Reduction of Predictive Uncertainty in Estimating Irrigation Water Requirement Through Multi-Model Ensembles and Ensemble Averaging
GEOSCIENTIFIC MODEL DEVELOPMENT(2015)
Key words
Hydro-Economic Models,Hydrological Modeling,Water Demand,Model Evaluation,Uncertainty Assessment
AI Read Science
Must-Reading Tree
Example
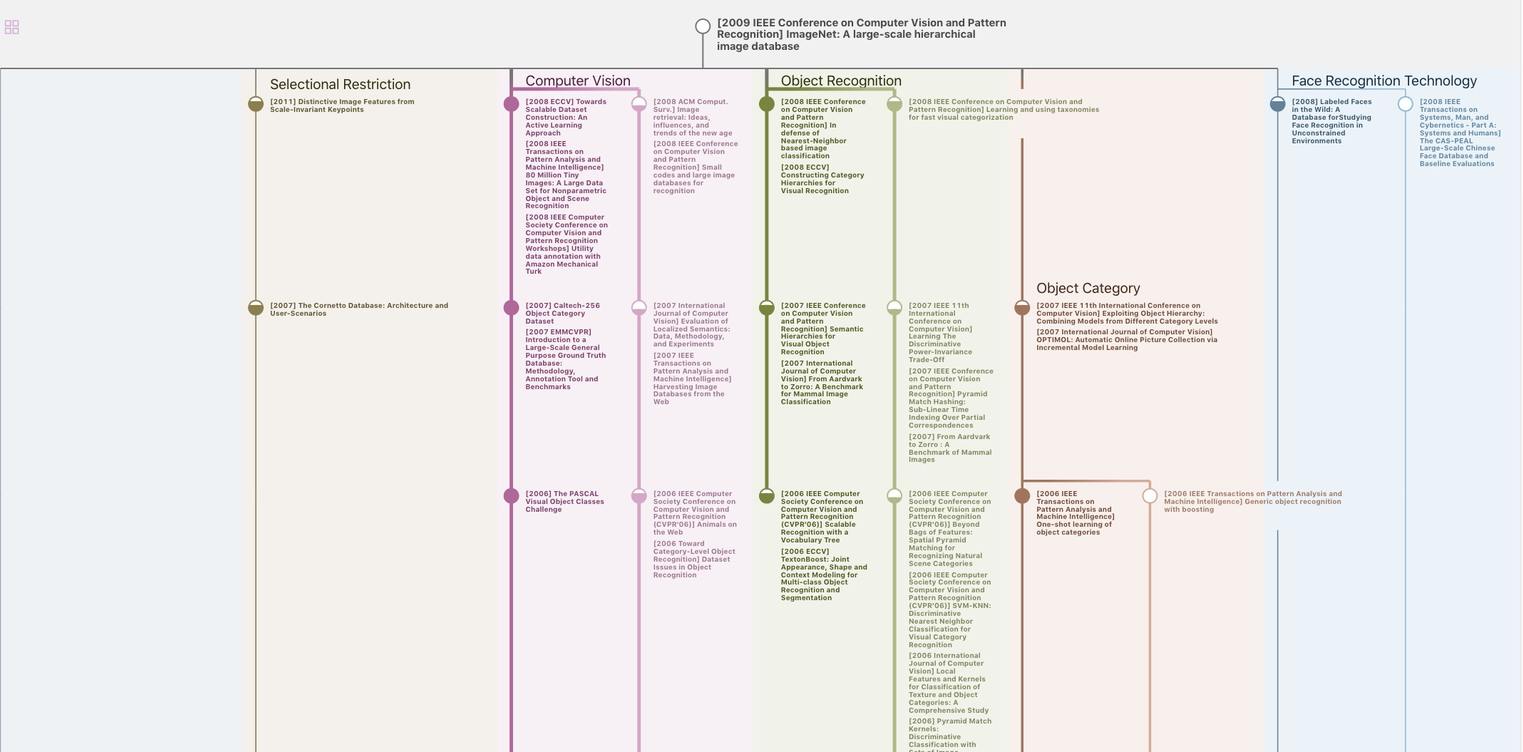
Generate MRT to find the research sequence of this paper
Chat Paper
Summary is being generated by the instructions you defined