The Limits of Estimation of Distribution Algorithms
Advanced Materials Research(2014)
摘要
In this paper, we study the ability limit of EDAs to effectively solve problems in relation to the number of interactions among the variables. More in particular, we numerically analyze the learning limits that different EDA implementations encounter to solve problems on a sequence of additively decomposable functions (ADFs) in which new sub-functions are progressively added. The study is carried out in a worst-case scenario where the sub-functions are defined as deceptive functions. We argue that the limits for this type of algorithm are mainly imposed by the probabilistic model they rely on. Beyond the limitations of the approximate learning methods, the results suggest that, in general, the use of bayesian networks can entail strong computational restrictions to overcome the limits of applicability.
更多查看译文
关键词
probabilistic model,interaction,edas
AI 理解论文
溯源树
样例
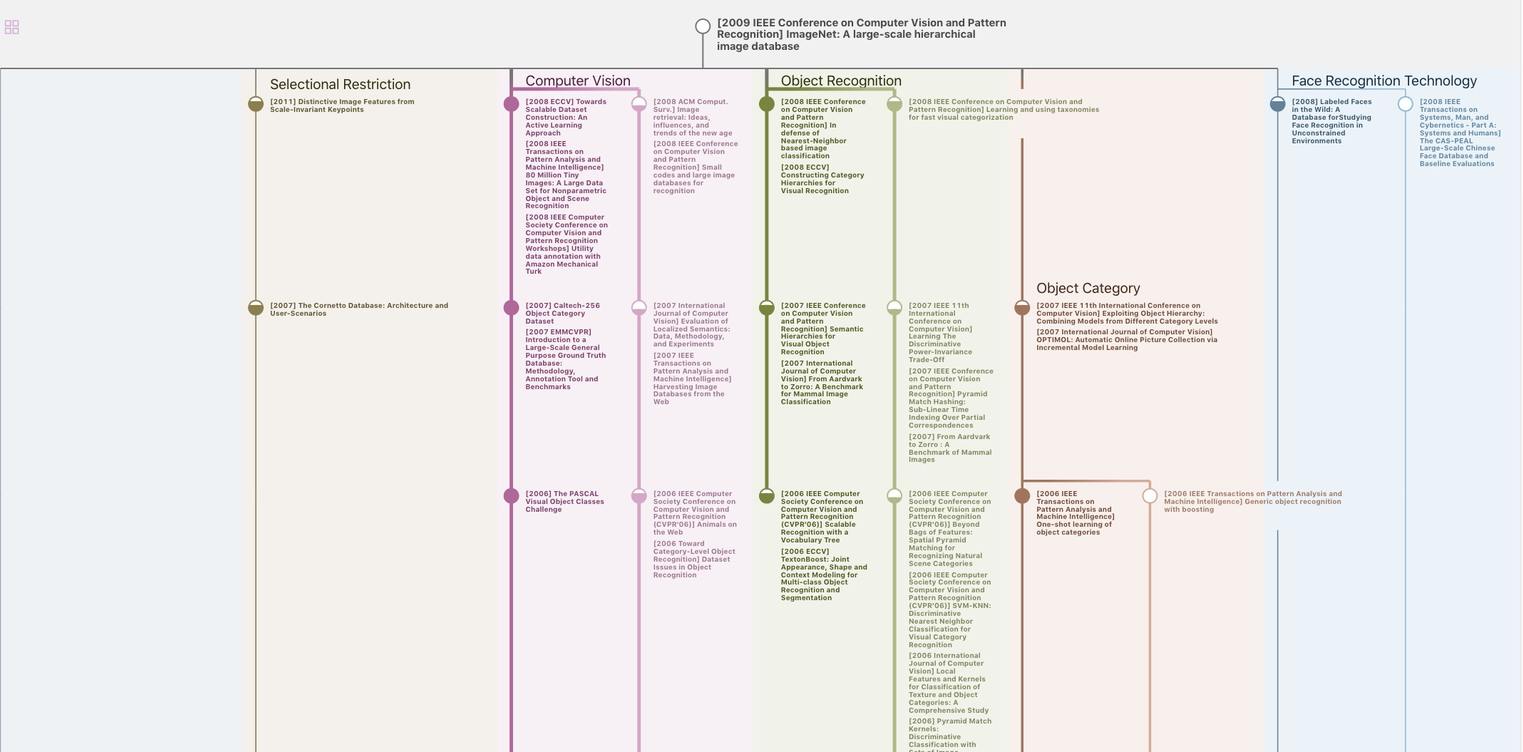
生成溯源树,研究论文发展脉络
Chat Paper
正在生成论文摘要