Validation Of A Radiosensitivity Gene Signature In Radiation Therapy-Treated Patients From The Cancer Genome Atlas (Tcga)
INTERNATIONAL JOURNAL OF RADIATION ONCOLOGY BIOLOGY PHYSICS(2014)
摘要
Purpose/Objective(s)Gene expression signature for predicting radiosensitivity has been developed to subclassify tumor characteristics. Recently, a radiosensitivity gene signature, which includes 31 genes derived by integrating four different microarray experiments, has been found to have a potential for predicting radiosensitivity of cancer cells, but it was seldom validated in clinical cancer samples. In our study, we proposed that the gene signature may serve as a predictive biomarker for estimating the overall survival of radiation-treated patients. The significance of gene signature was tested in independent datasets with The Cancer Genome Atlas (TCGA), which is the most powerful cancer information database in the world.Materials/MethodsWe downloaded the mRNA expression profiling of the 16 different cancer types and corresponding clinical information from TCGA. After filtering out cancer types without radiation therapy information or insufficient clinical survival information, the 31 gene signature was applied to three independent datasets: glioblastoma multiforme (GBM), low-grade glioma (LGG), and head and neck squamous cell carcinoma (HNSCC). There were a total of 708 samples treated with radiation therapy, including 465 patients with GBM, 92 patients with HNSCC, and 151 patients with LGG, respectively. The patients were stratified into radiosensitive (RS) and radioresistant (RR) groups according to the above-mentioned 31-gene signature. The Kaplan-Meier method was used to estimate survival time and multivariate analysis was carried out using Cox proportional hazard model.ResultsAll the RT-treated patients were stratified into radiosensitive (RS) and radioresistant (RR) groups according to radiosensitivity gene signature. Patients assigned to RS group had an improved overall survival compared with RR group in GBM (median survival days: RS vs RR, 562 d vs 448 d, p = 0.001, HR = 1.568, 95% CI = 1.207-2.038) and HNSCC (median survival days: RS vs RR, 3314 d vs 2318 d, p = 0.004, HR = 3.960, 95% CI = 1.447-10.833) cohorts. The gene radiosensitivity gene signature has been proved to be an independent predictor, when considering age, KPS, molecular subtype, and additional chemotherapy as covariates for GBM (p = 0.015), and age and pathologic stage for HNSCC (p = 0.020). No significant difference of survival time was observed between the predicted RS and RR patients with LGG (p = 0.128).ConclusionsThis is the first study to use a radiosensitivity gene signature model to predict radiation-treated patients’ prognostic in three independent cohorts from TCGA. Although promising, this model needed to be further clinically validated in the routine diagnostic of GBM and HNSCC for an optimal treatment decision. Purpose/Objective(s)Gene expression signature for predicting radiosensitivity has been developed to subclassify tumor characteristics. Recently, a radiosensitivity gene signature, which includes 31 genes derived by integrating four different microarray experiments, has been found to have a potential for predicting radiosensitivity of cancer cells, but it was seldom validated in clinical cancer samples. In our study, we proposed that the gene signature may serve as a predictive biomarker for estimating the overall survival of radiation-treated patients. The significance of gene signature was tested in independent datasets with The Cancer Genome Atlas (TCGA), which is the most powerful cancer information database in the world. Gene expression signature for predicting radiosensitivity has been developed to subclassify tumor characteristics. Recently, a radiosensitivity gene signature, which includes 31 genes derived by integrating four different microarray experiments, has been found to have a potential for predicting radiosensitivity of cancer cells, but it was seldom validated in clinical cancer samples. In our study, we proposed that the gene signature may serve as a predictive biomarker for estimating the overall survival of radiation-treated patients. The significance of gene signature was tested in independent datasets