Hierarchical Clustering of Multiobjective Optimization Results to Inform Land-Use Decision Making
Urisa Journal(2009)
Abstract
INTRODUCTION Planning problems often have many potential solutions and multiple competing objectives. These types of problems are well addressed by multiobjective optimization methods. Multiobjective optimization is applied to problems in a variety of fields where multiple conflicting objectives must be considered. The result is a nondominated set of potential solutions. A solution is nondominated if no feasible solution exists that is better on all objectives. Balling (2004) used a multiobjective optimization algorithm to consider city and regional land-use and transportation planning. Like this paper and that of Roberts (2003), the goal of using multiobjective optimization was to improve on traditional planning methods. In most planning decisions, the alternative plans are formulated based on the experience and preferences of planners then presented to the public and the decision makers. This small set of plans cannot adequately capture the complexity of the planning problem and is inherently subjective (Balling 2004). To evaluate their approach, Balling (2004) presented the results of their analysis to local city planners, state planners, and environmental planners, as well as local politicians. All persons consulted approved of this approach and encouraged continued work although a final plan was not chosen from the 100 resulting plans. Motivating this work, Balling (2004) believes that one reason that a plan was not chosen from the optimization results is the difficulty of considering such a large number of plans. Even with a large number of plans for consideration, planners uncovered key aspects of the problem that were used in the selection of a final plan. With current computing power, it is possible to consider multiobjective problems with very large nondominated solution sets. For example, the land-use problem in this paper has 6,561 nondominated solutions. According to Balling (2004), the number of plans to be considered must be objectively reduced to a set of plans representing distinct conceptual ideas. In other words, decision makers need a sample of the nondominated solutions that is sufficiently representative of the possibilities and trade-offs but small enough for tractable consideration. The multiobjective optimization literature acknowledges the need for a method of reducing or organizing the nondominated set (Benson and Sayin 1997). Several researchers (Rosenman and Gero 1985, Morse 1980, Taboada et al. 2007) have dealt with this issue using cluster analysis or filtering. This paper differs from their work for it aims not only to make the nondominated set tractable but to do so without removing any elements of the nondominated set before presenting the solutions to the decision makers. A set of potential land-use plans is organized into nested groups of similarly performing plans without filtering out any plans. Cluster analysis can be applied to the results of a multiobjective optimization algorithm to organize or partition solutions based on their objective function values. In this paper, clustering is used to take a large set of land-use plans and organize them based on proportions of urban, natural, and agricultural land-use as well as landscape-ecology measures. The goal of clustering is to create an efficient representation that characterizes the population being sampled (Jain and Dubes 1988, p. 55). Such a representation allows a decision maker to further understand the decision by making available the attainable limits for each objective, key decisions and their consequences, and the most relevant variables; this presentation is an improvement on a list of potential solutions and their associated objective function values. This paper details a hierarchical cluster analysis approach to organize Pareto optimization results into a hierarchical representation. The methodology is applied to a greenlands system design problem in an urban fringe area in southern Ontario, Canada. …
MoreTranslated text
Key words
multiobjective optimization results,hierarchical clustering,decision making,land-use
AI Read Science
Must-Reading Tree
Example
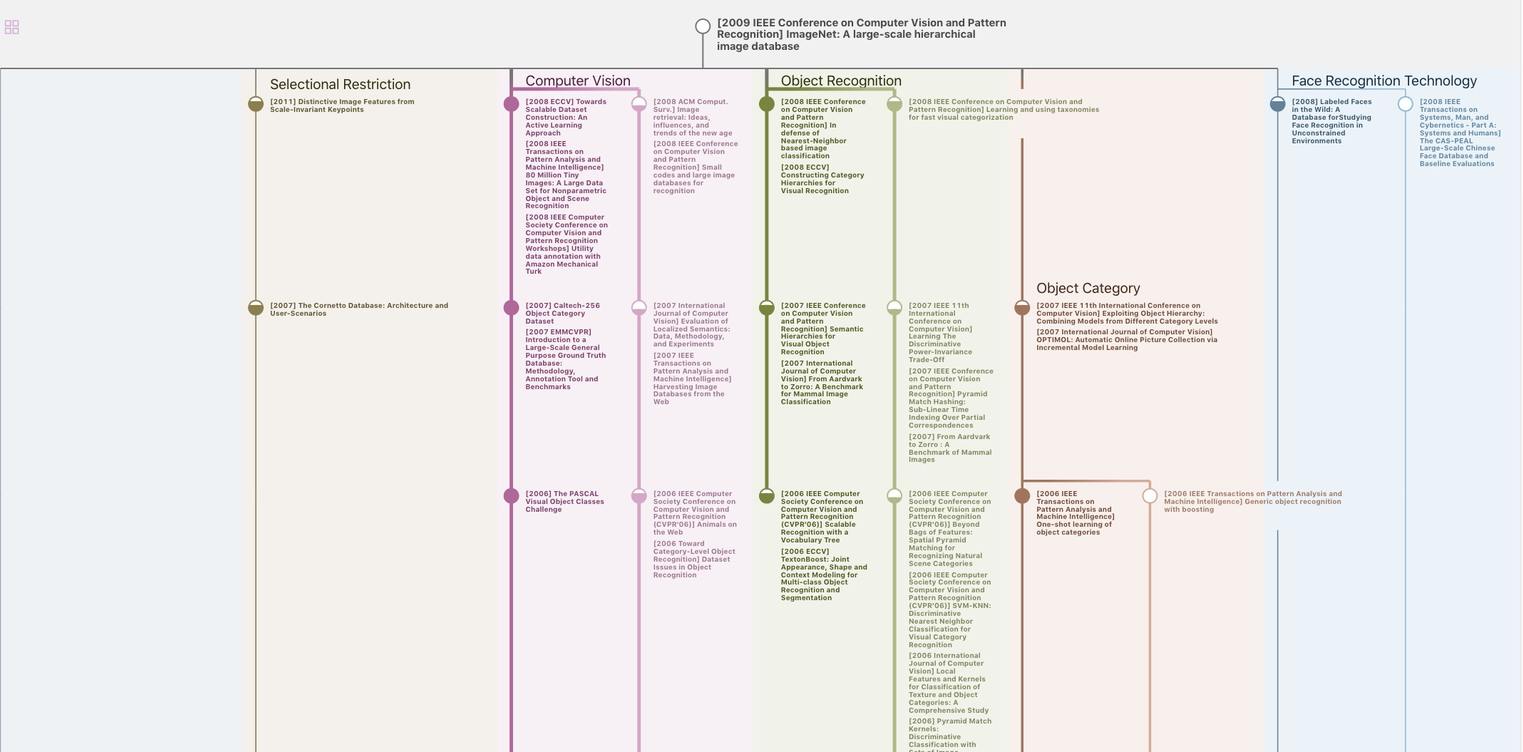
Generate MRT to find the research sequence of this paper
Chat Paper
Summary is being generated by the instructions you defined