Probabilistic 0–1-h Convective Initiation Nowcasts that Combine Geostationary Satellite Observations and Numerical Weather Prediction Model Data
JOURNAL OF APPLIED METEOROLOGY AND CLIMATOLOGY(2015)
摘要
The Geostationary Operational Environmental Satellite (GOES)-R convective initiation (CI) algorithm predicts CI in real time over the next 0-60 min. While GOES-R CI has been very successful in tracking nascent clouds and obtaining cloud-top growth and height characteristics relevant to CI in an object-tracking framework, its performance has been hindered by elevated false-alarm rates, and it has not optimally combined satellite observations with other valuable data sources. Presented here are two statistical learning approaches that incorporate numerical weather prediction (NWP) input within the established GOES-R CI framework to produce probabilistic forecasts: logistic regression (LR) and an artificial-intelligence approach known as random forest (RF). Both of these techniques are used to build models that are based on an extensive database of CI events and nonevents and are evaluated via cross validation and on independent case studies. With the proper choice of probability thresholds, both the LR and RF techniques incorporating NWP data produce substantially fewer false alarms than when only GOES data are used. The NWP information identifies environmental conditions (as favorable or unfavorable) for the development of convective storms and improves the skill of the CI nowcasts that operate on GOES-based cloud objects, as compared with when the satellite IR fields are used alone. The LR procedure performs slightly better overall when 14 skill measures are used to quantify the results and notably better on independent case study days.
更多查看译文
关键词
Cumulus clouds,Satellite observations,Statistical techniques,Nowcasting
AI 理解论文
溯源树
样例
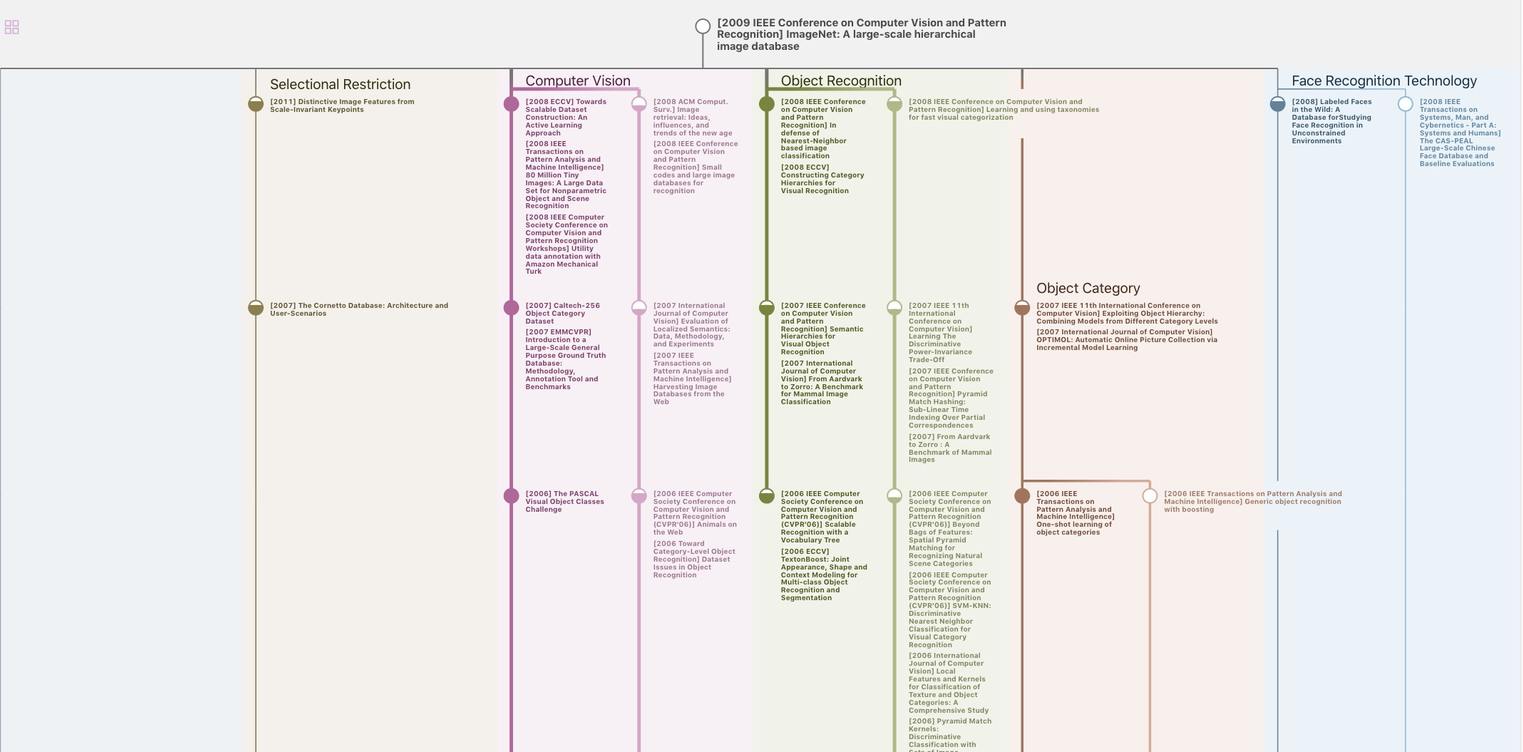
生成溯源树,研究论文发展脉络
Chat Paper
正在生成论文摘要