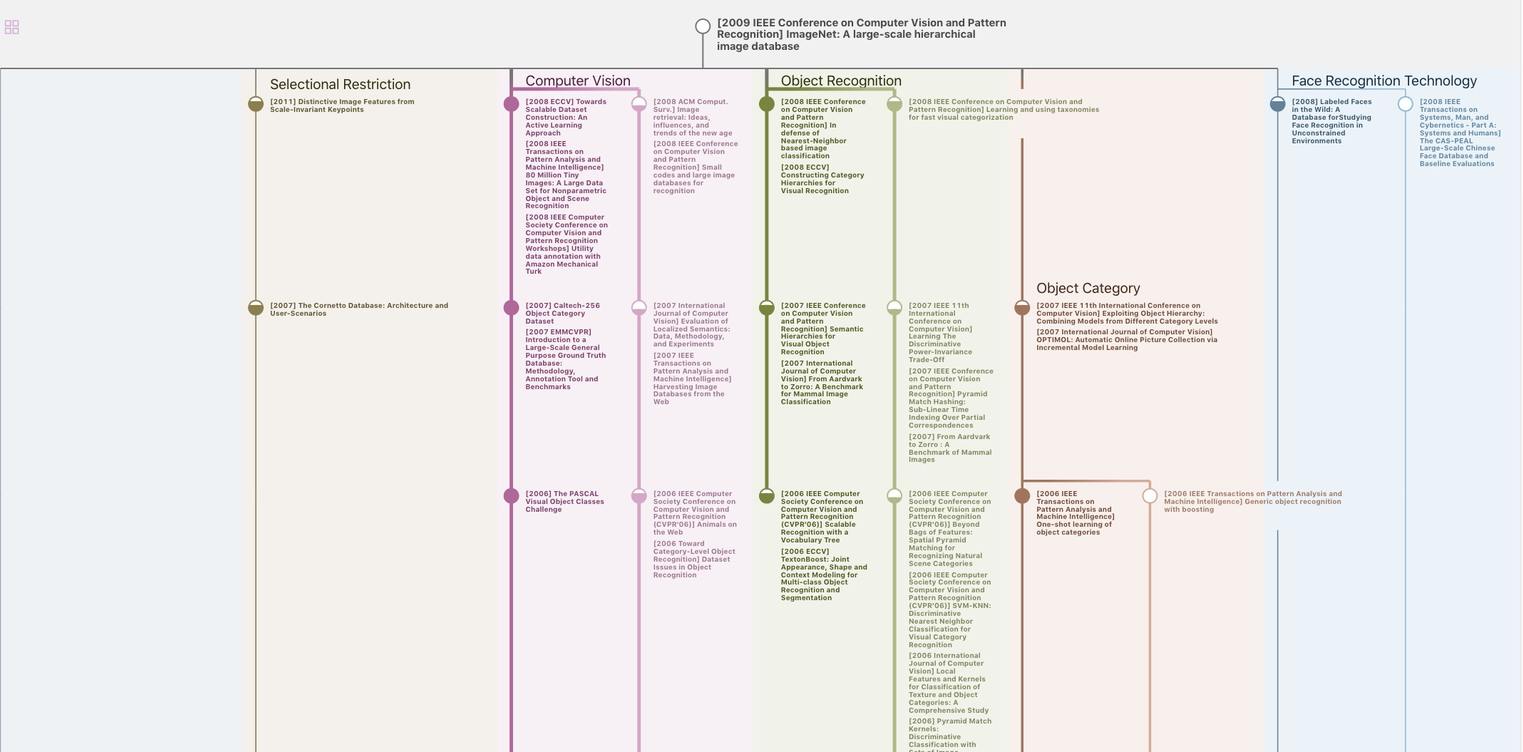
生成溯源树,研究论文发展脉络
Chat Paper
正在生成论文摘要