Model selection and inference for censored lifetime medical expenditures.
BIOMETRICS(2016)
摘要
Identifying factors associated with increased medical cost is important for many micro-and macro-institutions, including the national economy and public health, insurers and the insured. However, assembling comprehensive national databases that include both the cost and individual-level predictors can prove challenging. Alternatively, one can use data from smaller studies with the understanding that conclusions drawn from such analyses may be limited to the participant population. At the same time, smaller clinical studies have limited follow-up and lifetime medical cost may not be fully observed for all study participants. In this context, we develop new model selection methods and inference procedures for secondary analyses of clinical trial data when lifetime medical cost is subject to induced censoring. Our model selection methods extend a theory of penalized estimating function to a calibration regression estimator tailored for this data type. Next, we develop a novel inference procedure for the unpenalized regression estimator using perturbation and resampling theory. Then, we extend this resampling plan to accommodate regularized coefficient estimation of censored lifetime medical cost and develop postselection inference procedures for the final model. Our methods are motivated by data from Southwest Oncology Group Protocol 9509, a clinical trial of patients with advanced nonsmall cell lung cancer, and our models of lifetime medical cost are specific to this population. But the methods presented in this article are built on rather general techniques and could be applied to larger databases as those data become available.
更多查看译文
关键词
Induced censoring,Marked point process,Regularization,Survival analysis
AI 理解论文
溯源树
样例
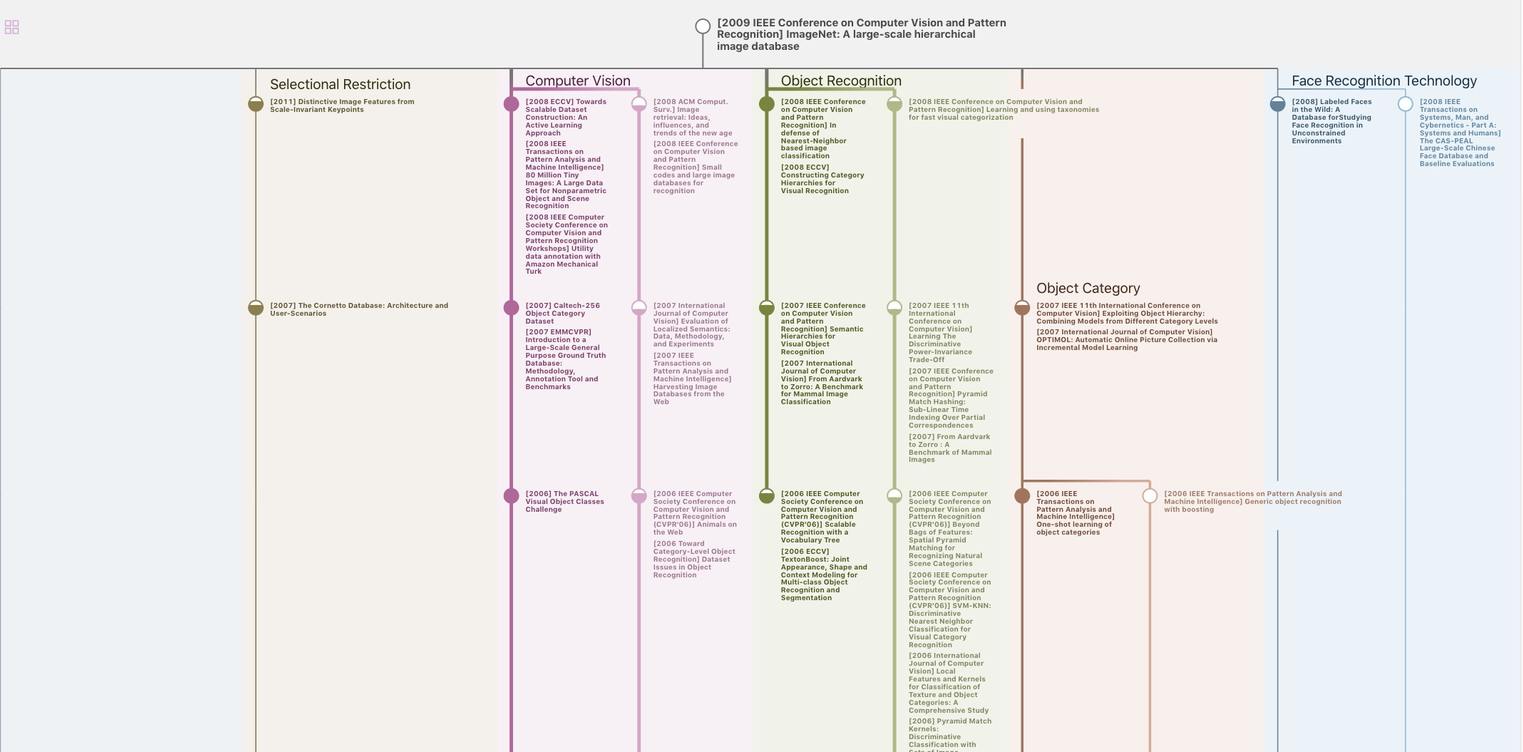
生成溯源树,研究论文发展脉络
Chat Paper
正在生成论文摘要