Benefits of textural characterization for the classification of hyperspectral images
WHISPERS(2010)
Abstract
Several spatial features are compared for the spatial/spectral classification of hyperspectral data. These features are extracted from texture spectra, co-occurrence matrices and morphological profiles. First, a PCA (Principal Components Analysis) is carried out on the hyperspectral image and textural features are computed on the first principal components. These textural features are concatenated together with spectral features (the principal components previously used) and the resulting image vector is then classified using SVM (Support Vector Machines) and a gaussian mixture algorithm. In the latter case, a hierarchical classification is used as a post-processing in order to reach a desired number of classes.
MoreTranslated text
Key words
data mining,hyperspectral imaging,feature extraction,pca,image classification,principal component,pixel,image texture,mathematical morphology,principal components analysis,support vector machines,classification,forestry,spatial resolution,hyperspectral,principal component analysis,support vector machine,svm
AI Read Science
Must-Reading Tree
Example
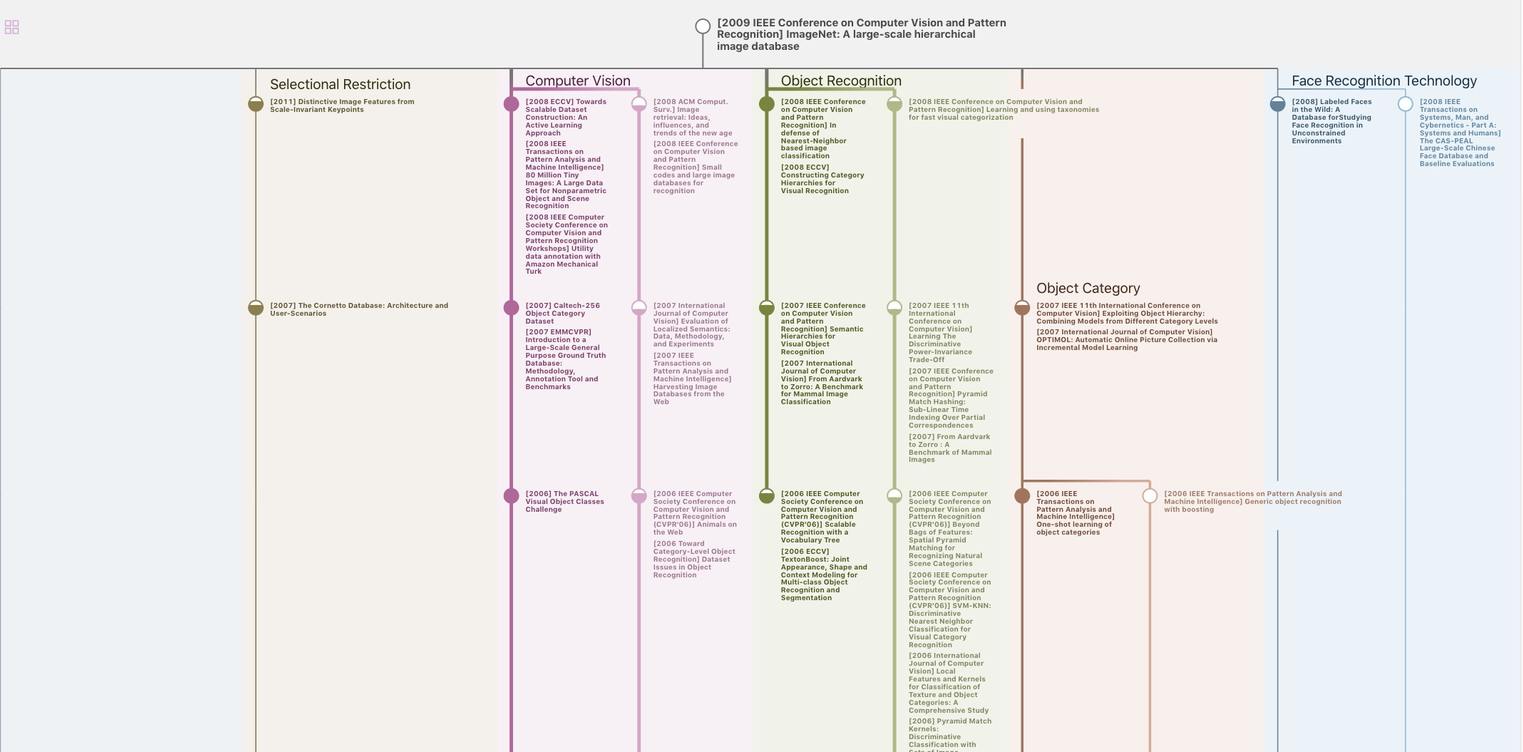
Generate MRT to find the research sequence of this paper
Chat Paper
Summary is being generated by the instructions you defined