with The Cancer Genome Atlas (TCGA), which is the most powerful cancer information database in the world. Materials/MethodsWe downloaded the mRNA expression profiling of the 16 different cancer types and corresponding clinical information from TCGA. After filtering out cancer types without radiation therapy information or insufficient clinical survival information, the 31 gene signature was applied to three independent datasets: glioblastoma multiforme (GBM), low-grade glioma (LGG), and head and neck squamous cell carcinoma (HNSCC). There were a total of 708 samples treated with radiation therapy, including 465 patients with GBM, 92 patients with HNSCC, and 151 patients with LGG, respectively. The patients were stratified into radiosensitive (RS) and radioresistant (RR) groups according to the above-mentioned 31-gene signature. The Kaplan-Meier method was used to estimate survival time and multivariate analysis was carried out using Cox proportional hazard model. We downloaded the mRNA expression profiling of the 16 different cancer types and corresponding clinical information from TCGA. After filtering out cancer types without radiation therapy information or insufficient clinical survival information, the 31 gene signature was applied to three independent datasets: glioblastoma multiforme (GBM), low-grade glioma (LGG), and head and neck squamous cell carcinoma (HNSCC). There were a total of 708 samples treated with radiation therapy, including 465 patients with GBM, 92 patients with HNSCC, and 151 patients with LGG, respectively. The patients were stratified into radiosensitive (RS) and radioresistant (RR) groups according to the above-mentioned 31-gene signature. The Kaplan-Meier method was used to estimate survival time and multivariate analysis was carried out using Cox proportional hazard model. ResultsAll the RT-treated patients were stratified into radiosensitive (RS) and radioresistant (RR) groups according to radiosensitivity gene signature. Patients assigned to RS group had an improved overall survival compared with RR group in GBM (median survival days: RS vs RR, 562 d vs 448 d, p = 0.001, HR = 1.568, 95% CI = 1.207-2.038) and HNSCC (median survival days: RS vs RR, 3314 d vs 2318 d, p = 0.004, HR = 3.960, 95% CI = 1.447-10.833) cohorts. The gene radiosensitivity gene signature has been proved to be an independent predictor, when considering age, KPS, molecular subtype, and additional chemotherapy as covariates for GBM (p = 0.015), and age and pathologic stage for HNSCC (p = 0.020). No significant difference of survival time was observed between the predicted RS and RR patients with LGG (p = 0.128). All the RT-treated patients were stratified into radiosensitive (RS) and radioresistant (RR) groups according to radiosensitivity gene signature. Patients assigned to RS group had an improved overall survival compared with RR group in GBM (median survival days: RS vs RR, 562 d vs 448 d, p = 0.001, HR = 1.568, 95% CI = 1.207-2.038) and HNSCC (median survival days: RS vs RR, 3314 d vs 2318 d, p = 0.004, HR = 3.960, 95% CI = 1.447-10.833) cohorts. The gene radiosensitivity gene signature has been proved to be an independent predictor, when considering age, KPS, molecular subtype, and additional chemotherapy as covariates for GBM (p = 0.015), and age and pathologic stage for HNSCC (p = 0.020). No significant difference of survival time was observed between the predicted RS and RR patients with LGG (p = 0.128). ConclusionsThis is the first study to use a radiosensitivity gene signature model to predict radiation-treated patients’ prognostic in three independent cohorts from TCGA. Although promising, this model needed to be further clinically validated in the routine diagnostic of GBM and HNSCC for an optimal treatment decision. This is the first study to use a radiosensitivity gene signature model to predict radiation-treated patients’ prognostic in three independent cohorts from TCGA. Although promising, this model needed to be further clinically validated in the routine diagnostic of GBM and HNSCC for an optimal treatment decision.
更多查看译文
关键词
Cancer Imaging,Tumor Microenvironment,Tumor Heterogeneity,Gene Expression
AI 理解论文
溯源树
样例
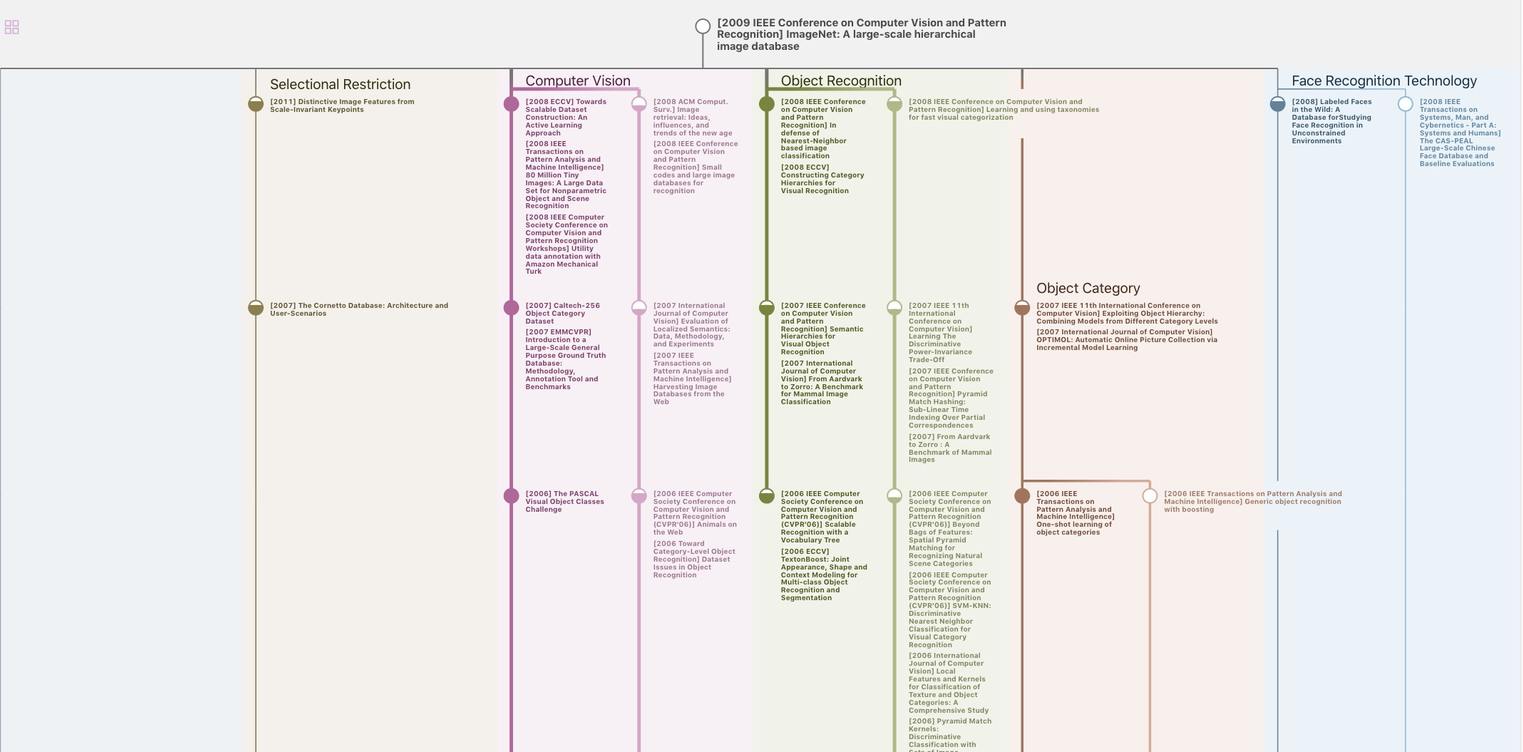
生成溯源树,研究论文发展脉络
Chat Paper
正在生成论文摘要