Spatial logistic regression and change-of-support in Poisson point processes
ELECTRONIC JOURNAL OF STATISTICS(2010)
Abstract
In Geographical Information Systems, spatial point pattern data are often analysed by dividing space into pixels, recording the presence or absence of points in each pixel, and fitting alogistic regression. We study weaknesses of this approach, propose improvements, and demonstrate an application to prospective geology in Western Australia. Models based on different pixel grids are incompatible (a 'change-of-support' problem) unless the pixels are very small. On a fine pixel grid, a spatial logistic regression is approximately a Poisson point process with log linear intensity; we give explicit distributional bounds. For a loglinear Poisson process, the optimal parameter estimator from pixel data is not spatial logistic regression, but complementary log-log regression with an offset depending on pixel area. If the pixel raster is randomly sub sampled, logistic regression is conditionally optimal. Bias and efficiency depend strongly on the spatial regularity of the covariates. For discontinuous covariates, we propose a new algorithmic strategy in which pixels are subdivided, and demonstrate its efficiency.
MoreTranslated text
Key words
Change of support,complementary log-log regression,ecological fallacy,exponential family,generalized linear models,geographical information systems,likelihood,logistic regression,missing information principle,mixed pixels,mixels,modifiable area unit problem,modulated Poisson process,Poisson point process,prospective geology,prospectivity,spatial point process,spatial statistics,split pixels,Western Australia
AI Read Science
Must-Reading Tree
Example
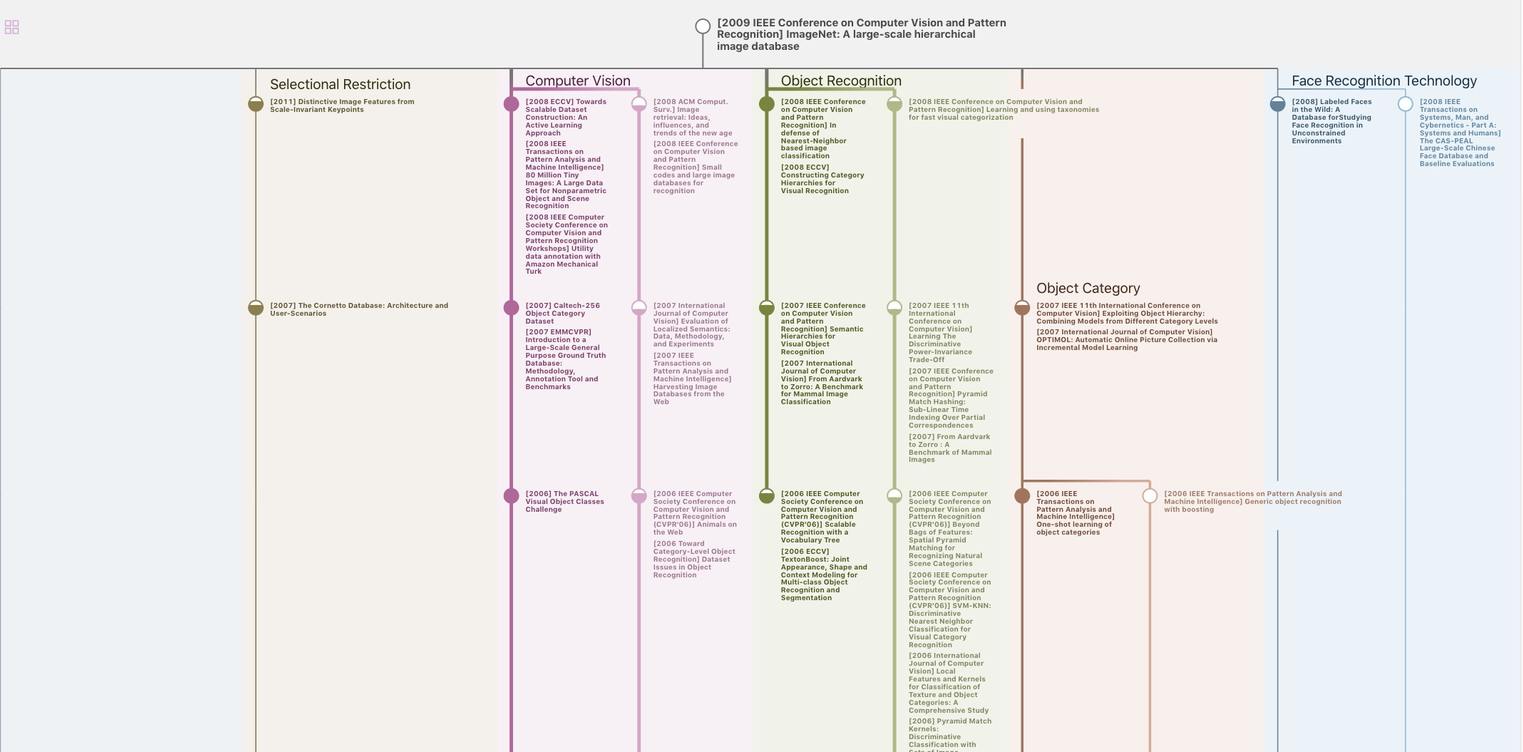
Generate MRT to find the research sequence of this paper
Chat Paper
Summary is being generated by the instructions you defined