A Model Ranking Based Selective Ensemble Approach for Time Series Forecasting
Procedia Computer Science(2015)
摘要
Time series analysis is a highly active research topic that encompasses various domains of science, engineering, and finance. A major challenge in this field is to obtain reasonably accurate forecasts of future data from analyzing the past records. A fruitful alternative to using a single forecasting technique is to combine the forecasts from several conceptually different models. Numerous research studies in literature strongly recommend this approach, due to the fact that a combination of multiple forecasts almost always substantially reduces the overall forecasting errors as well as outperforms the component models. In this paper, we propose an ensemble method that selectively combines some of the constituent forecasting models, instead of combining all of them. On each time series, the component models are successively ranked as per their past forecasting accuracies and then we combine the forecasts of a group of high ranked models. Empirical analysis is conducted with nine individual models and four real-world time series datasets. Results clearly show that our proposed ensemble mechanism achieves consistently better accuracies than all component models and other conventional forecasts combination schemes.
更多查看译文
关键词
Time series,Combination of forecasts,Forecasting accuracy,Box-Jenkins models,Neural networks,Support vector machines.
AI 理解论文
溯源树
样例
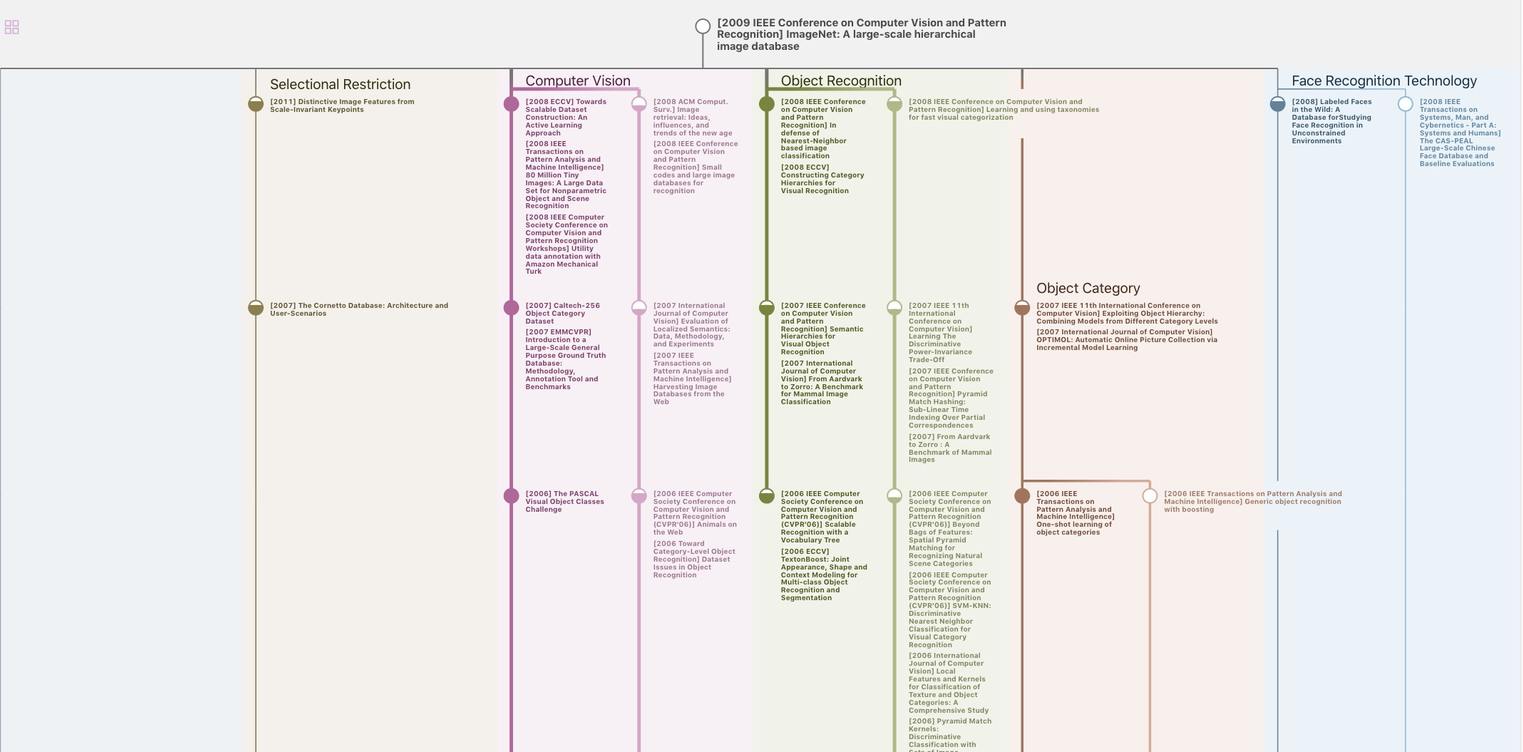
生成溯源树,研究论文发展脉络
Chat Paper
正在生成论文摘要