Using Wearable Sensors and Machine Learning Models to Separate Functional Upper Extremity Use From Walking-Associated Arm Movements
Archives of Physical Medicine and Rehabilitation(2016)
摘要
OBJECTIVE:To improve measurement of upper extremity (UE) use in the community by evaluating the feasibility of using body-worn sensor data and machine learning models to distinguish productive prehensile and bimanual UE activity use from extraneous movements associated with walking.
DESIGN:Comparison of machine learning classification models with criterion standard of manually scored videos of performance in UE prosthesis users.
SETTING:Rehabilitation hospital training apartment.
PARTICIPANTS:Convenience sample of UE prosthesis users (n=5) and controls (n=13) similar in age and hand dominance (N=18).
INTERVENTIONS:Participants were filmed executing a series of functional activities; a trained observer annotated each frame to indicate either UE movement directed at functional activity or walking. Synchronized data from an inertial sensor attached to the dominant wrist were similarly classified as indicating either a functional use or walking. These data were used to train 3 classification models to predict the functional versus walking state given the associated sensor information. Models were trained over 4 trials: on UE amputees and controls and both within subject and across subject. Model performance was also examined with and without preprocessing (centering) in the across-subject trials.
MAIN OUTCOME MEASURE:Percent correct classification.
RESULTS:With the exception of the amputee/across-subject trial, at least 1 model classified >95% of test data correctly for all trial types. The top performer in the amputee/across-subject trial classified 85% of test examples correctly.
CONCLUSIONS:We have demonstrated that computationally lightweight classification models can use inertial data collected from wrist-worn sensors to reliably distinguish prosthetic UE movements during functional use from walking-associated movement. This approach has promise in objectively measuring real-world UE use of prosthetic limbs and may be helpful in clinical trials and in measuring response to treatment of other UE pathologies.
更多查看译文
关键词
Amputation,Artificial intelligence,Artificial limbs,Outcome assessment (health care),Rehabilitation,Task performance and analysis,Upper extremity
AI 理解论文
溯源树
样例
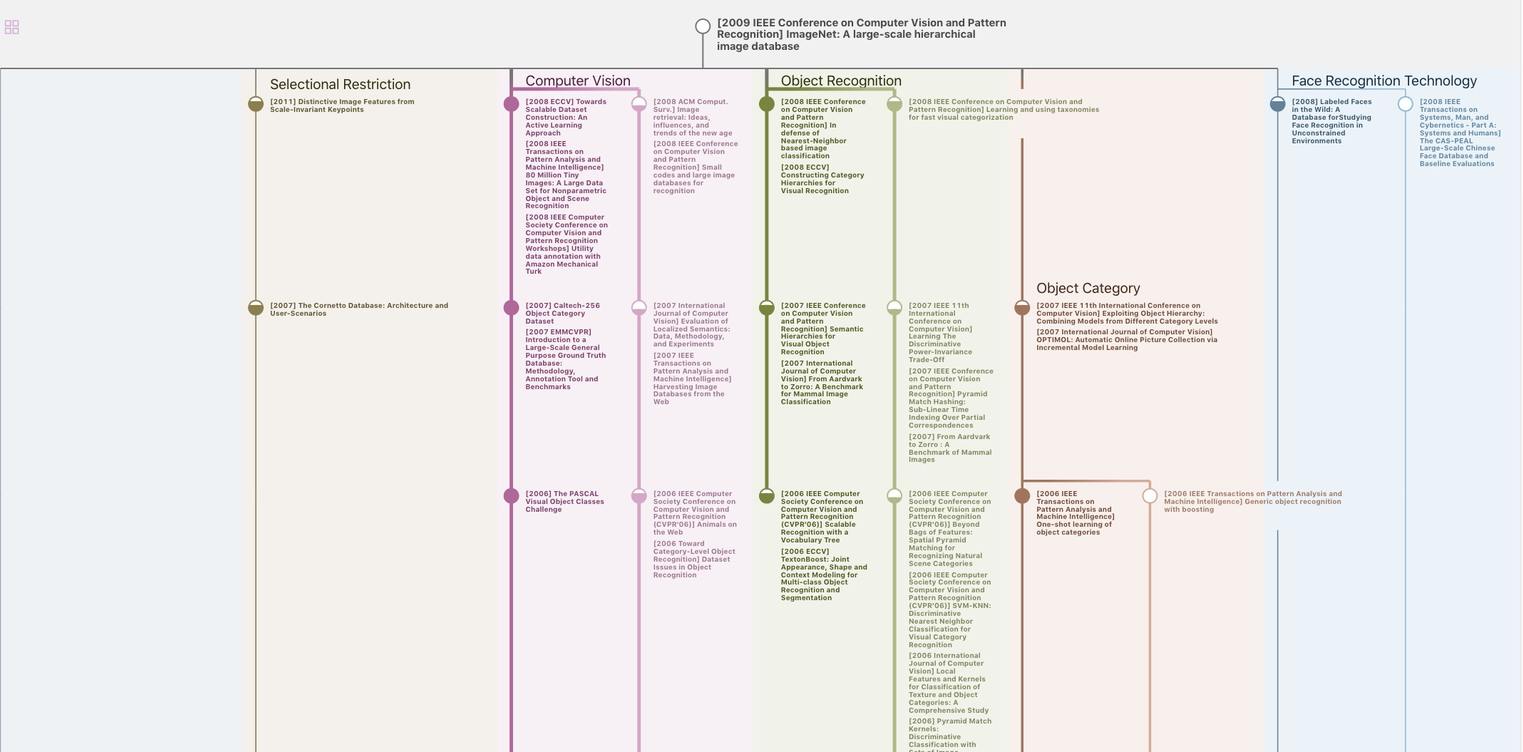
生成溯源树,研究论文发展脉络
Chat Paper
正在生成论文摘要