Learning-based Nonlinear Model Predictive Control to Improve Vision-based Mobile Robot Path Tracking
Periodicals(2016)
摘要
AbstractThis paper presents a Learning-based Nonlinear Model Predictive Control LB-NMPC algorithm to achieve high-performance path tracking in challenging off-road terrain through learning. The LB-NMPC algorithm uses a simple a priori vehicle model and a learned disturbance model. Disturbances are modeled as a Gaussian process GP as a function of system state, input, and other relevant variables. The GP is updated based on experience collected during previous trials. Localization for the controller is provided by an onboard, vision-based mapping and navigation system enabling operation in large-scale, GPS-denied environments. The paper presents experimental results including over 3ï km of travel by three significantly different robot platforms with masses ranging from 50 to 600 kg and at speeds ranging from 0.35 to 1.2 m/s associated video at http://tiny.cc/RoverLearnsDisturbances. Planned speeds are generated by a novel experience-based speed scheduler that balances overall travel time, path-tracking errors, and localization reliability. The results show that the controller can start from a generic a priori vehicle model and subsequently learn to reduce vehicle- and trajectory-specific path-tracking errors based on experience.
更多查看译文
AI 理解论文
溯源树
样例
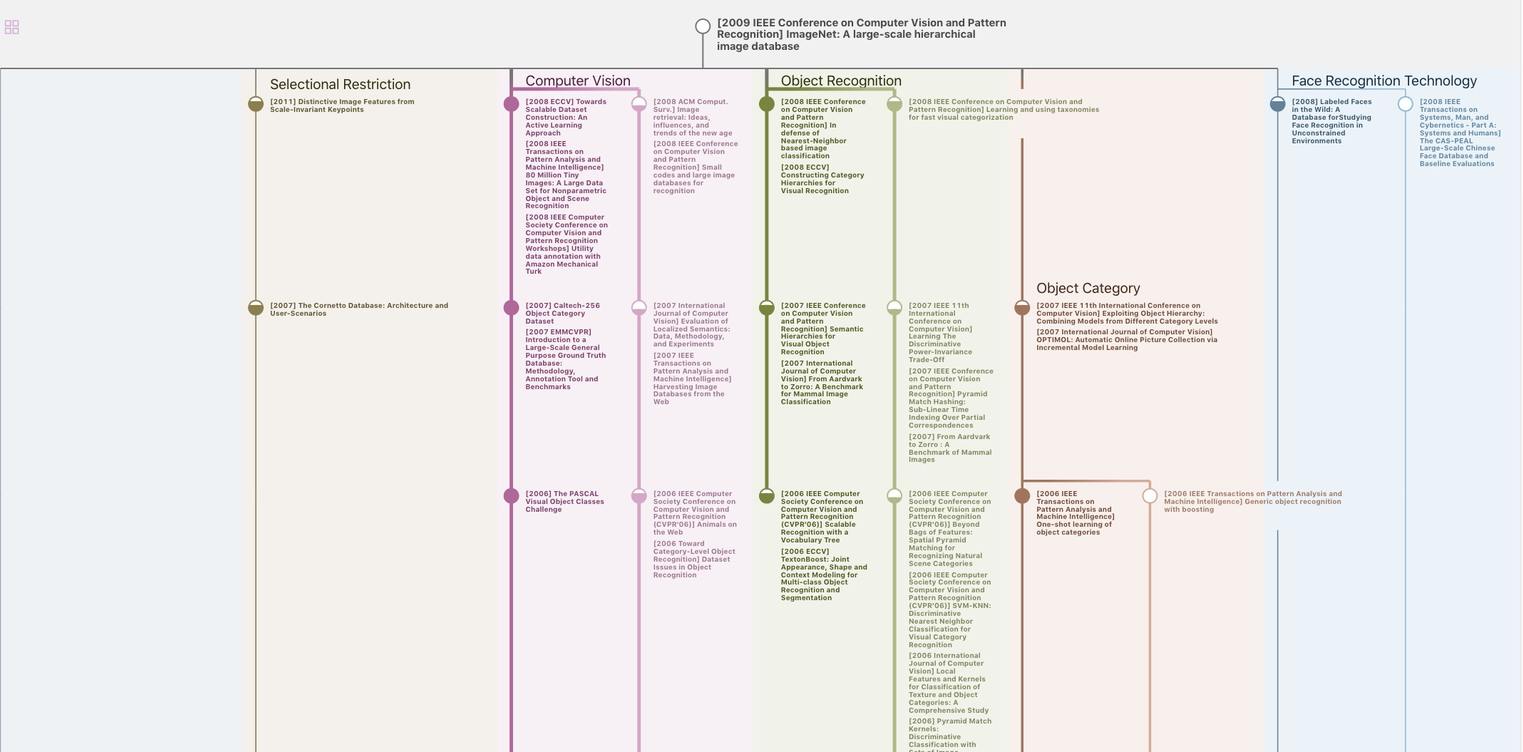
生成溯源树,研究论文发展脉络
Chat Paper
正在生成论文摘要