Comparison of Bayesian nonparametric density estimation methods
COMMUNICATIONS IN STATISTICS-THEORY AND METHODS(2020)
摘要
In this paper, we propose a nonparametric Bayesian approach for Lindsey and penalized Gaussian mixtures methods. We compare these methods with the Dirichlet process mixture model. Our approach is a Bayesian nonparametric method not based solely on a parametric family of probability distributions. Thus, the fitted models are more robust to model misspecification. Also, with the Bayesian approach, we have the entire posterior distribution of our parameter of interest; it can be summarized through credible intervals, mean, median, standard deviation, quantiles, etc. The Lindsey, penalized Gaussian mixtures, and Dirichlet process mixture methods are reviewed. The estimations are performed via Markov chain Monte Carlo (MCMC) methods. The penalized Gaussian mixtures method is implemented via Hamiltonian Monte Carlo (HMC). We show that under certain regularity conditions, and as n increases, the posterior distribution of the weights converges to a Normal distribution. Simulation results and data analysis are reported.
更多查看译文
关键词
Bayesian inference, asymptotic distribution, density estimation, Hamiltonian Monte Carlo, MCMC, penalized Gaussian mixtures
AI 理解论文
溯源树
样例
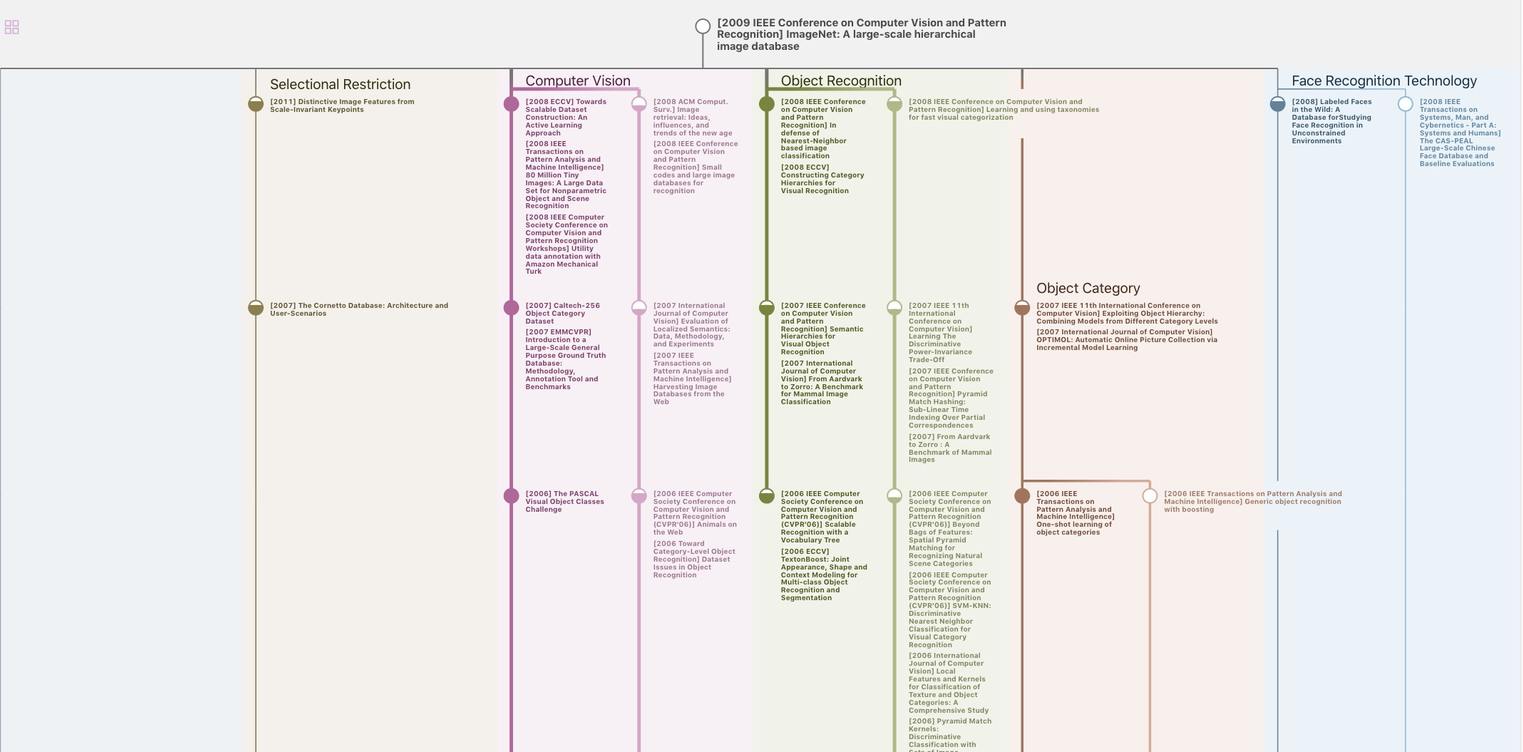
生成溯源树,研究论文发展脉络
Chat Paper
正在生成论文摘要