Named Entity Aware Transfer Learning for Biomedical Factoid Question Answering.
IEEE/ACM Transactions on Computational Biology and Bioinformatics(2022)
摘要
Biomedical factoid question answering is an important task in biomedical question answering applications. It has attracted much attention because of its reliability. In question answering systems, better representation of words is of great importance, and proper word embedding can significantly improve the performance of the system. With the success of pretrained models in general natural language processing tasks, pretrained models have been widely used in biomedical areas, and many pretrained model-based approaches have been proven effective in biomedical question-answering tasks. In addition to proper word embedding, name entities also provide important information for biomedical question answering. Inspired by the concept of transfer learning, in this study, we developed a mechanism to fine-tune BioBERT with a named entity dataset to improve the question answering performance. Furthermore, we applied BiLSTM to encode the question text to obtain sentence-level information. To better combine the question level and token level information, we use bagging to further improve the overall performance. The proposed framework was evaluated on BioASQ 6b and 7b datasets, and the results have shown that our proposed framework can outperform all baselines.
更多查看译文
关键词
Language,Learning,Machine Learning,Natural Language Processing,Reproducibility of Results
AI 理解论文
溯源树
样例
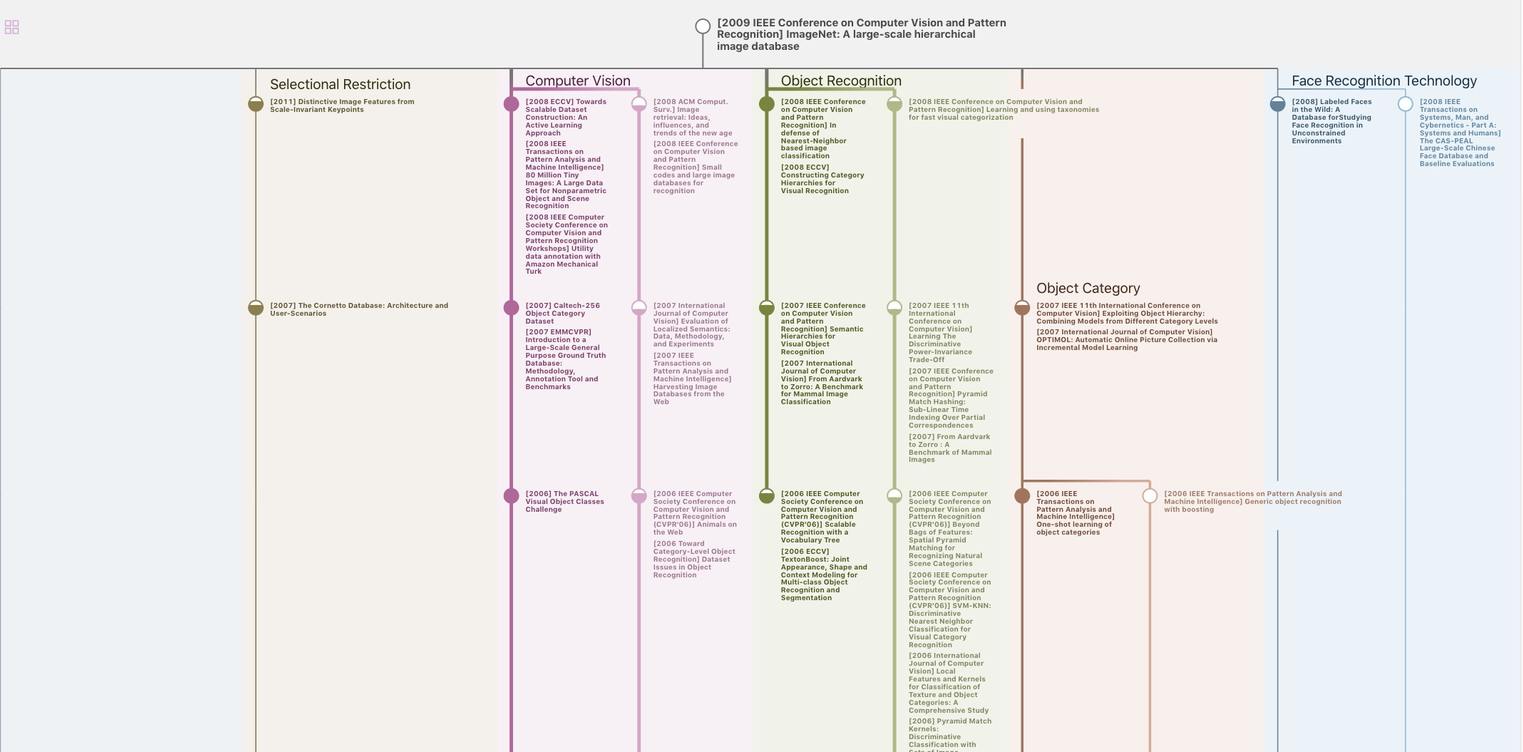
生成溯源树,研究论文发展脉络
Chat Paper
正在生成论文摘要