Machine learning and linear regression models to predict catchment‐level base cation weathering rates across the southern Appalachian Mountain region, USA
WATER RESOURCES RESEARCH(2014)
摘要
Accurate estimates of soil mineral weathering are required for regional critical load (CL) modeling to identify ecosystems at risk of the deleterious effects from acidification. Within a correlative modeling framework, we used modeled catchment-level base cation weathering (BCw) as the response variable to identify key environmental correlates and predict a continuous map of BCw within the southern Appalachian Mountain region. More than 50 initial candidate predictor variables were submitted to a variety of conventional and machine learning regression models. Predictors included aspects of the underlying geology, soils, geomorphology, climate, topographic context, and acidic deposition rates. Low BCw rates were predicted in catchments with low precipitation, siliceous lithology, low soil clay, nitrogen and organic matter contents, and relatively high levels of canopy cover in mixed deciduous and coniferous forest types. Machine learning approaches, particularly random forest modeling, significantly improved model prediction of catchment-level BCw rates over traditional linear regression, with higher model accuracy and lower error rates. Our results confirmed findings from other studies, but also identified several influential climatic predictor variables, interactions, and nonlinearities among the predictors. Results reported here will be used to support regional sulfur critical loads modeling to identify areas impacted by industrially derived atmospheric S inputs. These methods are readily adapted to other regions where accurate CL estimates are required over broad spatial extents to inform policy and management decisions.
更多查看译文
关键词
base cation weathering,machine learning,sulfur,acid deposition,critical loads,regression
AI 理解论文
溯源树
样例
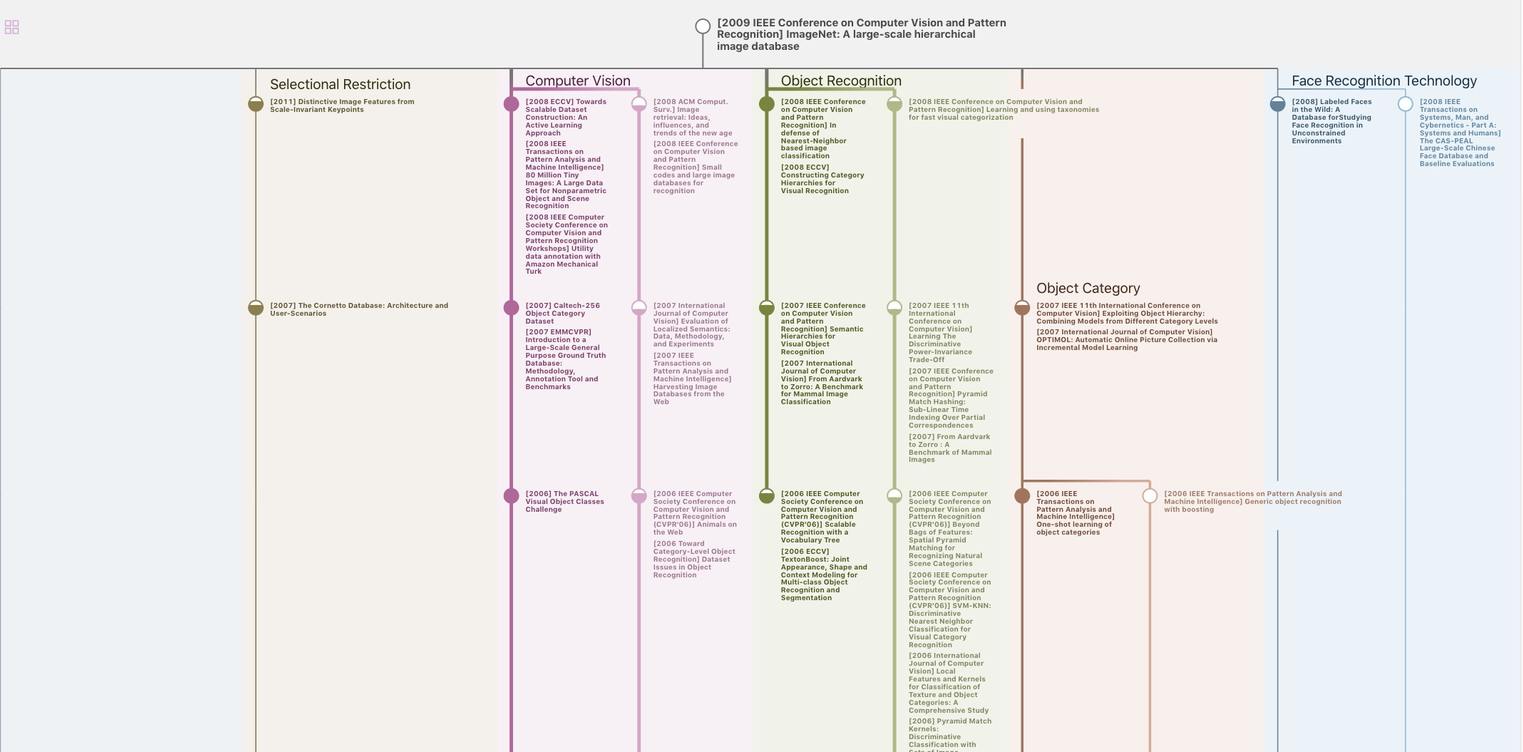
生成溯源树,研究论文发展脉络
Chat Paper
正在生成论文摘要