Petro-elastic and Lithology-fluid Inversion from Seismic Data – State-of-the-art and New Opportunities
Proceedings73rd EAGE Conference and Exhibition - Workshops 2011(2011)
Abstract
During the last couple of decades, there have been great advances in seismic inversion and lithology/fluid prediction. In the last few years, we have seen breakthroughs in integration of seismic methods, rock physics, spatial statistics and reservoir geology, allowing for more robust and realistic predictions of reservoir parameters from seismic data. The greatest advances have been made in academia and at research centers; now is the time to implement recent technologies into the workflows of the oil industry, in order to reduce exploration risk in frontier areas and boost oil recovery in existing fields. In this presentation, we give an overview of major breakthroughs in the last decade, and we suggest further extensions on how to integrate seismic inversion, rock physics, spatial statistics and geological knowledge during seismic reservoir prediction. In particular, we demonstrate that the uncertainties in lithology/fluids predictions can be reduced if geological trends are included as constraints in the inversion model. The lithology/fluid classification is constrained by depth trends and a Markov random field prior model for spatial coupling of the discrete lithology/fluids classes. A Bayesian method then combines seismic data, well observations, and prior information to predict lithology/fluid classes with associated uncertainties. The inversion approach is evaluated on a real case from the North Sea. The prior Markov random field makes it possible to identify complex structures in the lithology/fluid characteristics, by improving spatial continuity laterally. Furthermore, the posterior estimates of lithologies and fluids are used to constrain the estimation of continuous reservoir parameters like porosity. By honoring spatial continuity and vertical transitions in lithologies and fluids, we obtain sharper porosity sections and unravel details in the data not detectable from more conventional inversion algorithms. Our results also show better match with well log porosities than direct estimates of porosities from elastic parameters.
MoreTranslated text
Key words
Lithological Mapping,Seismic Data Processing,Seismic Waveform Inversion,Production Forecasting,Mineral Prospectivity
AI Read Science
Must-Reading Tree
Example
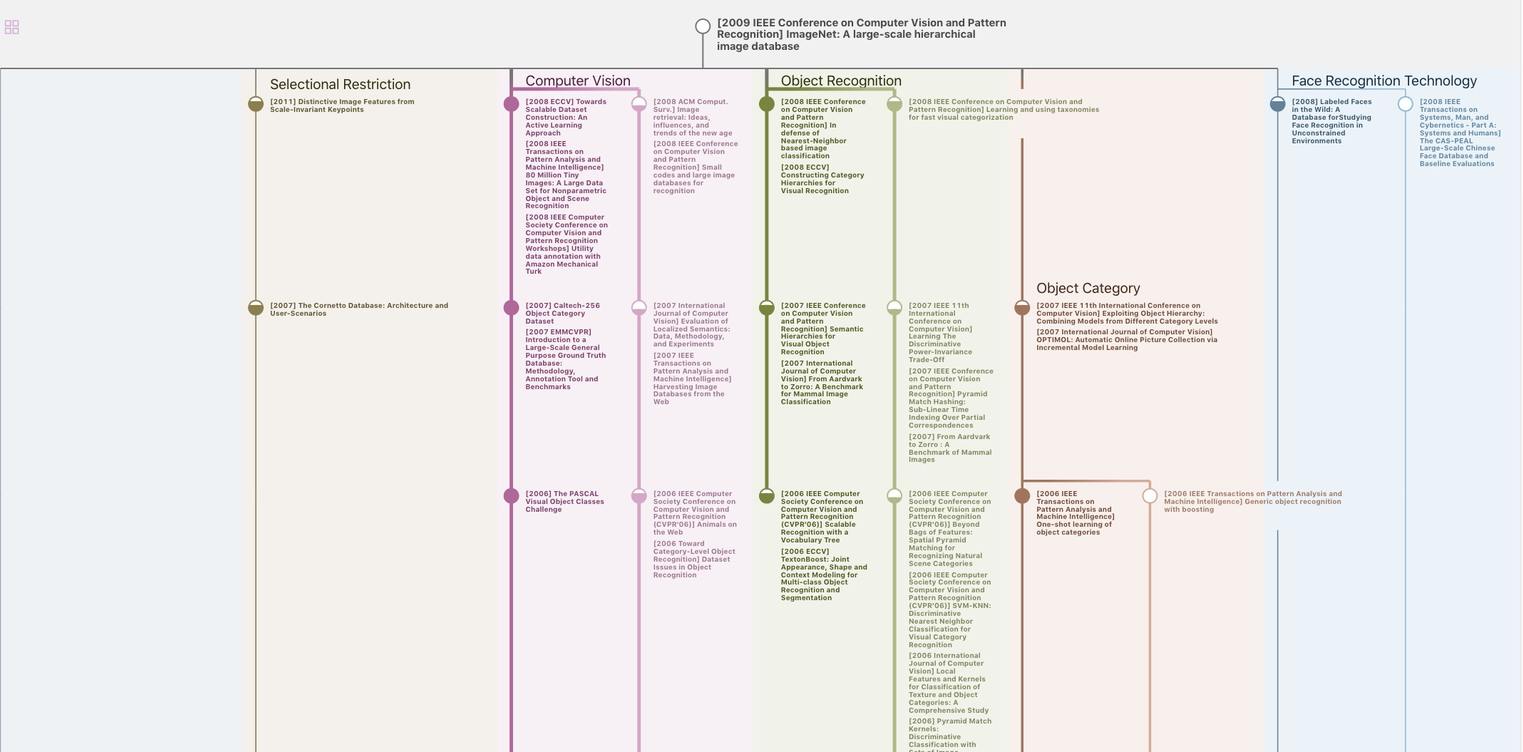
Generate MRT to find the research sequence of this paper
Chat Paper
Summary is being generated by the instructions you defined