Condition Relaxation In Conditional Statistical Shape Models
SUBSPACE METHODS FOR PATTERN RECOGNITION IN INTELLIGENT ENVIRONMENT(2014)
Abstract
A conditional statistical shape model is a valuable subspace method in medical image analysis. During training of the model, the relationship between the shape of the object of interest and a set of conditional features is established. Subsequently, while analyzing an unseen image, a measured condition is matched with this conditional distribution and then a subspace of the training data is marked as relevant and used for the desired reconstruction of the object shape. This approach can work properly only in the case when the conditional term is reliable. Unfortunately, the reliability of the conditional term is not always sufficiently high and in such situation, instead of being beneficial, the conditional term is hampering the statistical shape model. This chapter describes the advantages and disadvantages of conditional statistical shape models and discusses how relaxation of the conditional term can help to deal with possible unreliability of the conditional term. The requirements for the construction of a properly functioning relaxed conditional shape model are defined and the optimal design is tested against various alternative (relaxed) conditional shape models, showing the superiority of the optimally designed relaxed conditional shape model.
MoreTranslated text
AI Read Science
Must-Reading Tree
Example
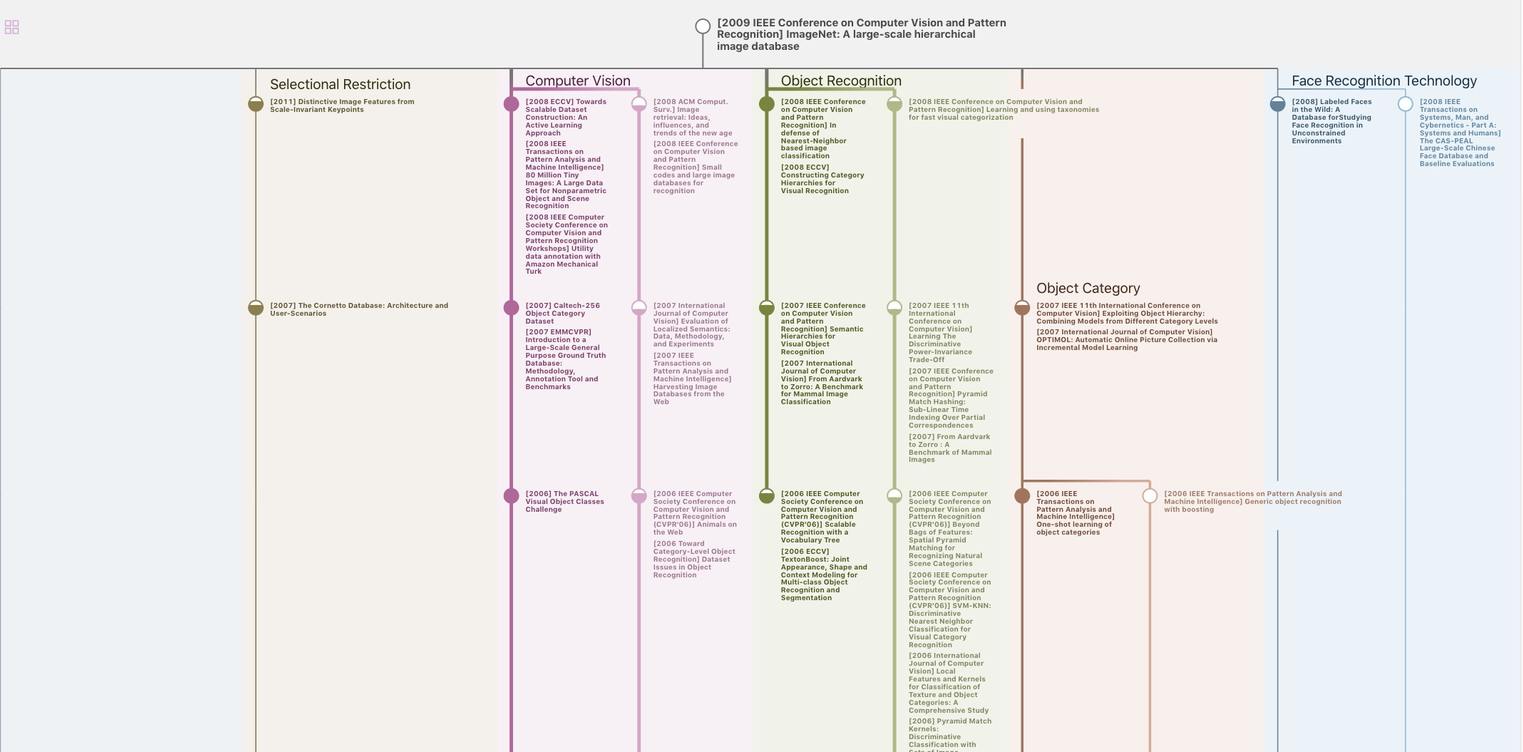
Generate MRT to find the research sequence of this paper
Chat Paper
Summary is being generated by the instructions you defined