Seasonal prediction of winter extreme precipitation over Canada by support vector regression
HYDROLOGY AND EARTH SYSTEM SCIENCES(2011)
摘要
For forecasting the maximum 5-day accumulated precipitation over the winter season at lead times of 3, 6, 9 and 12 months over Canada from 1950 to 2007, two nonlinear and two linear regression models were used, where the models were support vector regression (SVR) (nonlinear and linear versions), nonlinear Bayesian neural network (BNN) and multiple linear regression (MLR). The 118 stations were grouped into six geographic regions by K-means clustering. For each region, the leading principal components of the winter maximum 5-d accumulated precipitation anomalies were the predictands. Potential predictors included quasi-global sea surface temperature anomalies and 500 hPa geopotential height anomalies over the Northern Hemisphere, as well as six climate indices (the Nino-3.4 region sea surface temperature, the North Atlantic Oscillation, the Pacific-North American teleconnection, the Pacific Decadal Oscillation, the Scandinavia pattern, and the East Atlantic pattern). The results showed that in general the two robust SVR models tended to have better forecast skills than the two non-robust models (MLR and BNN), and the nonlinear SVR model tended to forecast slightly better than the linear SVR model. Among the six regions, the Prairies region displayed the highest forecast skills, and the Arctic region the second highest. The strongest nonlinearity was manifested over the Prairies and the weakest nonlinearity over the Arctic.
更多查看译文
关键词
multiple linear regression,linear regression model,seasonality,support vector regression,north atlantic oscillation,principal component,pacific decadal oscillation,sea surface temperature,k means clustering,neural network
AI 理解论文
溯源树
样例
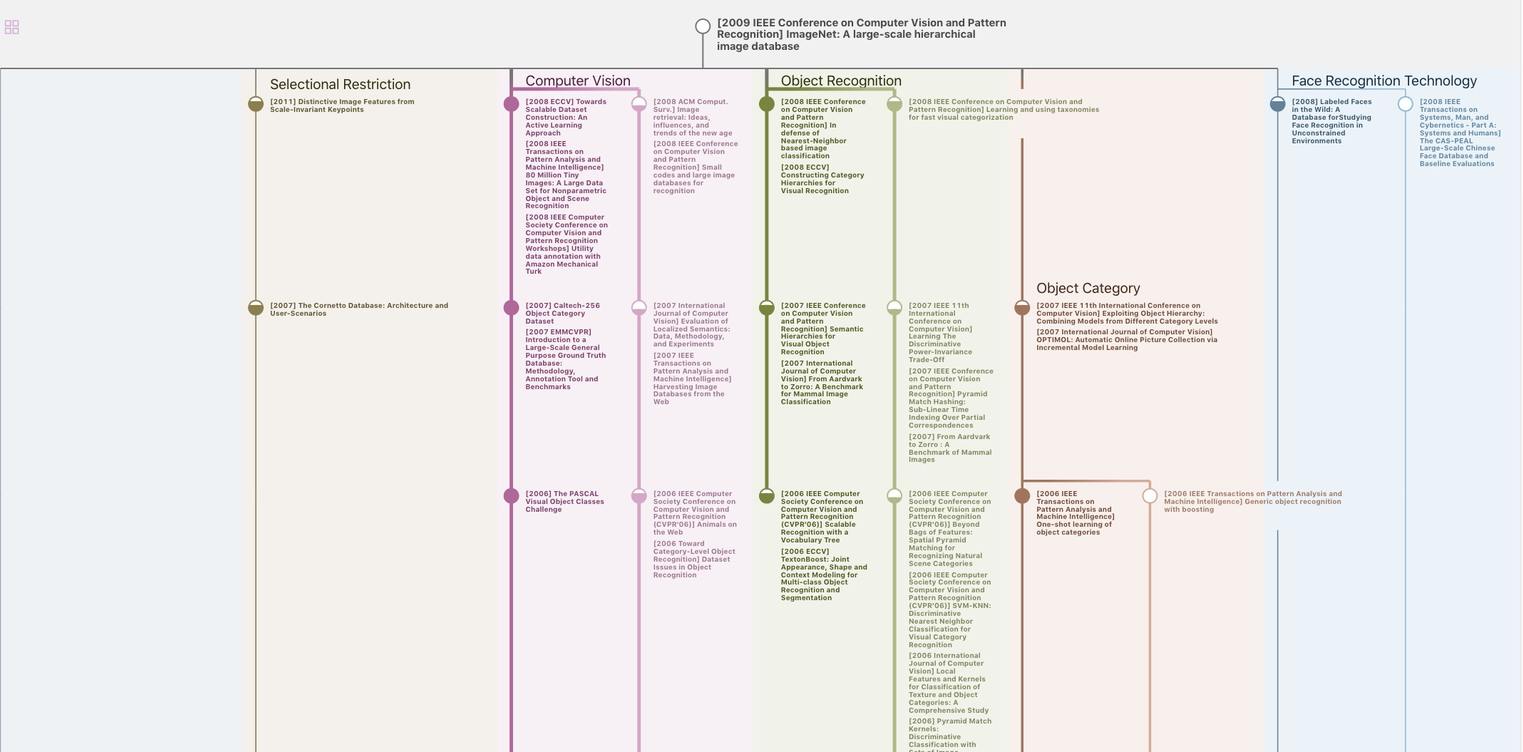
生成溯源树,研究论文发展脉络
Chat Paper
正在生成论文摘要