Bayesian Rapid Optimal Adaptive Design (BROAD): Method and application distinguishing models of risky choice
mag(2019)
摘要
Economic surveys and experiments usually present fixed questions to respondents. Rapid computation now allows adaptively optimized questions, based on previous responses, to maximize expected information. We describe a novel method of this type introduced in computer science, and apply it experimentally to six theories of risky choice. The EC2 method creates equivalence classes, each consisting of a true theory and its noisy-response perturbations, and chooses questions with the goal of distinguishing between equivalence classes by cutting edges connecting them. The edge-cutting information measure is adaptively submodular, which enables a provable performance bound and “lazy” evaluation which saves computation. The experimental data show that most subjects, making only 30 choices, can be reliably classified as choosing according to EV or two variants of prospect theory. We also show that it is difficult for subjects to manipulate by misreporting preferences, and find no evidence of manipulation.
更多查看译文
AI 理解论文
溯源树
样例
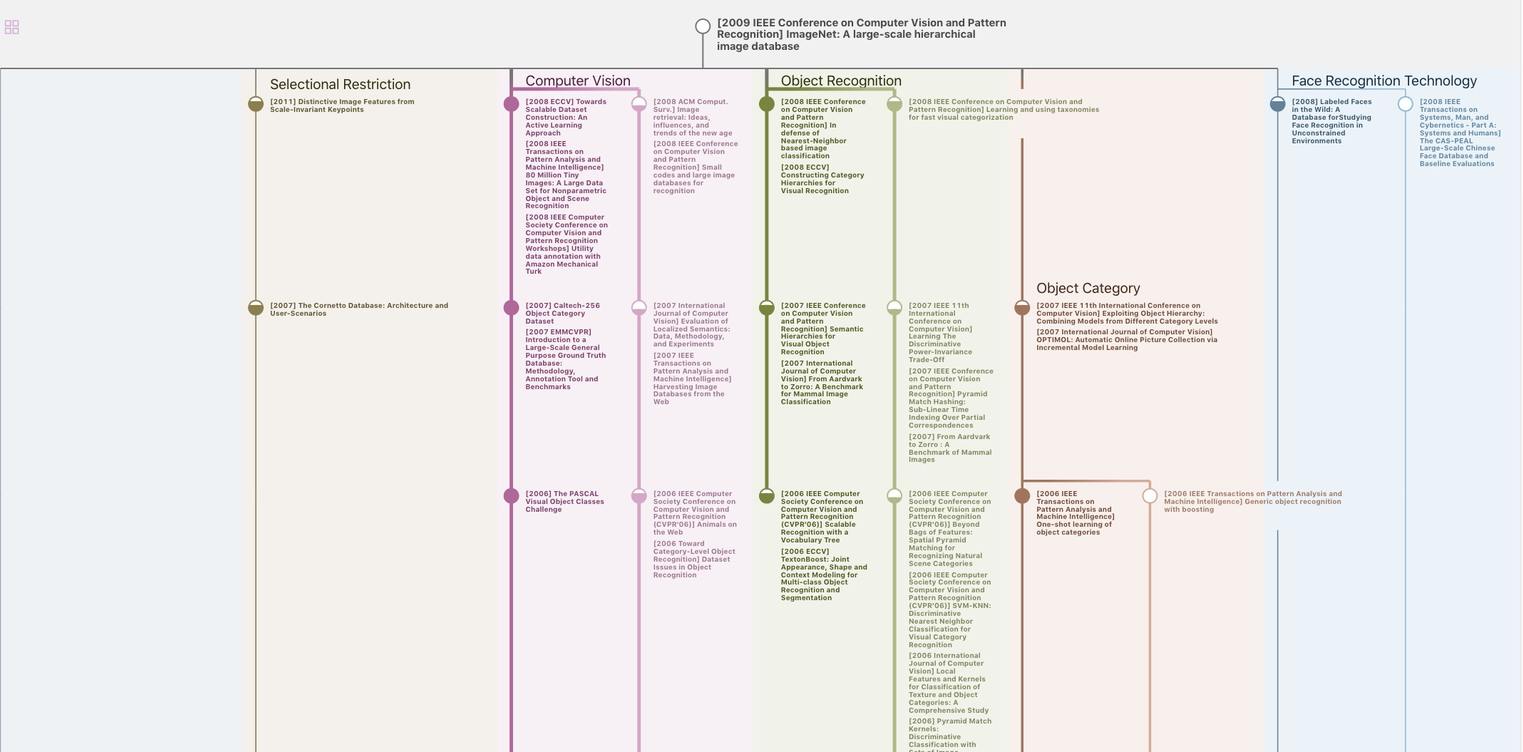
生成溯源树,研究论文发展脉络
Chat Paper
正在生成论文摘要