Generalized Polynomial Chaos-Based Extended Kalman Filter: Improvement And Expansion
PROCEEDINGS OF THE ASME INTERNATIONAL DESIGN ENGINEERING TECHNICAL CONFERENCES AND COMPUTERS AND INFORMATION IN ENGINEERING CONFERENCE, 2013, VOL 7A(2014)
摘要
The generalized polynomial chaos (gPC) method for propagating uncertain parameters through dynamical systems (previously developed at Virginia Tech) has been shown to be very computationally efficient. This method seems also to be ideal for real-time parameter estimation when merged with the Extended Kalman Filter (EKF). The resulting technique is shown in the present paper for systems in state-space representations, and then expanded to systems in regressions formulations. Due to the way the filter interacts with the polynomial chaos expansions, the covariance matrix is forced to zero in finite time. This problem shows itself as an inability to perform state estimations and causes the parameters to converge to incorrect values for state space systems. In order to address this issue, improvements to the method are implemented and the updated method is applied to both state space and regression systems. The resultant technique shows high accuracy of both state and parameter estimations.
更多查看译文
关键词
polynomials,kalman filters
AI 理解论文
溯源树
样例
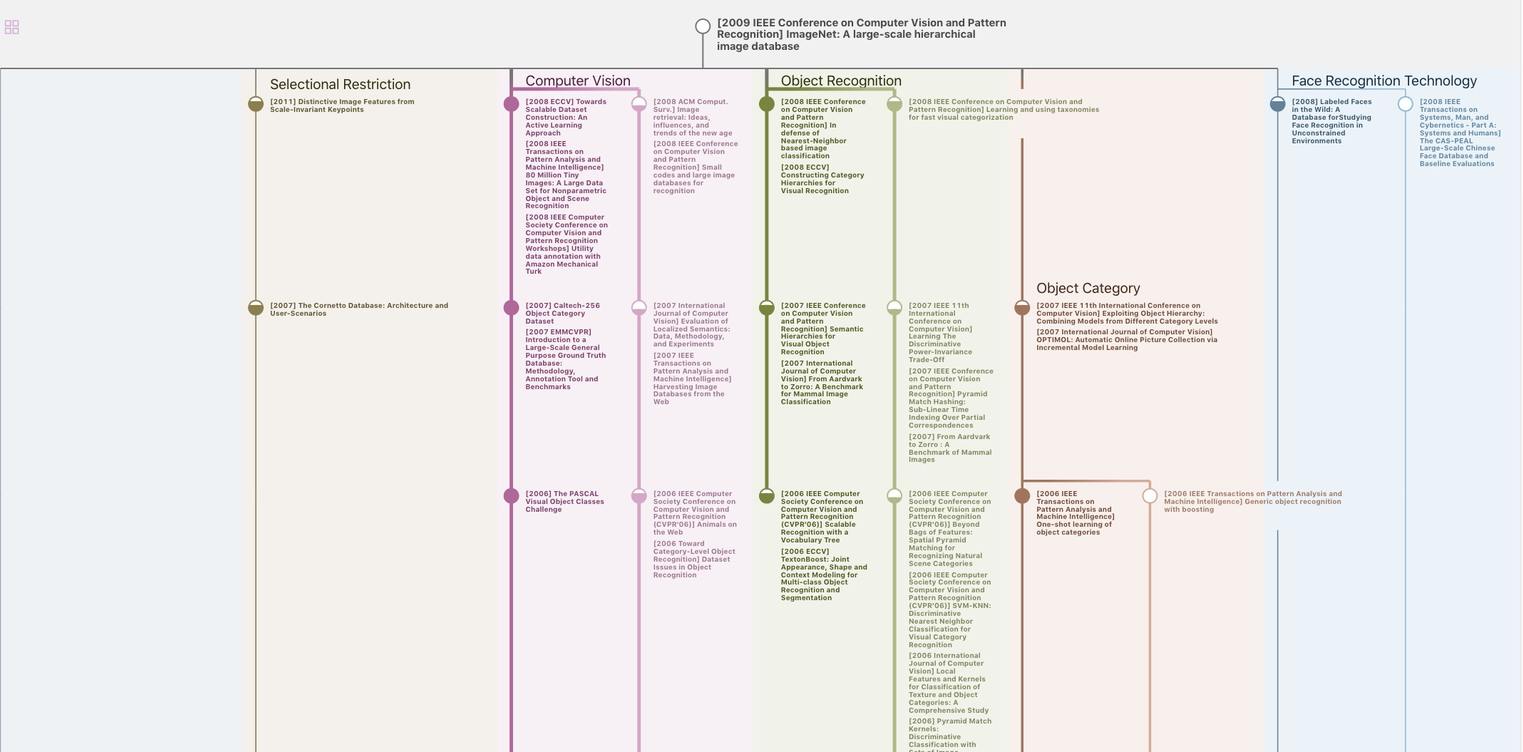
生成溯源树,研究论文发展脉络
Chat Paper
正在生成论文摘要