Classification of hyperspectral urban data using adaptive simultaneous orthogonal matching pursuit
JOURNAL OF APPLIED REMOTE SENSING(2014)
摘要
Simultaneous orthogonal matching pursuit (SOMP) has been recently developed for hyperspectral image classification. It utilizes a joint sparsity model with the assumption that each pixel can be represented by a linear combination of labeled samples. We present an approach to improve the performance of SOMP based on a priori segmentation map. According to the map, we first build a local region where within-segment pixels are preserved while between-segment pixels are excluded. Hyperspectral pixels in the preserved region around the test pixel are then simultaneously represented by a linear combination of training samples, whose weights are recovered by solving a sparsity-constrained optimization problem. Finally, the label of the test pixel is determined to be the class that yields the minimal total residuals between the test samples and the approximations. Experimental results demonstrate that the proposed adaptive SOMP (ASOMP) is superior to some existing classifiers, such as the original SOMP and the recently proposed weighted-SOMP (WSOMP). For example, the ASOMP performed with an accuracy of 95.53% for the ROSIS University of Pavia data with 120 training samples per class, while SOMP obtained an accuracy of 87.61%, an improvement of approximately 8%. (C) 2014 Society of Photo-Optical Instrumentation Engineers (SPIE)
更多查看译文
关键词
hyperspectral image,sparse representation,segmentation,simultaneous orthogonal matching pursuit
AI 理解论文
溯源树
样例
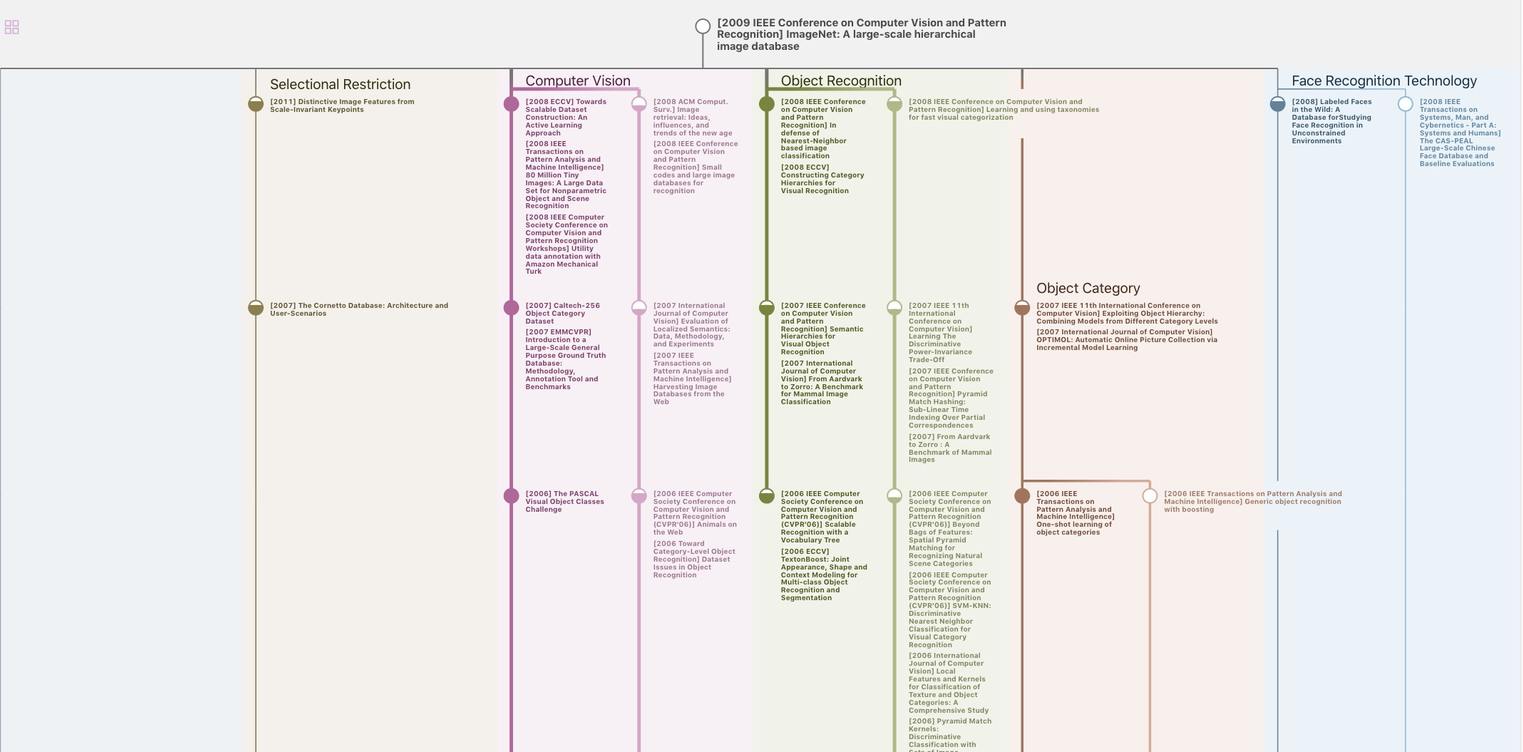
生成溯源树,研究论文发展脉络
Chat Paper
正在生成论文摘要