Domain Adaptation of SRL Systems for Biological Processes
SIGBIOMED WORKSHOP ON BIOMEDICAL NATURAL LANGUAGE PROCESSING (BIONLP 2019)(2019)
摘要
Domain adaptation remains one of the most challenging aspects in the wide-spread use of Semantic Role Labeling (SRL) systems. Current state-of-the-art methods are typically trained on large-scale datasets, but their performances do not directly transfer to low-resource domain-specific settings. In this paper, we propose two approaches for domain adaptation in biological domain that involve pre-training LSTM-CRF based on existing large-scale datasets and adapting it for a low-resource corpus of biological processes. Our first approach defines a mapping between the source labels and the target labels, and the other approach modifies the final CRF layer in sequence-labeling neural network architecture. We perform our experiments on ProcessBank (Berant et al., 2014) dataset which contains less than 200 paragraphs on biological processes. We improve over the previous state-of-the-art system on this dataset by 21 F1 points. We also show that, by incorporating event-event relationship in ProcessBank, we are able to achieve an additional 2.6 F1 gain, giving us possible insights into how to improve SRL systems for biological process using richer annotations.
更多查看译文
关键词
Subcellular Localization
AI 理解论文
溯源树
样例
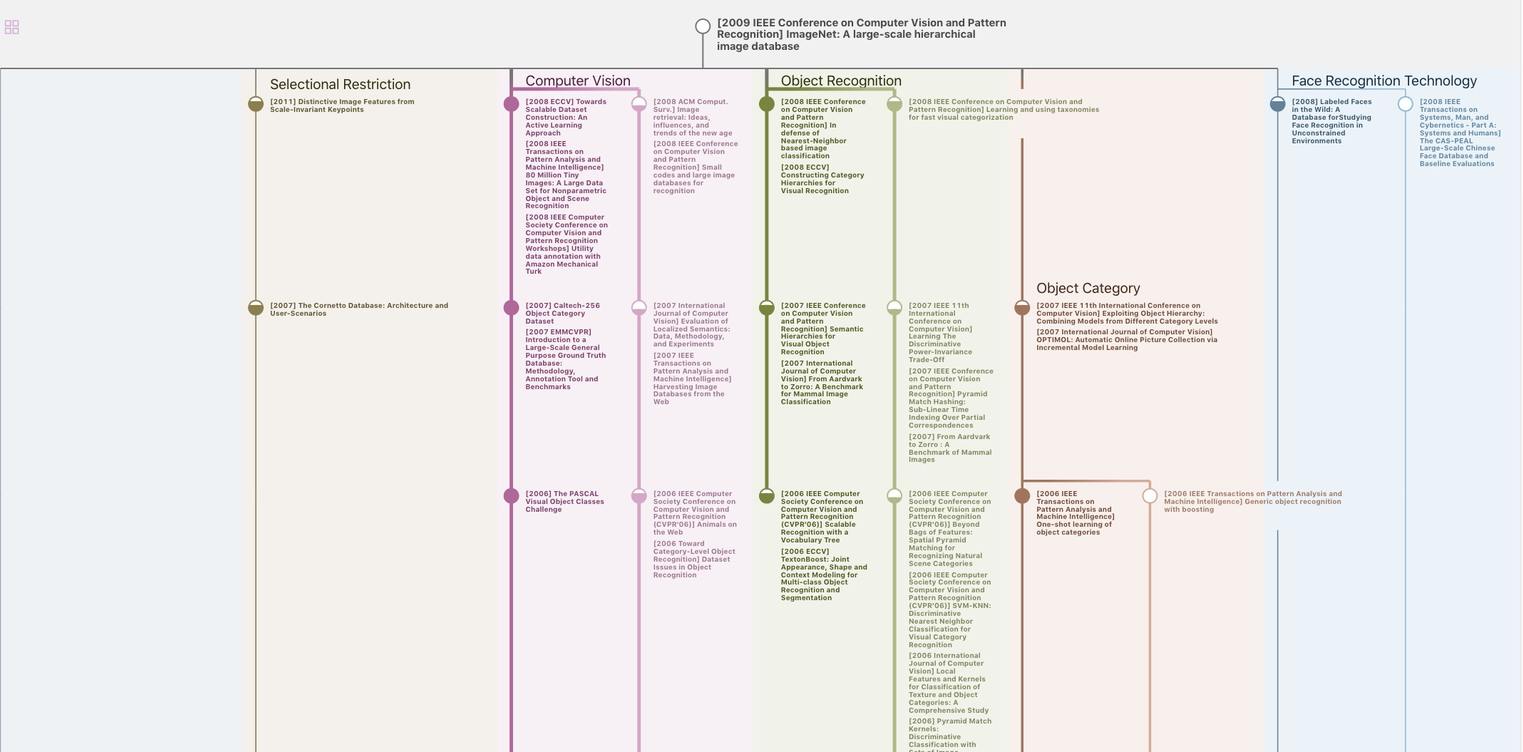
生成溯源树,研究论文发展脉络
Chat Paper
正在生成论文摘要