Evaluating soil biogeochemistry parameterizations in Earth system models with observations
Global Biogeochemical Cycles(2014)
摘要
Soils contain large reservoirs of terrestrial carbon (C), yet soil C dynamics simulated in Earth systems models show little agreement with each other or with observational data sets. This uncertainty underscores the need to develop a framework to more thoroughly evaluate model parameterizations, structures, and projections. Toward this end we used an analytical solution to calculate approximate equilibrium soil C pools for the Community Land Model version 4 (CLM4cn) and Daily Century (DAYCENT) soil biogeochemistry models. Neither model generated sufficient soil C pools when forced with litterfall inputs from CLM4cn; however, global totals and spatial correlations of soil C pools for both models improved when calculated with litterfall inputs derived from observational data. DAYCENT required additional modifications to simulate soil C pools in deeper soils (0-100cm). Our best simulations produced global soil C pools totaling 746 and 978 Pg C for CLM4cn and DAYCENT parameterizations, respectively, compared to observational estimates of 1259 Pg C. In spite of their differences in complexity and equilibrium soil C pools, predictions of soil C losses with warming temperatures through 2100 were strikingly similar for both models. Ultimately, CLM4cn and DAYCENT come from the same class of models that represent the turnover of soil C as a first-order decay process. While this approach may have utility in calculating steady state soil C pools, the applicability of this model configuration in transient simulations remains poorly evaluated. Key Points
更多查看译文
关键词
soil biogeochemistry,Earth system model,soil organic matter,model benchmark,carbon-climate feedback,Community Land Model
AI 理解论文
溯源树
样例
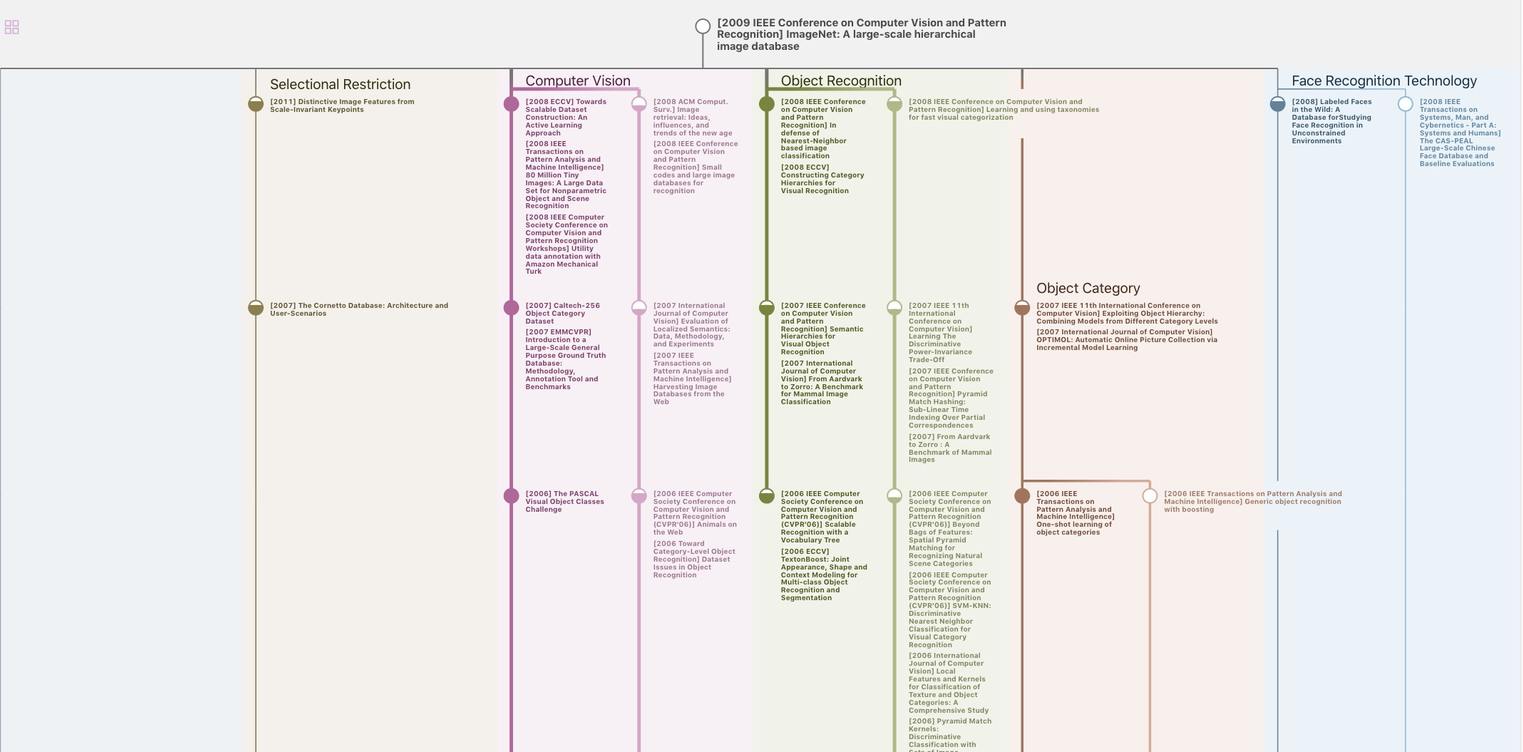
生成溯源树,研究论文发展脉络
Chat Paper
正在生成论文摘要