ProteinInferencer: Confident protein identification and multiple experiment comparison for large scale proteomics projects
Journal of Proteomics(2015)
Abstract
Shotgun proteomics generates valuable information from large-scale and target protein characterizations, including protein expression, protein quantification, protein post-translational modifications (PTMs), protein localization, and protein–protein interactions. Typically, peptides derived from proteolytic digestion, rather than intact proteins, are analyzed by mass spectrometers because peptides are more readily separated, ionized and fragmented. The amino acid sequences of peptides can be interpreted by matching the observed tandem mass spectra to theoretical spectra derived from a protein sequence database. Identified peptides serve as surrogates for their proteins and are often used to establish what proteins were present in the original mixture and to quantify protein abundance. Two major issues exist for assigning peptides to their originating protein. The first issue is maintaining a desired false discovery rate (FDR) when comparing or combining multiple large datasets generated by shotgun analysis and the second issue is properly assigning peptides to proteins when homologous proteins are present in the database. Herein we demonstrate a new computational tool, ProteinInferencer, which can be used for protein inference with both small- or large-scale data sets to produce a well-controlled protein FDR. In addition, ProteinInferencer introduces confidence scoring for individual proteins, which makes protein identifications evaluable.
MoreTranslated text
Key words
Proteomics,Mass spectrometry,Database search,Protein inference,False discovery rate (FDR),Peptide-spectrum match (PSM)
AI Read Science
Must-Reading Tree
Example
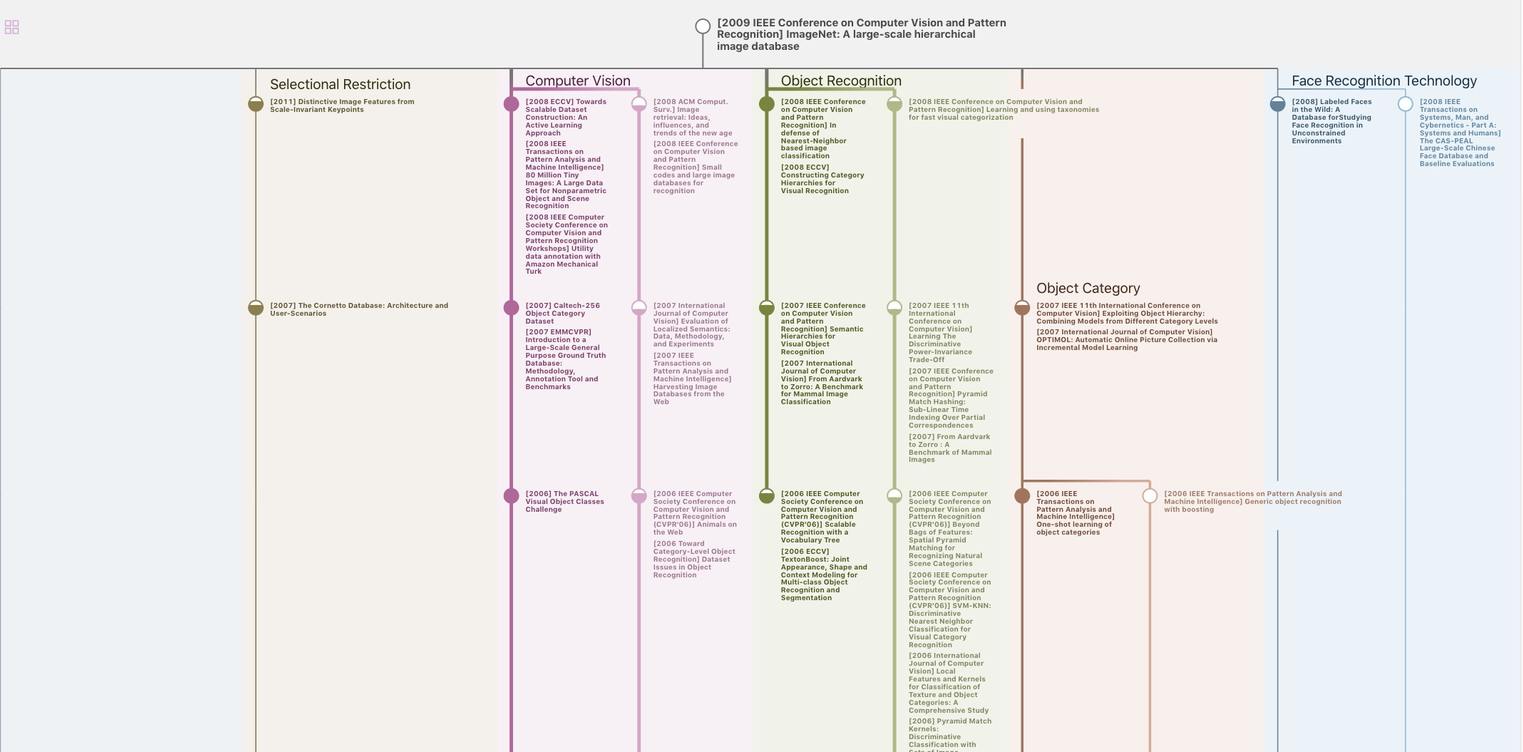
Generate MRT to find the research sequence of this paper
Chat Paper
Summary is being generated by the instructions you defined