Monotonically convergent algorithms for symmetric tensor approximation
Linear Algebra and its Applications(2013)
摘要
Reduced rank approximations to symmetric tensors find use in data compaction and in multi-user blind source separation. We derive iterative algorithms which feature monotonic convergence to a minimum of a Frobenius norm approximation criterion, for a certain rank-r Tucker product version of the approximation problem. The approach exploits the gradient inequality for convex functions to establish monotonic convergence, while sparing the cumbersome step size analysis required from a manifold gradient approach. It likewise overcomes some limitations of symmetric versions of alternating least-squares. The computational load per iteration amounts to computing an unfolded matrix and a QR decomposition.
更多查看译文
关键词
Tensor approximation,Tucker product,Polar decomposition,Convex gradient inequality,Monotonic convergence
AI 理解论文
溯源树
样例
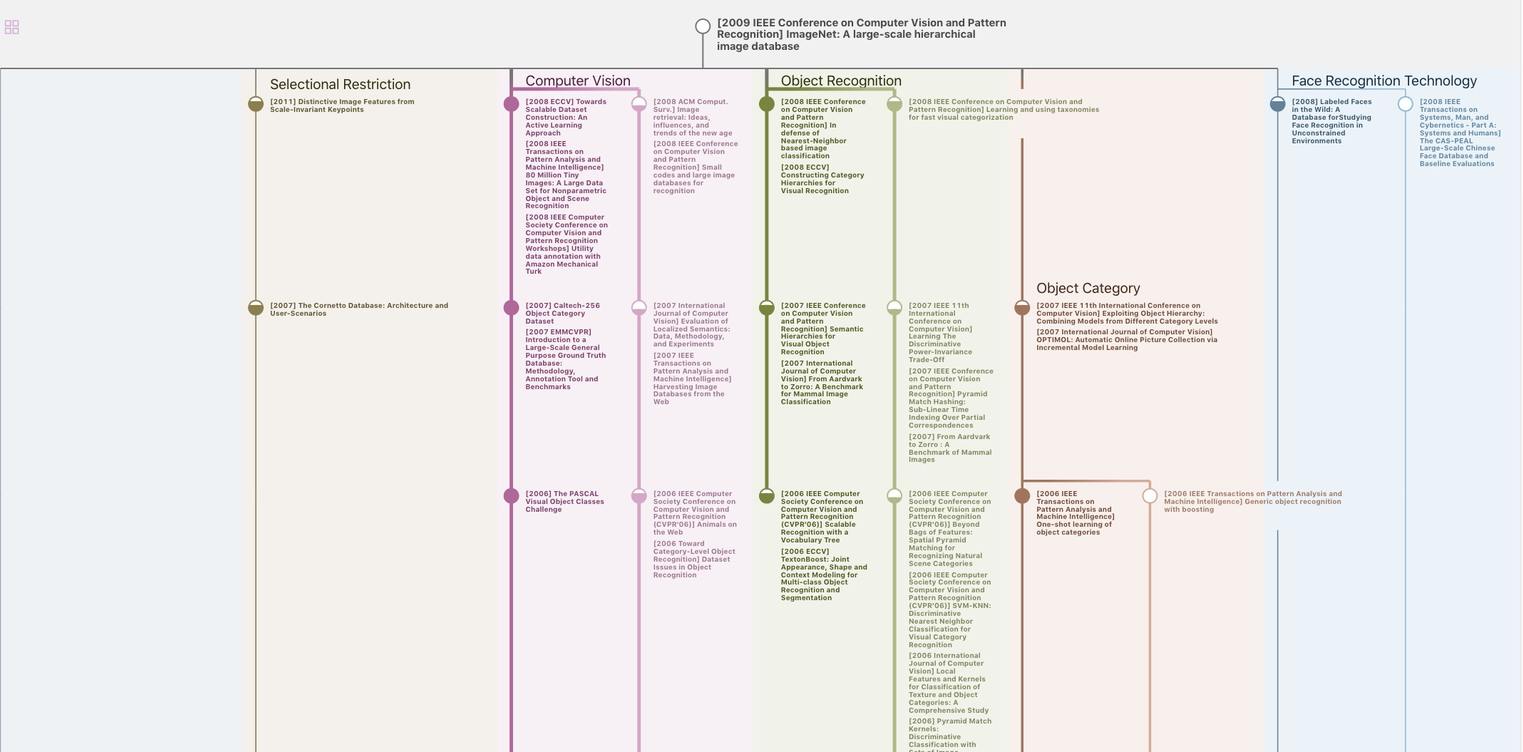
生成溯源树,研究论文发展脉络
Chat Paper
正在生成论文摘要