Nonparametric Latent Tree Graphical Models: Inference, Estimation, and Structure Learning
mag(2014)
摘要
Tree structured graphical models are powerful at expressing long range or hierarchical dependency among many variables, and have been widely applied in different areas of computer science and statistics. However, existing methods for parameter estimation, inference, and structure learning mainly rely on the Gaussian or discrete assumptions, which are restrictive under many applications. In this paper, we propose new nonparametric methods based on reproducing kernel Hilbert space embeddings of distributions that can recover the latent tree structures, estimate the parameters, and perform inference for high dimensional continuous and non-Gaussian variables. The usefulness of the proposed methods are illustrated by thorough numerical results.
更多查看译文
关键词
parameter estimation
AI 理解论文
溯源树
样例
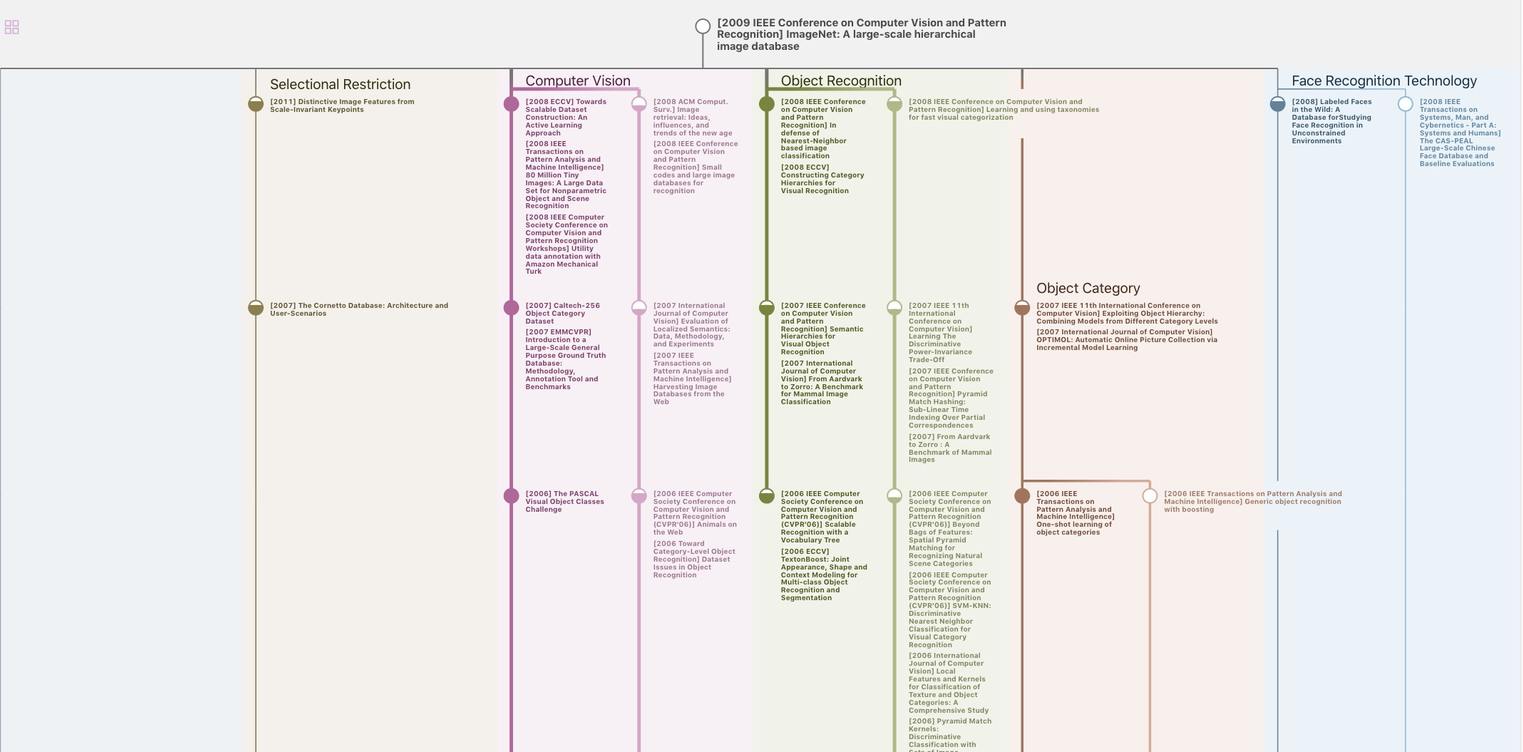
生成溯源树,研究论文发展脉络
Chat Paper
正在生成论文摘要