Data clustering: review and investigation of parallel genetic algorithms for revealing clusters
International Journal of Applied Management Science(2014)
摘要
This paper review some state-of-the art non-parallel and parallel approaches of data clustering and present the usefulness of parallel genetic algorithms (PGAs) for clustering. In solving the clustering problem many traditional methods stuck in local optimal solutions. Further, in such algorithms the user also asked to provide the number of clusters but in general it is unknown to the user. Therefore, clustering becomes a trial-and-error work and is also very expensive in terms of computation time. Genetic algorithm can be a solution to reduce the local optimal problem but it demands very high computation time, hence in this paper, we are using a PGA for data clustering. PGA not only exploits large search space to find the cluster centres but also reduces the computation vastly. Furthermore, this work exploits both data parallelism by distributing the data being mined across all available processors, and control parallelism by distributing the population of individuals across all available processors. Data parallelism coupled with control parallelism has shown to yield the best parallelism results on a diverse set of benchmark real life datasets taken in this article.
更多查看译文
关键词
data parallelism,pga,data mining,genetic algorithm,control parallelism,pgas,clustering
AI 理解论文
溯源树
样例
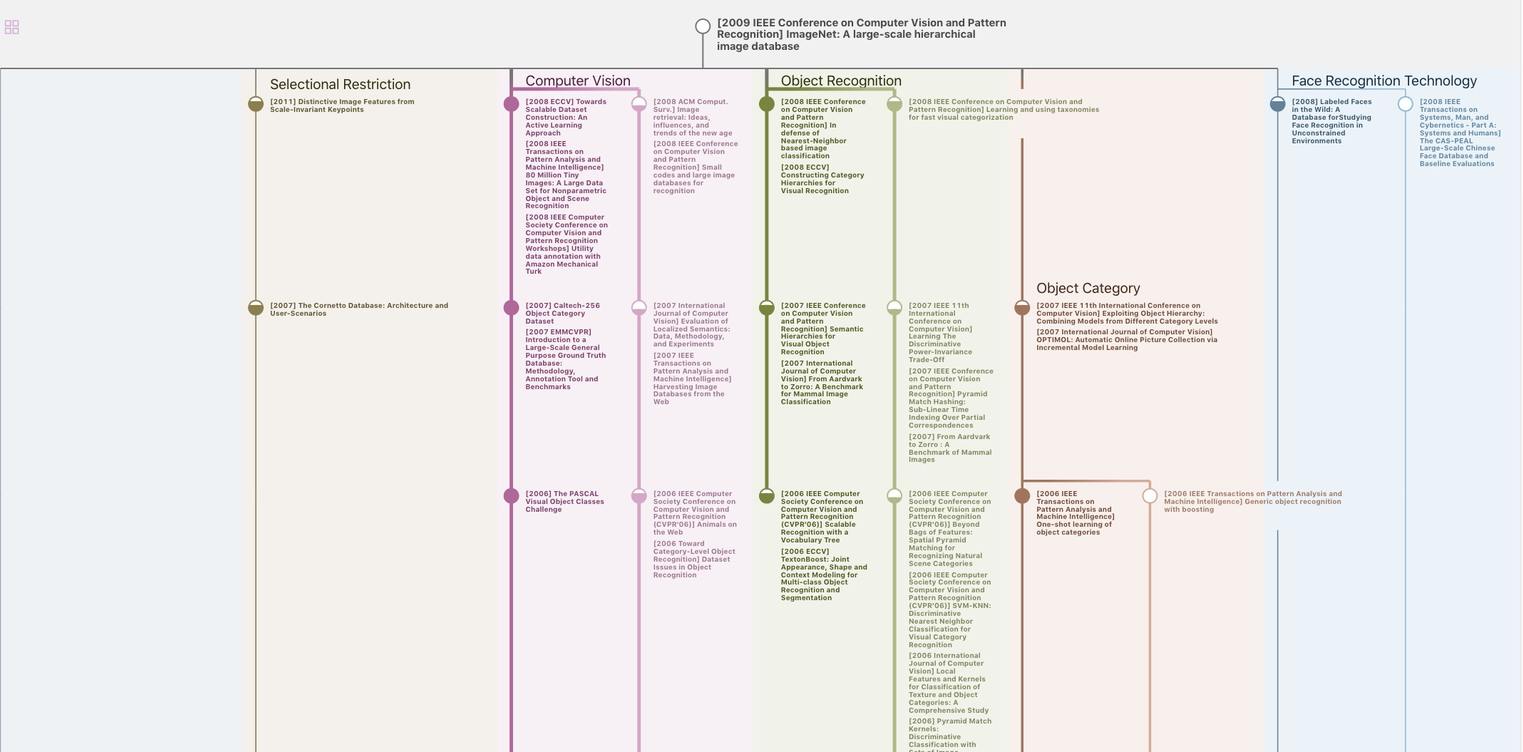
生成溯源树,研究论文发展脉络
Chat Paper
正在生成论文摘要