A Real-Time Data Clustering Scheme Using K-Medoids Based Optimal Neural Network Approach for Integrating Demographics and Diagnosis Codes
IETE JOURNAL OF RESEARCH(2023)
摘要
Data clustering from the Electronic Health Record(EHR) system is an important system for diagnosing the association between the preprocessing phase and the clinical profiles of patients. According to prior studies, data heterogeneity, high costs associated with medical equipment, privacy and utility preservation, computational complexity, and other aspects make developing new clustering algorithms difficult. In this study, we introduce K-Medoids-based Optimal Neural Network (Km-ONN) to cluster the real-time (RT) dataset. Both diagnosis codes and demographic attributes of patient's values are present in an RT dataset. Based on diagnosis codes and demographic codes, the identity disclosure is prevented from attackers by applying (K, K-n) in which the information loss is minimized. The data owner specifies the utility constraint that restricts the number of generalizations. In this analysis, we used two EHR datasets, INFORMS and VERMONT, on which the proposed model performed better. With a number of 1000 medoids, the proposed method offers an accuracy, recall, Mathews correlation coefficient(MCC), and F-score of 0.99, 0.998,0.999, and 1 during training. The proposed model, on the other hand, is well suited for clustering RT datasets, resulting in more scalable and efficient outputs than other existing models.
更多查看译文
关键词
Demographic attributes, Diagnosis codes, K-medoids and Optimal Neural Network, RT dataset
AI 理解论文
溯源树
样例
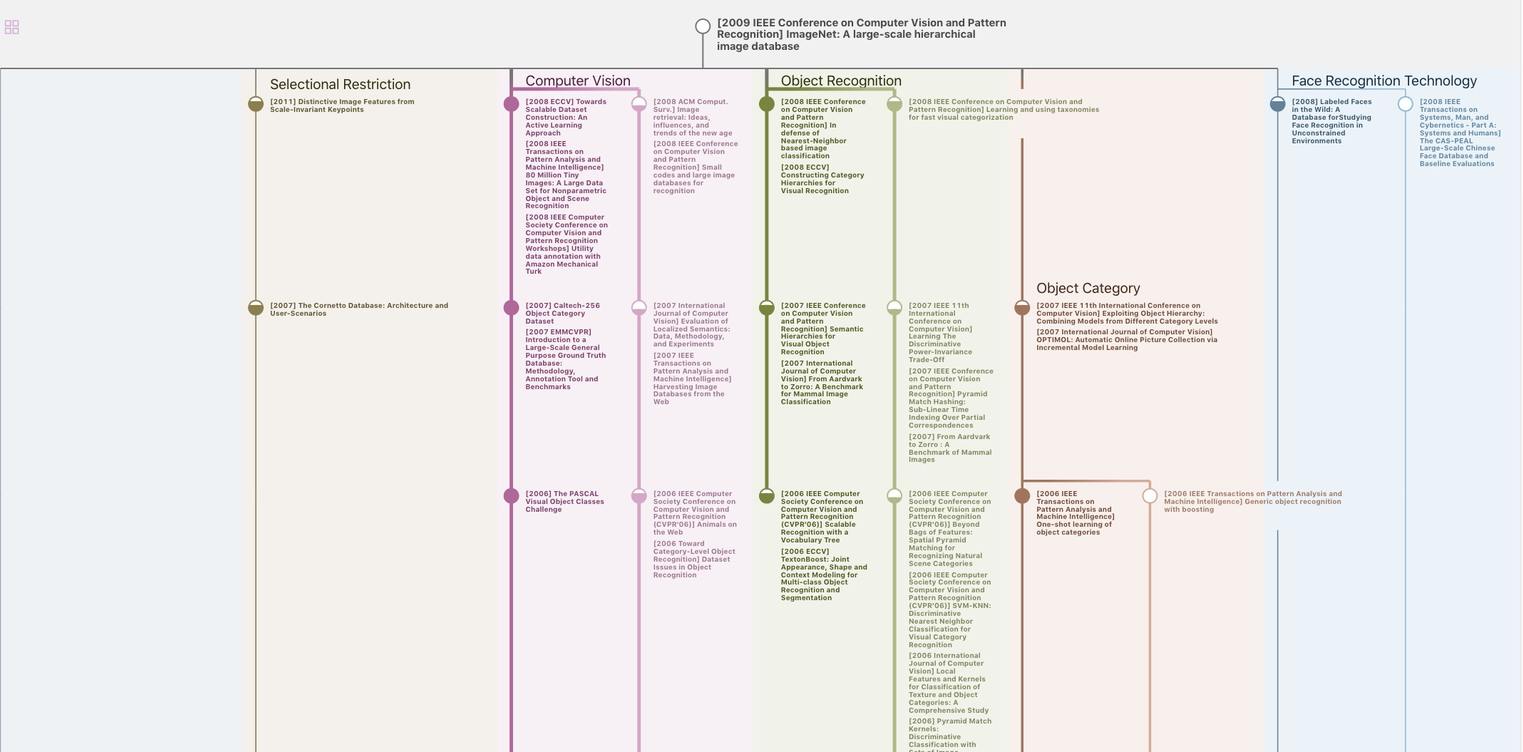
生成溯源树,研究论文发展脉络
Chat Paper
正在生成论文摘要