Deep Learning Based Load Forecasting with Decomposition and Feature Selection Techniques
JOURNAL OF SCIENTIFIC & INDUSTRIAL RESEARCH(2022)
摘要
The forecasting of short term electricity load plays a vital role in power system. It is essential for the power system's reliable, secure, and cost-effective functioning. This paper contributes significantly for enhancing the accuracy of short term electricity load forecasting. It presents a hybrid forecasting model called Gated Recurrent Unit with Ensemble Empirical Mode Decomposition and Boruta feature selection (EBGRU). It is a hybrid model that addresses the non-stationary, non-linearity and noisy issues of the time series input by using Ensemble Empirical Mode Decomposition (EEMD). It also addresses overfitting and curse of dimensionality issues of load forecasting by idaitifying the pertinent features using Bonita wrapper feature selection. It effectively handles the uncertainty and temporal dependency characteristics of load and forecasts the future load using deep learning based Gated Recurrent Unit (GRU). The proposed EBGRU model is experimented by using European and Australian Electricity load datasets. The temperature has high correlation with load demand. In this study, both load and temperature features are considered for the accurate short term load forecasting. The experimental outcome demonstrates that the proposed EBGRU model outpeiforms other deep learning models such as RNN, LSTM, GRU, RNN with EEMD and Bonita (EBRNN) and LSTM with EEMD and Bonita (EBLSTM).
更多查看译文
关键词
Boruta feature selection,Electricity load prediction,Ensemble empirical mode decomposition,Gated recurrent unit,Recurrent neural network
AI 理解论文
溯源树
样例
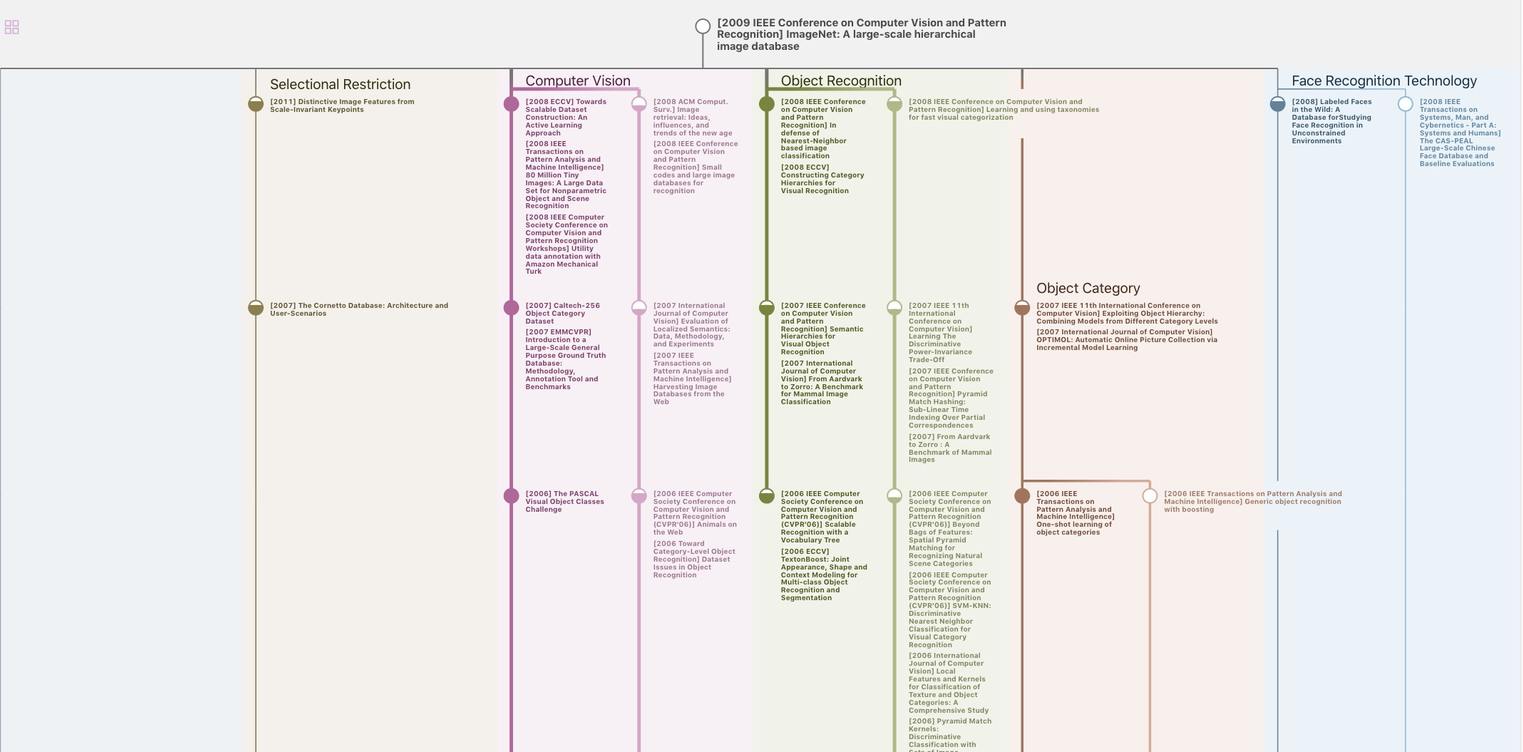
生成溯源树,研究论文发展脉络
Chat Paper
正在生成论文摘要