Fast mathematical modeling of partial-breach dam-break flow using a time-series field-reconstruction deep learning approach
PHYSICS OF FLUIDS(2023)
摘要
Mathematical modeling of dam-breach flow can provide a better understanding of dam failure events, which in turn helps people to reduce potential losses. In the present study, the smooth particle hydrodynamics (SPH) modeling approach was employed to simulate the three-dimensional (3D) partial-breach dam-break flow using two different viscosity models: the artificial viscosity and sub-particle-scale models. The validated and best-performing SPH model was further employed to conduct numerical experiments for various scenarios, which generated a comprehensive dataset. The current work also presents a novel time-series field-reconstruction deep learning (DL) approach: Time Series Convolutional Neural Input Network (TSCNIN) for modeling the transient process of partial-breach dam-break flow and for providing the complete flow field. This approach was constructed based on the long short-term memory and convolutional neural network algorithms with additional input layers. A DL-based model was trained and validated using the numerical data, and tested using two additional unseen scenarios. The results demonstrated that the DL-based model can accurately and efficiently predict the transient water inundation process, and model the influence of dam-break gaps. This study provided a new avenue of simulating partial-breach dam-break flow using the time-series DL approaches and demonstrated the capability of the TSCNIN algorithm in reconstructing the complete fields of transient variables.
更多查看译文
关键词
deep learning,deep learning approach,flow,fast mathematical modeling,partial-breach,dam-break,time-series,field-reconstruction
AI 理解论文
溯源树
样例
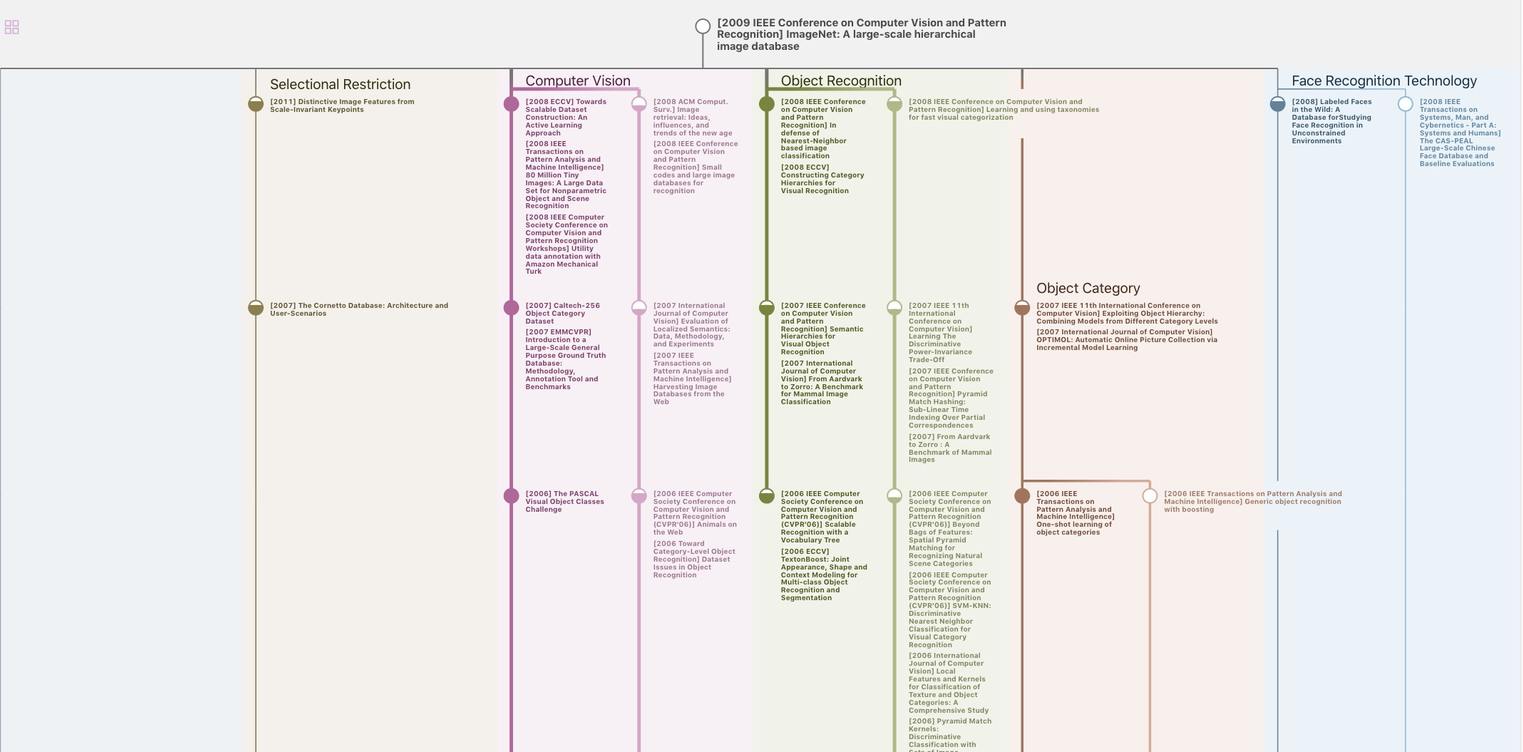
生成溯源树,研究论文发展脉络
Chat Paper
正在生成论文摘要