The two-dimensional Gabor function adapted to natural image statistics: An analytical model of simple-cell responses in the early visual system
arXiv: Computer Vision and Pattern Recognition(2013)
摘要
The two-dimensional Gabor function is adapted to natural image statistics by learning the joint distribution of the Gabor function parameters. The joint distribution is then approximated to yield an analytical model of simple-cell receptive fields. Adapting a basis of Gabor functions is found to take an order of magnitude less computation than learning an equivalent non-parameterized basis. Derived learning rules are shown to be capable of adapting Gabor parameters to the statistics of images of man-made and natural environments. Learning is found to be most pronounced in three Gabor parameters that represent the size, aspect-ratio, and spatial frequency of the two-dimensional Gabor function. These three parameters are characterized by non-uniform marginal distributions with heavy tails -- most likely due to scale invariance in natural images -- and all three parameters are strongly correlated: resulting in a basis of multiscale Gabor functions with similar aspect-ratios, and size-dependent spatial frequencies. The Gabor orientation and phase parameters do not appear to gain anything from learning over natural images. Different tuning strategies are found by controlling learning through the Gabor parameter learning rates. Two opposing strategies include well-resolved orientation and well-resolved spatial frequency. On image reconstruction, a basis of Gabor functions with fitted marginal distributions is shown to significantly outperform a basis of Gabor functions generated from uniformly sampled parameters. An additional increase in performance results when the strong correlations are included. However, the best analytical model does not yet achieve the performance of the learned model. A comparison with estimates for biological simple cells shows that the Gabor function adapted to natural image statistics correctly predicts some key receptive field properties.
更多查看译文
AI 理解论文
溯源树
样例
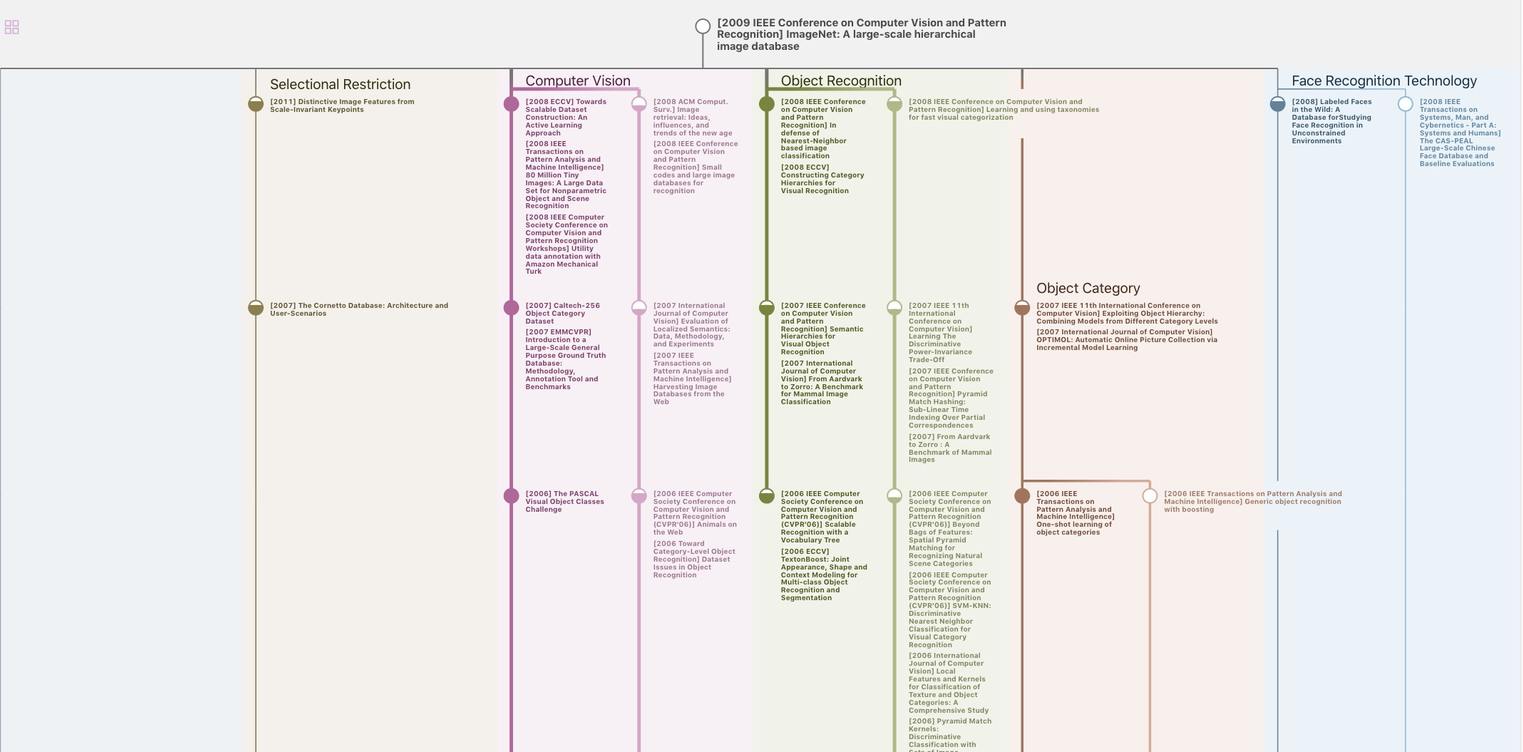
生成溯源树,研究论文发展脉络
Chat Paper
正在生成论文摘要