Multi-Attribute NMS: An Enhanced Non-Maximum Suppression Algorithm for Pedestrian Detection in Crowded Scenes
APPLIED SCIENCES-BASEL(2023)
Abstract
Removing duplicate proposals is a critical process in pedestrian detection, and is usually performed via Non-Maximum Suppression (NMS); however, in crowded scenes, the detection proposals of occluded pedestrians are hard to distinguish from duplicate proposals, making the detection results inaccurate. In order to address the above-mentioned problem, the authors of this paper propose a Multi-Attribute NMS (MA-NMS) algorithm, which combines density and count attributes in order to adaptively adjust suppression, effectively preserving the proposals of occluded pedestrians while removing duplicate proposals. In order to obtain the density and count attributes, an attribute branch (ATTB), which uses a context extraction module (CEM) to extract the context of pedestrians, and then, concatenates the context with the features of pedestrians in order to predict both the density and count attributes simultaneously, is also proposed. With the proposed ATTB, a pedestrian detector, based on MA-NMS, is constructed for pedestrian detection in crowded scenes. Extensive experiments are conducted using the CrowdHuman and CityPersons datasets, and the results show that the proposed method outperforms mainstream methods on AP (average precision), Recall, and MR-2 (log-average miss rate), sufficiently validating the effectiveness of the proposed MA-NMS algorithm.
MoreTranslated text
Key words
pedestrian detection,intra-class occlusion,non-maximum suppression,multi-attribute
AI Read Science
Must-Reading Tree
Example
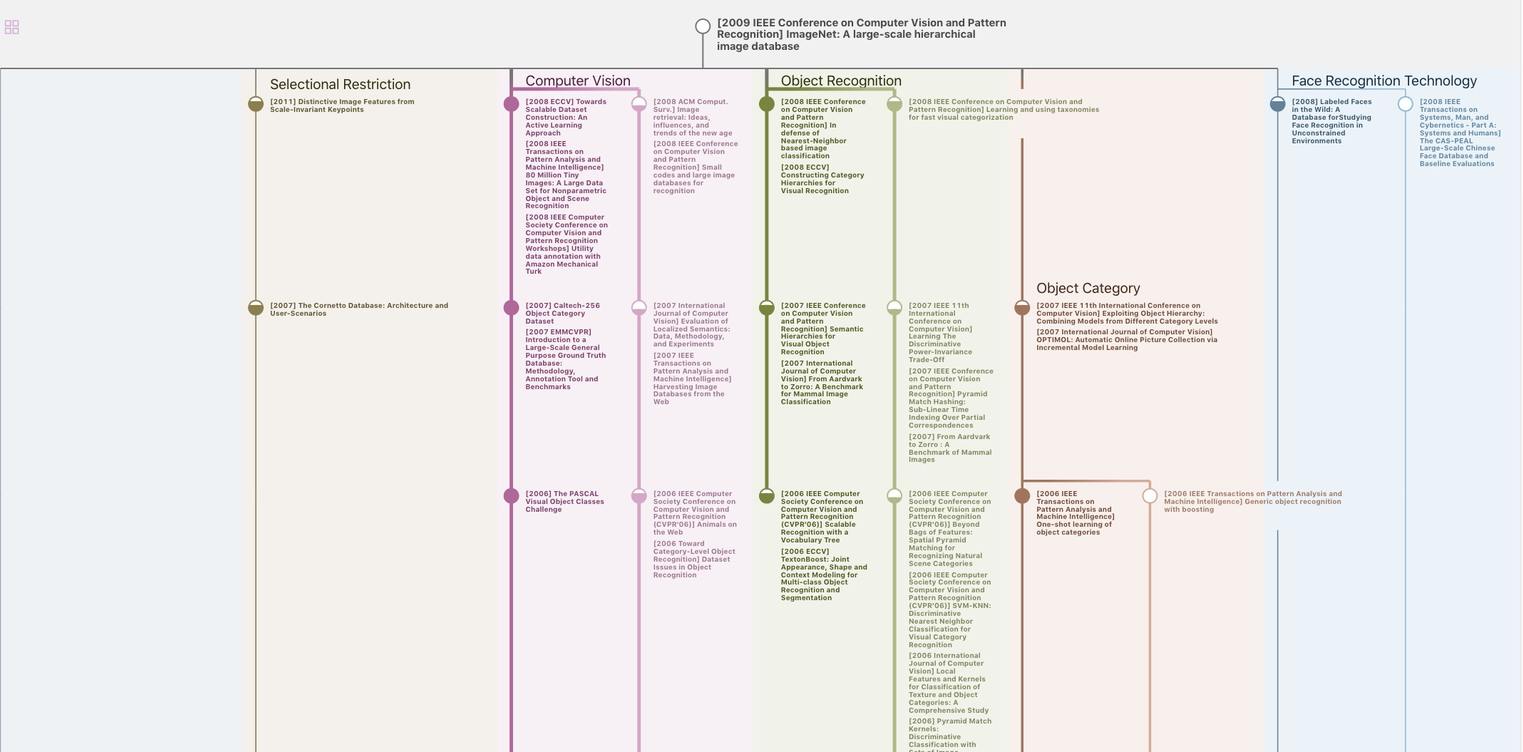
Generate MRT to find the research sequence of this paper
Chat Paper
Summary is being generated by the instructions you defined