An Adaptive And Efficient Greedy Procedure For The Optimal Training Of Parametric Reduced-Order Models
INTERNATIONAL JOURNAL FOR NUMERICAL METHODS IN ENGINEERING(2015)
摘要
An adaptive and efficient approach for constructing reduced-order models (ROMs) that are robust to changes in parameters is developed. The approach is based on a greedy sampling of the underlying high-dimensional model (HDM) together with an efficient procedure for exploring the configuration space and identifying parameters for which the error is likely to be high. Because this exploration is based on a surrogate model for an error indicator, it is amenable to a fast training phase. Furthermore, a model for the exact error based on the error indicator is constructed and used to determine when the greedy procedure reaches a desired error tolerance. An efficient procedure for updating the reduced-order basis constructed by proper orthogonal decomposition is also introduced in this paper, avoiding the cost associated with computing large-scale singular value decompositions. The proposed procedure is then shown to require less evaluations of a posteriori error estimators than the classical procedure in order to identify locations of the parameter space to be sampled. It is illustrated on the training of parametric ROMs for three linear and nonlinear mechanical systems, including the realistic prediction of the response of a V-hull vehicle to underbody blasts. Copyright (c) 2014 John Wiley & Sons, Ltd.
更多查看译文
关键词
projection-based model reduction, error estimation, adaptive POD-greedy procedure, adaptive sampling, surrogate modeling
AI 理解论文
溯源树
样例
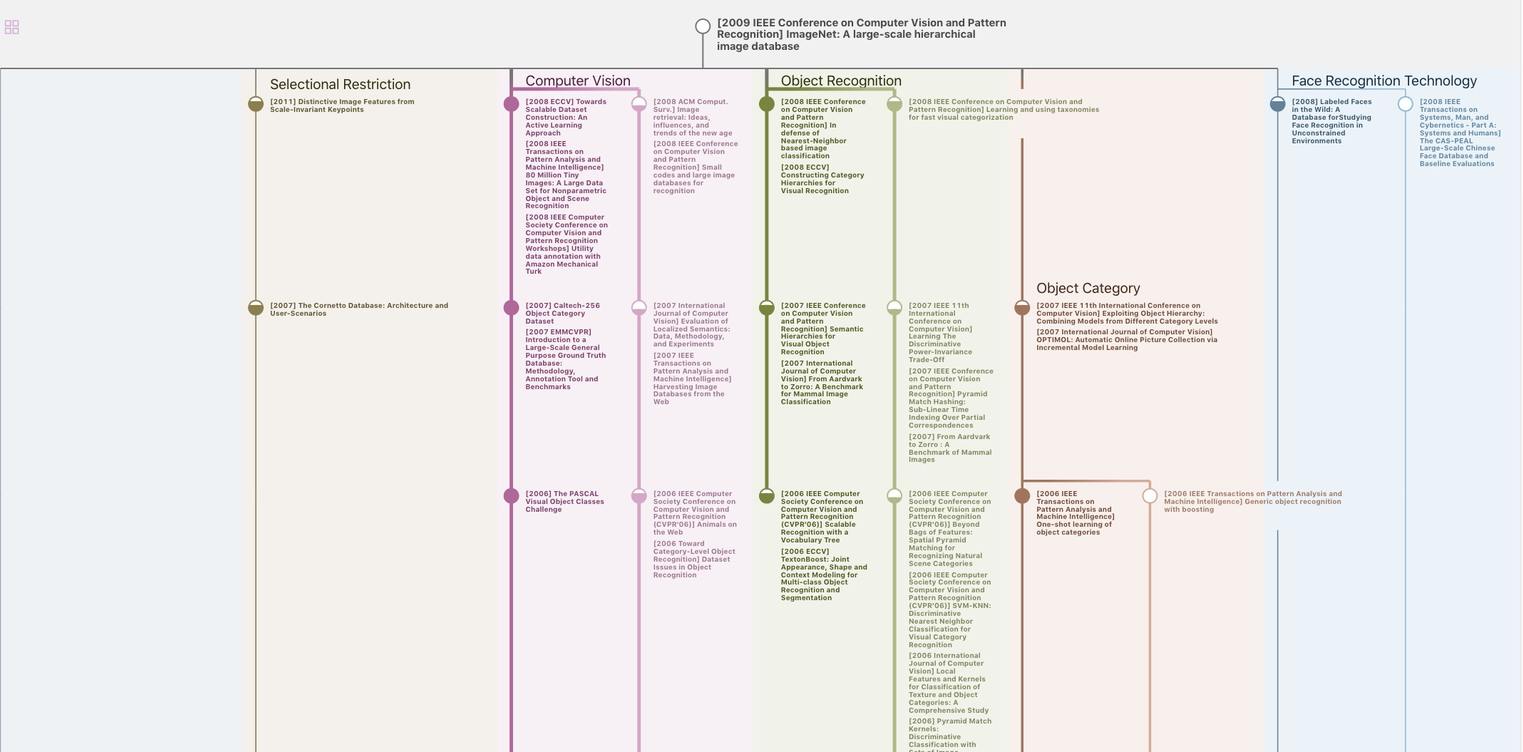
生成溯源树,研究论文发展脉络
Chat Paper
正在生成论文摘要