Experimental Randomization and the Validity of Normal-Theory Inference
Journal of the American Statistical Association(2012)
摘要
This article examines how randomization helps to validate model-based probability statements. The asymptotic validity of normal-theory tests for means in multivariate linear models is established under general conditions. Numerical results are presented showing how randomization provides a limited degree of robustness in small experiments. The conditional relevance of randomization is also discussed. Consider the following additive model for a comparative experiment: Y = θ + GU, where Y is a vector of responses, θ is a vector of treatment effects, U is a vector of unit effects, and G is a permutation representing the assignment of treatments to units. The vector GU is obtained by using G to permute the coordinates of U. It is assumed that θ is fixed and G and U are random and independent. The experimenter observes Y and G, but not θ or U, and chooses the distribution of G, that is, the randomization strategy. In this setup, three probabilities may be considered: conditional given G, conditional given U, and unconditional. Fixing θ and letting ϕ(Y) be an indicator function, we denote the probabilities by E(ϕ | G), E(ϕ | U), and Eϕ. The first probability appears to be the most relevant since it conditions on the experiment that is actually carried out. Unfortunately, E(ϕ | G) often depends on unknown patterns of dependence among the unit effects and hence cannot be evaluated without unreasonable assumptions. The unconditional probability can be evaluated under weaker assumptions because randomization effectively removes many patterns of dependence. If the randomization strategy is appropriate given the knowledge available about the unit effects, then Eϕ is a reasonable substitute for the unknown E(ϕ | G). The main results of the article provide conditions on ϕ and the distributions of U and G so that E(ϕ | U) is close to Eϕ with high probability. Under these conditions the evaluation of Eϕ is insensitive to changes in the distribution of U that preserve the range of typical values of U. Thus the probability depends mainly on the unit-effects model being plausible and not on the exact shape of the distribution. This kind of robustness is useful because the plausibility of a model can be verified, even in small experiments, through residual analysis.
更多查看译文
关键词
random effects,additive model,treatment effect,randomization test,random testing,robustness,asymptotic
AI 理解论文
溯源树
样例
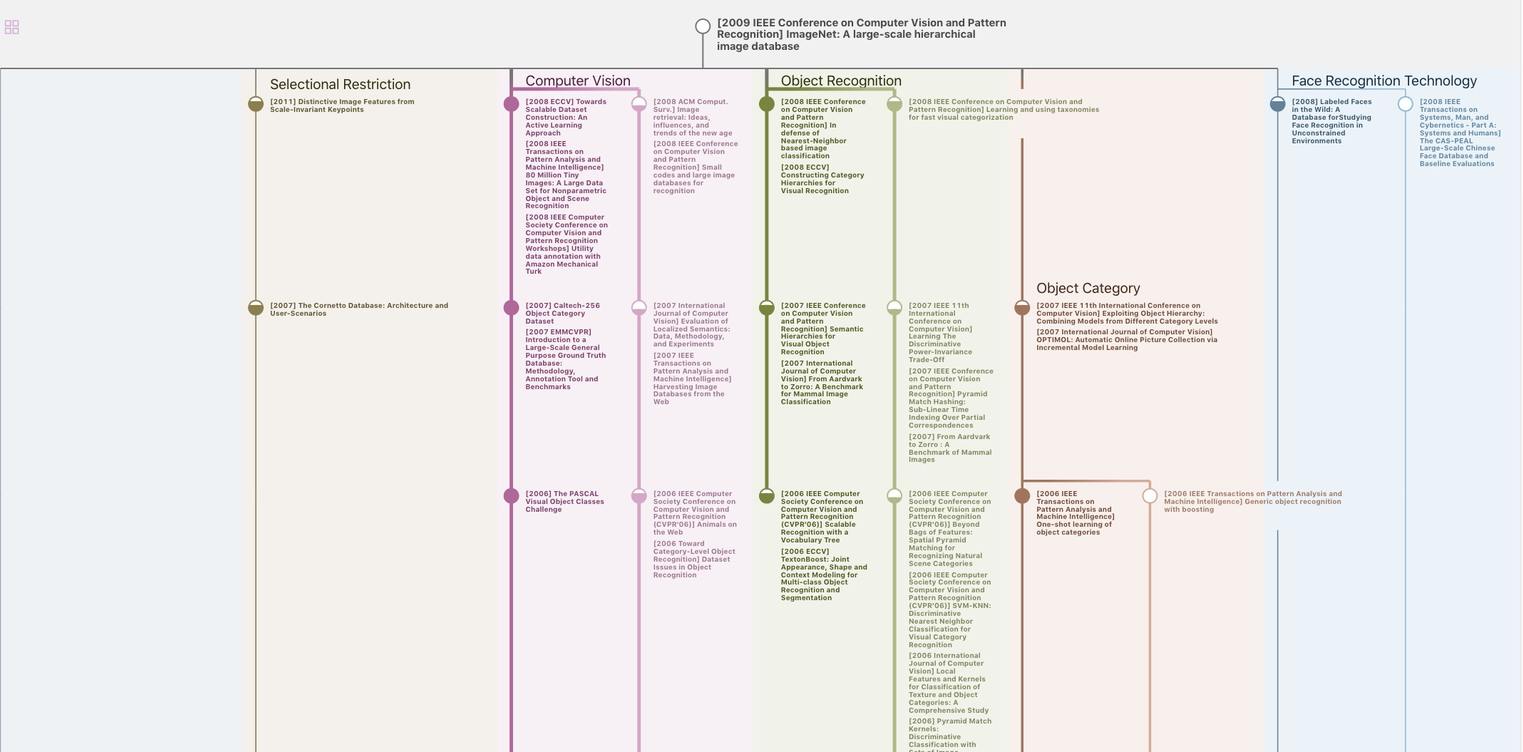
生成溯源树,研究论文发展脉络
Chat Paper
正在生成论文摘要