Reconstructing input for artificial neural networks based on embedding theory and mutual information to simulate soil porewater salinity in tidal floodplain
WATER RESOURCES RESEARCH(2016)
Abstract
Soil pore water salinity plays an important role in the distribution of vegetation and biogeochemical processes in coastal floodplain ecosystems. In this study, artificial neural networks (ANNs) were applied to simulate the pore water salinity of a tidal floodplain in Florida. We present an approach based on embedding theory with mutual information to reconstruct ANN model input time series from one system state variable. Mutual information between system output and input was computed and the local minimum mutual information points were used to determine a time lag vector for time series embedding and reconstruction, with which the mutual information weighted average method was developed to compute the components of reconstructed time series. The optimal embedding dimension was obtained by optimizing model performance. The method was applied to simulate soil pore water salinity dynamics at 12 probe locations in the tidal floodplain influenced by saltwater intrusion using 4 years (2005-2008) data, in which adjacent river water salinity was used to reconstruct model input. The simulated electrical conductivity of the pore water showed close agreement with field observations (RMSE <= 0.031 S/m and R-2 >= 0.897), suggesting the reconstructed input by the proposed approach provided adequate input information for ANN modeling. Multiple linear regression model, partial mutual information algorithm for input variable selection, k-NN algorithm, and simple time delay embedding were also used to further verify the merit of the proposed approach.
MoreTranslated text
Key words
artificial neural networks,mutual information,embedding
AI Read Science
Must-Reading Tree
Example
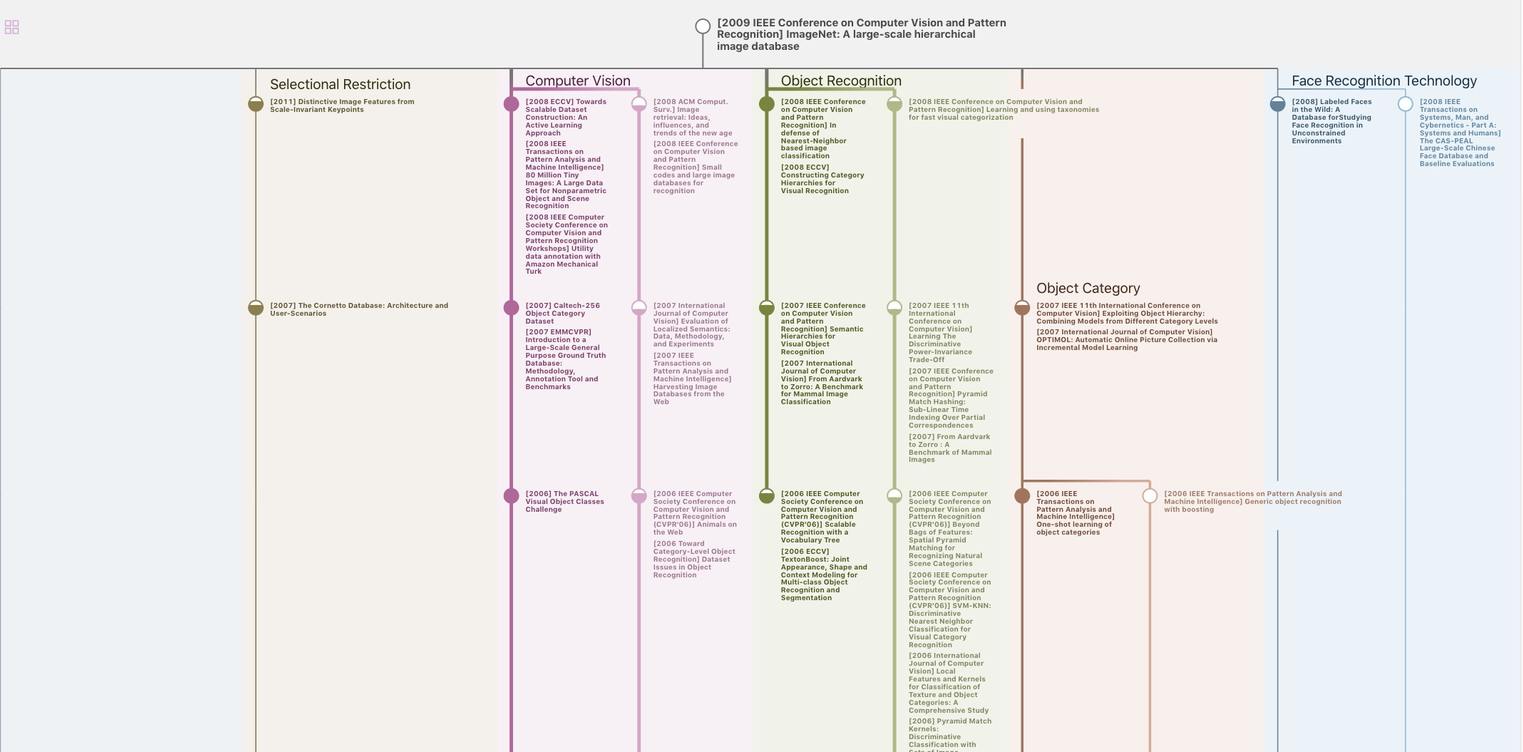
Generate MRT to find the research sequence of this paper
Chat Paper
Summary is being generated by the instructions you defined