Unsupervised building extraction using remote sensing data to detect changes in land use
Proceedings of SPIE(2014)
Abstract
Building detection plays a key role in the identification of change in urban development analysis. Algorithms currently used to detect buildings focus only on that are in use, but they cannot be used to detect buildings under construction. In this paper, we present an unsupervised classification method that detects both types of buildings. This algorithm changes the traditional idea of region growth, and combines the advantages of two spectral-based analysis techniques-mean-shift clustering and neutrosophic set theory. This case study uses images collected by unmanned aerial vehicle over different time domains. The algorithm output is more accurate than the two latest object-based classification programs: Environment for Visualizing Images Feature Extraction (ENVI-EX) and Berkeley Image Segmentation (BIS). Commission error (CE), omission error (OE) and overall accuracy (OA) are used to assess the performances of different methods. The new algorithm performs well in both building detection and change detection. In building detection, it has an overall accuracy (OA) of up to 96.4168%. In change detection, the accuracy can reach 90.6045%. Experiments show that this new algorithm works well in detecting buildings that are in use and buildings under construction, which can be used to characterize urban change.
MoreTranslated text
Key words
buildings detection,neutrosophic set,buildings under construction detection,region growing
AI Read Science
Must-Reading Tree
Example
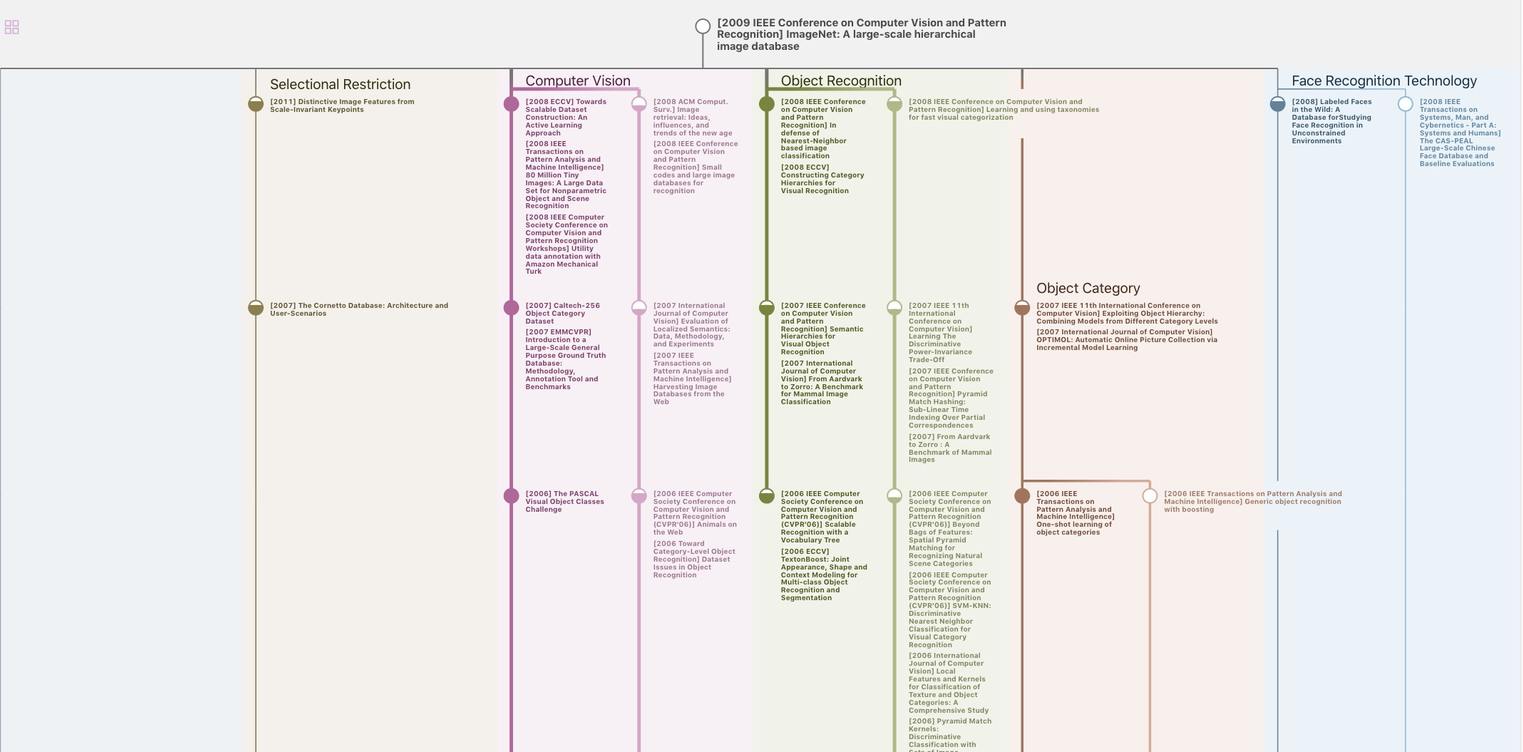
Generate MRT to find the research sequence of this paper
Chat Paper
Summary is being generated by the instructions you defined