An Analysis Of Spectral Transformation Techniques On Graphs
COMPRESSIVE SENSING IV(2015)
摘要
Emerging methods for the spectral analysis of graphs are analyzed in this paper, as graphs are currently used to study interactions in many fields from neuroscience to social networks. There are two main approaches related to the spectral transformation of graphs. The first approach is based on the Laplacian matrix. The graph Fourier transform is defined as an expansion of a graph signal in terms of eigenfunctions of the graph Laplacian. The calculated eigenvalues carry the notion of frequency of graph signals. The second approach is based on the graph weighted adjacency matrix, as it expands the graph signal into a basis of eigenvectors of the adjacency matrix instead of the graph Laplacian. Here, the notion of frequency is then obtained from the eigenvalues of the adjacency matrix or its Jordan decomposition. In this paper, advantages and drawbacks of both approaches are examined. Potential challenges and improvements to graph spectral processing methods are considered as well as the generalization of graph processing techniques in the spectral domain. Its generalization to the time-frequency domain and other potential extensions of classical signal processing concepts to graph datasets are also considered. Lastly, it is given an overview of the compressive sensing on graphs concepts.
更多查看译文
关键词
graph theory, spectral analysis, eigenvalues and eigenvectors, graph filtering, compressed sensing
AI 理解论文
溯源树
样例
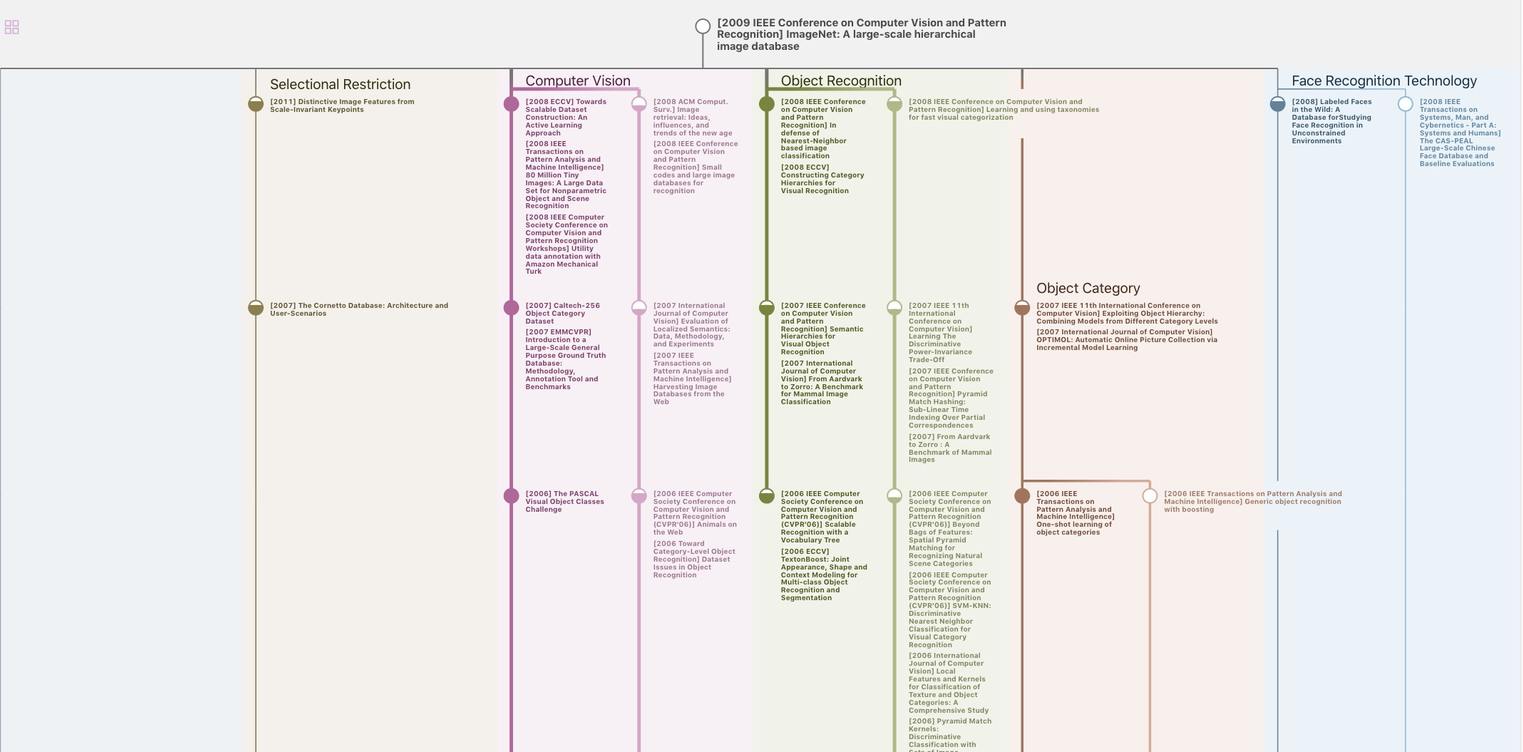
生成溯源树,研究论文发展脉络
Chat Paper
正在生成论文摘要